Low Light Image Enhancement for Video Object Detection Using Modified Zero DCE Deep Learning Model
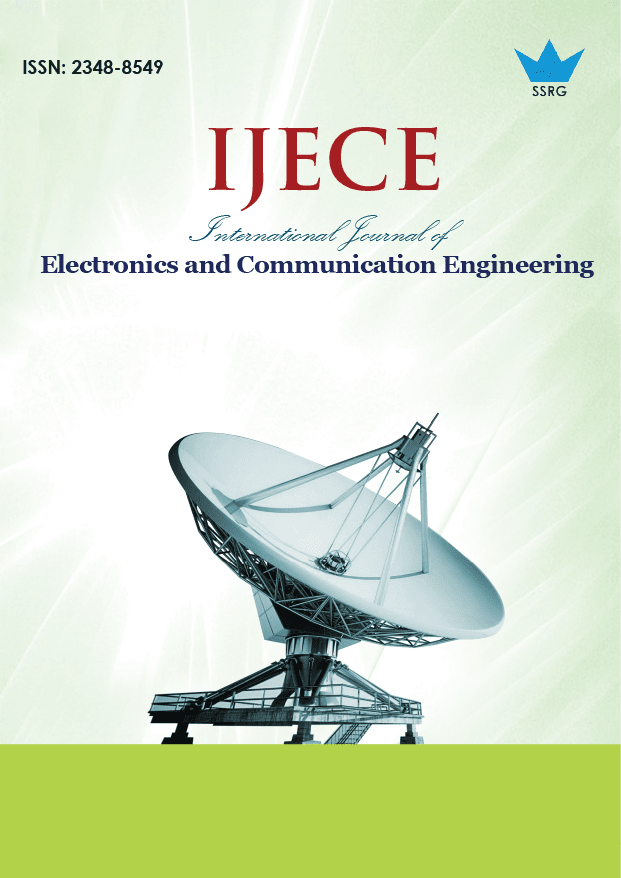
International Journal of Electronics and Communication Engineering |
© 2024 by SSRG - IJECE Journal |
Volume 11 Issue 9 |
Year of Publication : 2024 |
Authors : J. Premasagar, Sudha Pelluri |
How to Cite?
J. Premasagar, Sudha Pelluri, "Low Light Image Enhancement for Video Object Detection Using Modified Zero DCE Deep Learning Model," SSRG International Journal of Electronics and Communication Engineering, vol. 11, no. 9, pp. 290-304, 2024. Crossref, https://doi.org/10.14445/23488549/IJECE-V11I9P125
Abstract:
This paper shows how poor lighting can severely affect movies and influence the performance of video object detection systems and their practical applicability. This research problem was solved with the help of the proposed image enhancement model aimed at increasing the visibility and quality of low-illumination images. Hence, this study aims to help improve video object detection, particularly in regions of low illumination, utilizing the Enhanced Zero DCE model. This deep-learning framework does not require any reference images; therefore, it is appropriate for real-time applications. Enhanced Zero DCE eliminates DCE on high-order tonal curves, whereas the deep neural network boosts pixel values, resulting in better quality images. Using a variety of loss functions, including color constancy, exposure matching, smoothness, and spatial consistency, the model was deployed in the LoL dataset, which includes both high- and low-illumination pictures. From the experimental findings, a drastic enhancement in image quality improvement performance was evident. In terms of numbers, the effectiveness of the proposed model is outlined as follows: there is a gain of 23 percent luminance, 17 percent average illumination, 20 percent of the histogram mean, and illumination on objects when compared to traditional methods. These improvements were evident in an increase in detection accuracy by 15% and precision by 20%. Therefore, it can be said that the integration of the advanced Enhanced Zero DCE model significantly increases the efficiency of video detection of objects under dim-light conditions. These enhancements have practical applications in surveillance, automobiles, and other real-time video monitoring, especially in situations where accurate detection of objects is paramount.
Keywords:
Low-light image enhancement, Enhanced Zero DCE model, Deep learning, Reference-free image enhancement, Video object detection.
References:
[1] Mishuk Majumder, and Chester Wilmot, “Automated Vehicle Counting from Pre-Recorded Video Using You Only Look Once (YOLO) Object Detection Model,” Journal of Imaging, vol. 9, no. 7, pp. 1-19, 2023.
