Advanced Hybrid Method for Precise Identification and Categorization of Brain Stroke from CT Images
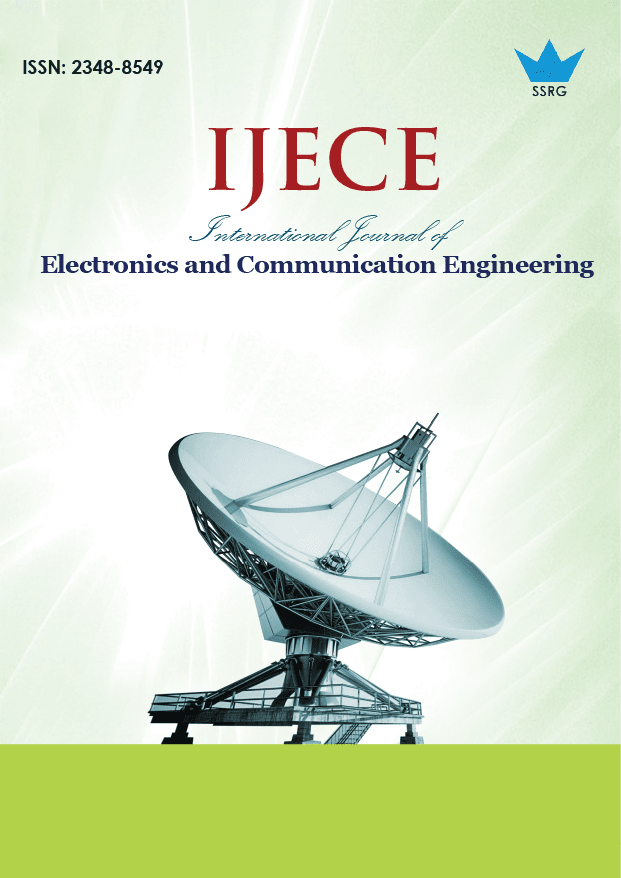
International Journal of Electronics and Communication Engineering |
© 2024 by SSRG - IJECE Journal |
Volume 11 Issue 10 |
Year of Publication : 2024 |
Authors : Shahina A.R, I. Sowmy |
How to Cite?
Shahina A.R, I. Sowmy, "Advanced Hybrid Method for Precise Identification and Categorization of Brain Stroke from CT Images," SSRG International Journal of Electronics and Communication Engineering, vol. 11, no. 10, pp. 135-148, 2024. Crossref, https://doi.org/10.14445/23488549/IJECE-V11I10P111
Abstract:
Brain stroke is a serious medical condition that occurs when the brain’s blood supply is disrupted, either due to a blockage or the rupture of a blood vessel. This interruption results in a sudden loss of brain function, manifesting as symptoms like difficulty speaking, weakness or paralysis of the limbs, confusion, or altered consciousness. The severity of a stroke is influenced by both the duration of the blood flow disruption and the specific location of the damage within the brain. Immediate medical intervention is crucial for reducing damage and improving the chances of recovery. Key risk factors for stroke include hypertension, diabetes, obesity, a sedentary lifestyle, and smoking. Given the critical need for timely and accurate stroke diagnosis, this study introduces a novel Deep Learning (DL) model for detecting and classifying brain strokes using brain CT images. The proposed method combines DenseNet 201 and Capsule Network (CapsNet) models to enhance classification accuracy. Experimental results demonstrate that the model achieved an accuracy of 93.45%, a precision of 92.18%, a recall of 92.56%, and an F1 score of 92.36%, underscoring its effectiveness in diagnosing and classifying strokes with high accuracy.
Keywords:
Brain Stroke, DenseNet 201, Capsule network, CT images, Medical imaging, Deep learning.
References:
[1] Lavdim Kurtaj, Vjosa Shatri, and Ilir Limani, “Cerebellar Model Controller with New Model of Granule Cell-Golgi Cell Building Blocks and Two-Phase Learning Acquires Multitude of Generalization Capabilities in Controlling Robot Joint Without Exponential Growth in Complexity,” International Journal of Electrical and Computer Engineering, vol. 8, no. 6, pp. 4292-4309, 2018.
[CrossRef] [Google Scholar] [Publisher Link]
[2] Michael S. Phipps, and Carolyn A. Cronin, “Management of Acute Ischemic Stroke,” BMJ, vol. 368, 2020.
[CrossRef] [Google Scholar] [Publisher Link]
[3] Saleh Salehi Zahabi et al., “Evaluation of Causes of Brain CT Scan in Patients with Minor Trauma,” International Journal of Surgery Open, vol. 27, pp. 220-224, 2020.
[CrossRef] [Google Scholar] [Publisher Link]
[4] Azhar Tursynova et al., “Deep Learning-Enabled Brain Stroke Classification on Computed Tomography Images,” Computers, Materials & Continua, vol. 75, no. 1, pp. 1431-1446, 2023.
