EEG Signal-Based Emotion Detection of Parkinson’s Patients for Classification and Feature Extraction by Deep Learning Architecture
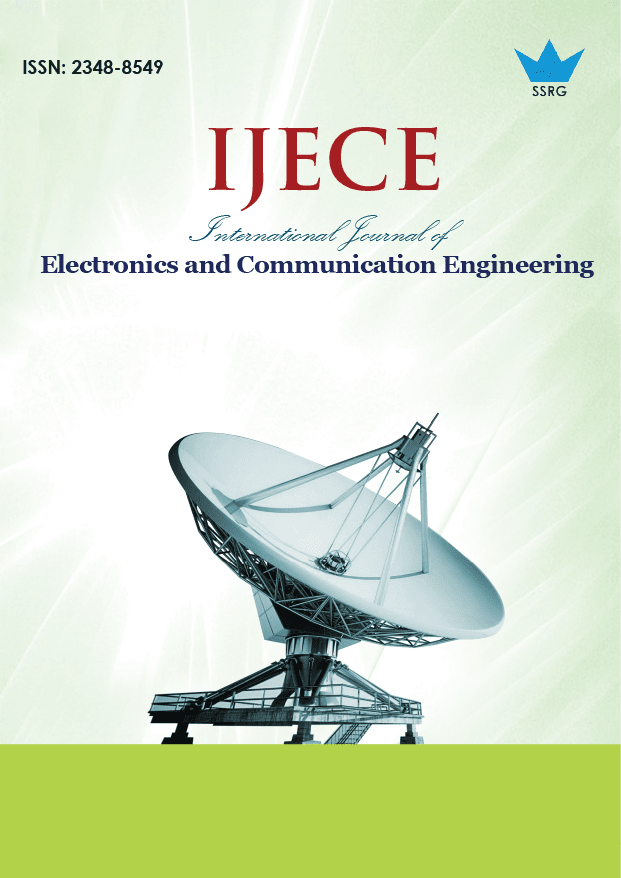
International Journal of Electronics and Communication Engineering |
© 2024 by SSRG - IJECE Journal |
Volume 11 Issue 10 |
Year of Publication : 2024 |
Authors : Shailaja Kotte, J R K Kumar Dabbakuti |
How to Cite?
Shailaja Kotte, J R K Kumar Dabbakuti, "EEG Signal-Based Emotion Detection of Parkinson’s Patients for Classification and Feature Extraction by Deep Learning Architecture," SSRG International Journal of Electronics and Communication Engineering, vol. 11, no. 10, pp. 266-276, 2024. Crossref, https://doi.org/10.14445/23488549/IJECE-V11I10P122
Abstract:
EEG-based emotion classification reflects both external and internal emotional states and has applications in the interactive brain-computer interface, patient psychological health monitoring, and entertainment consumption behaviour. This leads to more accurate, organic, and meaningful human-computer interaction—variations in experimental settings and cognitive health factors present difficulties for EEG-based emotion recognition in practical applications. The second most prevalent neurodegenerative condition, Parkinson’s Disease (PD), impairs the ability to recognize and express emotions. This research proposes a novel method in EEG signal-based emotion detection of Parkinson’s patients by classification and feature extraction utilizing DL(Deep Learning) methods. EEG brain waves from Parkinson’s patients are used as the input, cleaned up and normalized to produce EEG fragments. Quantum convolutional learning has been used to extract features from the processed input EEG signal. Then, the extracted features are classified utilizing spatial encoder back propagation neural networks, and the classified output shows the detected emotions of Parkinson’s patients. The experimental analysis is carried out for different Parkinson patient’s EEG brain wave datasets regarding accuracy, precision, recall, F-1 score, SNR, RMSE and MAP.
Keywords:
Electroencephalogram, Emotion recognition, Parkinson patients, Feature extraction, Classification.
References:
[1] Ashok Vajravelu et al., “Survey and Analysis of Preprocessing OfEEG Signal,” Annals of the Romanian Society for Cell Biology, vol. 25, no. 6, pp. 2461-2488, 2021.
[Google Scholar] [Publisher link]
[2] Pavlos Christodoulides et al., “Classification of EEG Signals From Young Adults with Dyslexia Combining a Brain Computer Interface Device and an Interactive Linguistic Software Tool,” Biomedical Signal Processing and Control, vol. 76, 2022.
[CrossRef] [Google Scholar] [Publisher link]
[3] Yao Zhang, and Qiang Ni, “Design of Quantum Neuron Model for Quantum Neural Networks,” Quantum Engineering, vol. 3, no. 3, 2021.
[CrossRef] [Google Scholar] [Publisher link]
[4] Stefanie Winkelmann et al., “Mathematical Modeling of Spatio-Temporal Population Dynamics and Application to Epidemic Spreading,” Mathematical Biosciences, vol. 336, pp. 1-17, 2021.
[CrossRef] [Google Scholar] [Publisher link]
[5] Hao Pan, “An Improved Back-Propagation Neural Network Algorithm,” Applied Mechanics and Materials, vol. 556, pp. 4586-4590, 2014.
[CrossRef] [Google Scholar] [Publisher link]
[6] Md. Asadur Rahman et al., “Emotion Recognition from EEG-Based Relative Power Spectral Topography Using Convolutional Neural Network,” Array, vol. 11, pp. 1-11, 2021.