[CrossRef] [Google Scholar] [Publisher Link]
[2] Wenhan Yang et al., “Advancing Image Understanding in Poor Visibility Environments: A Collective Benchmark Study,” IEEE Transactions on Image Processing, vol. 29, pp. 5737-5752, 2020.
[CrossRef] [Google Scholar] [Publisher Link]
[3] P.S. Gunde, and S.K. Shirgave, “Object Identification Using Weakly Supervised Semantic Segmentation,” International Journal of Computer Engineering in Research Trends, vol. 6, no. 7, pp. 334-339, 2019.
[Google Scholar] [Publisher Link]
[4] Chongyi Li et al., “Low-Light Image and Video Enhancement Using Deep Learning: A Survey,” IEEE Transactions on Pattern Analysis and Machine Intelligence, vol. 44, no. 12, pp. 9396-9416, 2021.
[CrossRef] [Google Scholar] [Publisher Link]
[5] Mate Krišto, Marina Ivasic-Kos, and Miran Pobar, “Thermal Object Detection in Difficult Weather Conditions Using YOLO,” IEEE Access, vol. 8, pp. 125459-125476, 2020.
[CrossRef] [Google Scholar] [Publisher Link]
[6] Kyu Beom Lee, and Hyu Soung Shin, “An Application of a Deep Learning Algorithm for Automatic Detection of Unexpected Accidents Under Bad CCTV Monitoring Conditions in Tunnels,” 2019 International Conference on Deep Learning and Machine Learning in Emerging Applications (Deep-ML), Istanbul, Turkey, pp. 7-11, 2019.
[CrossRef] [Google Scholar] [Publisher Link]
[7] Wujie Zhou et al., “ECFFNet: Effective and Consistent Feature Fusion Network for RGB-T Salient Object Detection,” IEEE Transactions on Circuits and Systems for Video Technology, vol. 32, no. 3, pp. 1224-1235, 2021.
[CrossRef] [Google Scholar] [Publisher Link]
[8] M. Hassaballah et al., “Vehicle Detection and Tracking in Adverse Weather Using a Deep Learning Framework,” IEEE Transactions on Intelligent Transportation Systems, vol. 22, no. 7, pp. 4230-4242, 2020.
[CrossRef] [Google Scholar] [Publisher Link]
[9] Ahmed Alhomoud et al., “Augmenting Real-Time Surveillance with EfficientDet a Leap Towards Scalable and Accurate Object Detection,” International Journal of Computer Engineering in Research Trends, vol. 11, no. 2, pp. 9-15, 2024.
[CrossRef] [Google Scholar] [Publisher Link]
[10] Uppalapati Vamsi Krishna et al., “Enhancing Airway Assessment with a Secure Hybrid Network-Blockchain System for CT & CBCT Image Evaluation,” International Research Journal of Multidisciplinary Technovation, vol. 6, no. 2, pp. 51-69, 2024.
[CrossRef] [Google Scholar] [Publisher Link]
[11] Xin Xuet al., “Exploring Image Enhancement for Salient Object Detection in Low Light Images,” ACM Transactions on Multimedia Computing, Communications, and Applications, vol. 17, no. 1S, pp. 1-19, 2021.
[CrossRef] [Google Scholar] [Publisher Link]
[12] Guofa Li et al., “A Deep Learning Based Image Enhancement Approach for Autonomous Driving at Night,” Knowledge-Based Systems, vol. 213, 2021.
[CrossRef] [Google Scholar] [Publisher Link]
[13] Silvânio Rodrigues dos Santos, Marcos Koiti Kondo, and M. Sai Kiran, “Multimodal Fusion for Robust Banana Disease Classification and Prediction: Integrating Image Data with Sensor Networks,” Frontiers in Collaborative Research, vol. 1, no. 2, pp. 22-31, 2023.
[Google Scholar] [Publisher Link]
[14] Bo Xiao, Qiang Lin, and Yuan Chen, “A Vision-Based Method for Automatic Tracking of Construction Machines at Nighttime Based on Deep Learning Illumination Enhancement,” Automation in Construction, vol. 127, 2021.