[CrossRef] [Google Scholar] [Publisher Link]
[5] Dhonita Tripura et al., “A BrainNet (BrN) Based New Approach to Classify Brain Stroke from CT Scan Images,” 2023 International Conference on Advancement in Computation & Computer Technologies (InCACCT), Gharuan, India, pp. 1-6, 2023.
[CrossRef] [Google Scholar] [Publisher Link]
[6] Kun-Yu Lee et al., “Automatic Detection and Vascular Territory Classification of Hyperacute Staged Ischemic Stroke on Diffusion Weighted Image Using Convolutional Neural Networks,” Scientific Reports, vol. 13, pp. 1-11, 2023.
[CrossRef] [Google Scholar] [Publisher Link]
[7] Syu-Jyun Peng et al., “Automated Cerebral Infarct Detection on Computed Tomography Images Based On Deep Learning,” Biomedicines, vol. 10, no. 1, pp. 1-14, 2022.
[CrossRef] [Google Scholar] [Publisher Link]
[8] Lifeng Zhang et al., “An Improved Detection Algorithm for Ischemic Stroke NCCT Based on YOLOv5,” Diagnostics, vol. 12, no. 11, pp. 1-10, 2022.
[CrossRef] [Google Scholar] [Publisher Link]
[9] Batyrkhan Omarov et al., “Modified UNet Model for Brain Stroke Lesion Segmentation on Computed Tomography Images,” Computers, Materials & Continua, vol. 71, no. 3, pp. 1-17, 2022.
[CrossRef] [Google Scholar] [Publisher Link]
[10] Anjali Gautam, and Balasubramanian Raman, “Towards Effective Classification of Brain Hemorrhagic and Ischemic Stroke Using CNN,” Biomedical Signal Processing and Control, vol. 63, 2021.
[CrossRef] [Google Scholar] [Publisher Link]
[11] Guoqing Wu et al., “Identification of Invisible Ischemic Stroke in Noncontrast CT Based on Novel Two‐Stage Convolutional Neural Network Model,” Medical Physics, vol. 48, no. 3, pp. 1262-1275, 2021.
[CrossRef] [Google Scholar] [Publisher Link]
[12] Chung-Ming Lo, Peng-Hsiang Hung, and Daw-Tung Lin, “Rapid Assessment of Acute Ischemic Stroke by Computed Tomography Using Deep Convolutional Neural Networks,” Journal of Digital Imaging, vol. 34, no. 3, pp. 637-646, 2021.
[CrossRef] [Google Scholar] [Publisher Link]
[13] R. Aishvarya et al., “Early Detection of Brain Stroke using MRI Images,” International Research Journal of Engineering and Technology, vol. 7, no. 9, pp. 2258-2261, 2020.
[Google Scholar] [Publisher Link]
[14] B.S. Maya, and T. Asha, “Predictive Model for Brain Stroke in CT Using Deep Neural Network,” International Journal of Recent Technology and Engineering, vol. 9, no. 1, pp. 2011-2017, 2020.
[CrossRef] [Google Scholar] [Publisher Link]
[15] Asit Subudhi, Manasa Dash, and Sukanta Sabut, “Automated Segmentation and Classification of Brain Stroke Using Expectation Maximization and Random Forest Classifier,” Biocybernetics and Biomedical Engineering, vol. 40, no. 1, pp. 277-289, 2020.
[CrossRef] [Google Scholar] [Publisher Link]
[16] R. Kanchana, and R. Menaka, “Ischemic Stroke Lesion Detection, Characterization and Classification in CT Images with Optimal Features Selection,” Biomedical Engineering Letters, vol. 10, pp. 333-344, 2020.
[CrossRef] [Google Scholar] [Publisher Link]
[17] Anjali Gautam, and Balasubramanian Raman, “Local Gradient of Gradient Pattern: A Robust Image Descriptor for the Classification of Brain Strokes from Computed Tomography Images,” Pattern Analysis and Applications, vol. 23, pp. 797-817, 2020.
[CrossRef] [Google Scholar] [Publisher Link]
[18] Mandeep Singh, Varinder Garg, and Parmod Bhat, “Early Detection of Stroke Using Texture Analysis,” Indian Journal of Forensic Medicine & Toxicology, vol. 13, no. 3, pp. 49-52, 2019.
[CrossRef] [Google Scholar] [Publisher Link]
[19] Brain Stroke Prediction CT Scan Image Dataset, Kaggle. [Online]. Available: https://www.kaggle.com/datasets/alymaher/brain-stroke-ct scan-image/data
[20] Poonguzhali Elangovan, “Detection of Glaucoma from Fundus Image Using Pre-Trained Densenet201 Model,” Indian Journal of Radio & Space Physics, vol. 50, no. 1, pp. 1-7, 2021.
[Google Scholar] [Publisher Link]
[21] Tianming Zhao et al., “A Deep Learning Iris Recognition Method Based on Capsule Network Architecture,” IEEE Access, vol. 7, pp. 49691-49701, 2019.
[CrossRef] [Google Scholar] [Publisher Link]