[CrossRef] [Google Scholar] [Publisher link]
[7] Muhammad Najam Dar et al., “EEG-Based Emotion Charting for Parkinson's Disease Patients Using Convolutional Recurrent Neural Networks and Cross Dataset Learning,” Computers in Biology and Medicine, vol. 144, 2022.
[CrossRef] [Google Scholar] [Publisher link]
[8] Turker Tuncer, Sengul Dogan, and Abdulhamit Subasi, “A New Fractal Pattern Feature Generation Function Based Emotion Recognition Method Using EEG,” Chaos, Solitons & Fractals, vol. 144, 2021.
[CrossRef] [Google Scholar] [Publisher link]
[9] Smith K. Khare, Varun Bajaj, and U. Rajendra Acharya, “PDCNNet: An Automatic Framework for the Detection of Parkinson’s Disease Using EEG Signals,” IEEE Sensors Journal, vol. 21, no. 15, pp. 17017-17024, 2021.
[CrossRef] [Google Scholar] [Publisher link]
[10] Abdulhamit Subasi et al., “EEG-Based Emotion Recognition Using Tunable Q Wavelet Transform and Rotation Forest Ensemble Classifier,” Biomedical Signal Processing and Control, vol. 68, pp. 1-8, 2021.
[CrossRef] [Google Scholar] [Publisher link]
[11] Geetanjali Sharma, Abhishek Parashar, and Amit M. Joshi, “DepHNN: A Novel Hybrid Neural Network for Electroencephalogram (EEG)-Based Screening of Depression,” Biomedical Signal Processing and Control, vol. 66, 2021.
[CrossRef] [Google Scholar] [Publisher link]
[12] Prabal Datta Barua et al., “Novel Automated PD Detection System Using Aspirin Pattern with EEG Signals,” Computers in Biology and Medicine, vol. 137, 2021.
[CrossRef] [Google Scholar] [Publisher link]
[13] Smith K. Khare, Varun Bajaj, and U. Rajendra Acharya, “Detection of Parkinson’s Disease Using Automated Tunable Q Wavelet Transform Technique with EEG signals,” Biocybernetics and Biomedical Engineering, vol. 41, no. 2, pp. 679-689, 2021.
[CrossRef] [Google Scholar] [Publisher link]
[14] Ravikiran Parameshwara et al., “Automated Parkinson's Disease Detection and Affective Analysis from Emotional EEG Signals,” Arxiv, pp. 1-12, 2022.
[CrossRef] [Google Scholar] [Publisher link]
[15] Ana M. Maitin, Juan Pablo Romero Muñoz, and Álvaro José García-Tejedor, “Survey of Machine Learning Techniques in the Analysis of EEG Signals for Parkinson’s Disease: A Systematic Review,” Applied Sciences, vol. 12, no. 14, pp. 1-33, 2022.
[CrossRef] [Google Scholar] [Publisher link]
[16] Sharaban Tahura et al., “Anomaly Detection in Electroencephalography Signal Using Deep Learning Model,” Proceedings of International Conference on Trends in Computational and Cognitive Engineering, pp. 205-217, 2020.
[CrossRef] [Google Scholar] [Publisher link]
[17] Ghita Amrani et al., “EEG Signal Analysis Using Deep Learning: A Systematic Literature Review,” 2021 Fifth International Conference on Intelligent Computing in Data Sciences, Fez, Morocco, pp. 1-8, 2021.
[CrossRef] [Google Scholar] [Publisher link]
[18] Seyed Alireza Khoshnevis, and Ravi Sankar, “Diagnosis of Parkinson’s Disease Using Higher Order Statistical Analysis of Alpha and Beta Rhythms,” Biomedical Signal Processing and Control, vol. 77, 2022.
[CrossRef] [Google Scholar] [Publisher link]
[19] M. Murugappan et al., Electroencephalogram Signals Based Emotion Classification in Parkinson’s Disease Using Recurrence Quantification Analysis and Non-Linear Classifiers, 1st ed., Computer-aided Design and Diagnosis Methods for Biomedical Applications, CRC Press, pp. 1-34, 2021.
[Google Scholar] [Publisher link]
[20] Ling Wang et al., “Multidimensional Emotion Recognition Based on Semantic Analysis of Biomedical EEG Signal for Knowledge Discovery in Psychological Healthcare,” Applied Sciences, vol. 11, no. 3, pp. 1-19, 2021.
[CrossRef] [Google Scholar] [Publisher link]
[21] Miguel Ángel Luján et al., “A Survey on EEG Signal Processing Techniques and Machine Learning: Applications to the Neurofeedback of Autobiographical Memory Deficits in Schizophrenia,” Electronics, vol. 10, no. 23, pp. 1-19, 2021.
[CrossRef] [Google Scholar] [Publisher link]
[22] Didar Dadebayev, Wei Wei Goh, and Ee Xion Tan, “EEG-Based Emotion Recognition: Review of Commercial EEG Devices and Machine Learning Techniques,” Journal of King Saud University-Computer and Information Sciences, vol. 34, no. 7, pp. 4385-4401, 2022.
[CrossRef] [Google Scholar] [Publisher link]