[CrossRef] [Google Scholar] [Publisher Link]
[15] K. Venkata Ramana et al., “An Approach for Mining Top-k High Utility Item Sets (HUI),” International Journal on Recent and Innovation Trends in Computing and Communication, vol. 11, no. 2S, pp. 198-203, 2023.
[CrossRef] [Google Scholar] [Publisher Link]
[16] Sophy Ai, and Jangwoo Kwon, “Extreme Low-Light Image Enhancement for Surveillance Cameras Using Attention U-Net,” Sensors, vol. 20, no. 2, pp. 1-10, 2020.
[CrossRef] [Google Scholar] [Publisher Link]
[17] E.V.N. Jyothi et al., “A Graph Neural Network-Based Traffic Flow Prediction System with Enhanced Accuracy and Urban Efficiency,” Journal of Electrical Systems, vol. 19, no. 4, pp. 336-349, 2023.
[Google Scholar] [Publisher Link]
[18] Jiaying Liu et al., “Benchmarking Low-Light Image Enhancement and Beyond,” International Journal of Computer Vision, vol. 129, pp. 1153-1184, 2021.
[CrossRef] [Google Scholar] [Publisher Link]
[19] Weijiang Wang et al., “Low-Illumination Image Enhancement for Night-Time UAV Pedestrian Detection,” IEEE Transactions on Industrial Informatics, vol. 17, no. 8, pp. 5208-5217, 2020.
[CrossRef] [Google Scholar] [Publisher Link]
[20] M. Bhavsingh, K. Venkatesh Sharma, and Mustafa Abdulkareem Salman Al-Nuaimi, “Integrating GAN-Based Image Enhancement with YOLOv5 Object Detection for Accurate Vehicle Number Plate Analysis,” International Journal of Computer Engineering in Research Trends, vol. 10, no. 6, pp. 9-14, 2023.
[CrossRef] [Publisher Link]
[21] Christian Brynning, A. Schirrer, and S. Jakubek, “Transfer Learning for Agile Pedestrian Dynamics Analysis: Enabling Real-Time Safety at Zebra Crossings,” Synthesis: A Multidisciplinary Research Journal, vol. 1, no. 1, pp. 22-31, 2023.
[Google Scholar] [Publisher Link]
[22] Long Ma et al., “Learning Deep Context-Sensitive Decomposition for Low-Light Image Enhancement,” IEEE Transactions on Neural Networks and Learning Systems, vol. 33, no. 10, pp. 5666-5680, 2021.
[CrossRef] [Google Scholar] [Publisher Link]
[23] Chunle Guo et al., “Zero-Reference Deep Curve Estimation for Low-Light Image Enhancement,” Proceedings of the IEEE/CVF Conference on Computer Vision and Pattern Recognition, pp. 1780-1789, 2020.
[Google Scholar] [Publisher Link]
[24] Zunjin Zhao et al., “RetinexDIP: A Unified Deep Framework for Low-Light Image Enhancement,” IEEE Transactions on Circuits and Systems for Video Technology, vol. 32, no. 3, pp. 1076-1088, 2021.
[CrossRef] [Google Scholar] [Publisher Link]
[25] Wencheng Wang et al., “An Experiment-Based Review of Low-Light Image Enhancement Methods,” IEEE Access, vol. 8, pp. 87884- 87917, 2020.
[CrossRef] [Google Scholar] [Publisher Link]
[26] P. SumanPrakash et al., “Learning-driven Continuous Diagnostics and Mitigation Program for Secure Edge Management through Zero-Trust Architecture,” Computer Communications, vol. 220, pp. 94-107, 2024.
[CrossRef] [Google Scholar] [Publisher Link]
[27] Li-Wen Wang et al., “Lightening Network for Low-Light Image Enhancement,” IEEE Transactions on Image Processing, vol. 29, pp. 7984-7996, 2020.
[CrossRef] [Google Scholar] [Publisher Link]
[28] Atik Garg, Xin-Wen Pan, and Lan-Rong Dung, “LiCENt: Low-Light Image Enhancement Using the Light Channel of HSL,” IEEE Access, vol. 10, pp. 33547-33560, 2022.
[CrossRef] [Google Scholar] [Publisher Link]