Intelligent Robotic Medical Assistive Device for Elderly Individuals Support
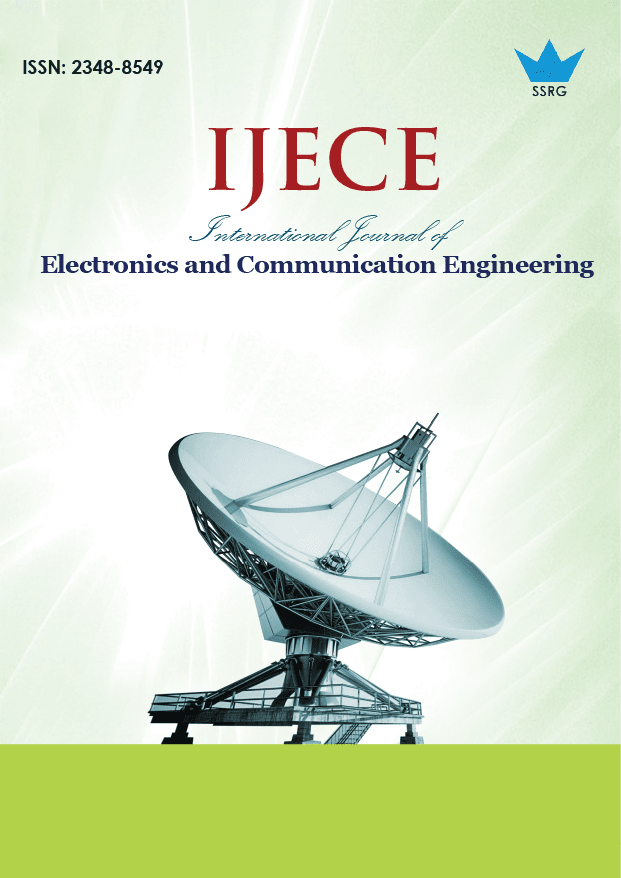
International Journal of Electronics and Communication Engineering |
© 2024 by SSRG - IJECE Journal |
Volume 11 Issue 11 |
Year of Publication : 2024 |
Authors : Chandrashekhar Kumar, T. Muthumanickam, T. Sheela |
How to Cite?
Chandrashekhar Kumar, T. Muthumanickam, T. Sheela, "Intelligent Robotic Medical Assistive Device for Elderly Individuals Support," SSRG International Journal of Electronics and Communication Engineering, vol. 11, no. 11, pp. 1-11, 2024. Crossref, https://doi.org/10.14445/23488549/IJECE-V11I11P101
Abstract:
This paper discusses trends in technology like Artificial Intelligence and machine learning algorithms in developing intelligent robotic systems. It focuses on attribute-picking points, classification, and fuzzy rule-based decision-making in settings for robot actuation planning. The system uses a Natural Language Processing-based User Interface, cameras, and image processing modules. It proposes two new feature selection and classification algorithms, Intelligent Voice to Text Conversion and Fuzzy Temporal Rule-based Semantic Analysis Algorithm. The system also introduces three new algorithms for object detection and grasping. Robots with assistive technology may help with senior or geriatric care, but their ability to track objects, estimate motion, and estimate poses a barrier. Although researchers have suggested real-time posture estimates as a dependable option, traditional tracking techniques still highly value stance. The volume of data, extensive processing duties, and start-up all contribute to the complexity of real-time tracking. An innovative mobile robot system designed to assist has been put forth to enable elderly individuals to live longer, safer lives in their homes. The study aims to address these concerns and develop technology that meets the needs of senior citizens and geriatrics.
Keywords:
Artificial Intelligence, Machine Learning, Medical Assistive, Intelligent Systems, Robotics, Elderly Support.
References:
[1] Ossama Abdel-Hamid et al., “Convolutional Neural Networks for Speech Recognition,” IEEE/ACM Transactions on Audio, Speech, and Language Processing, vol. 22, no.10, pp. 1533-1545, 2014.
[CrossRef] [Google Scholar] [Publisher Link]
[2] Abdulla Mohamed, Chenguang Yang, and Angelo Cangelosi, “Stereo Vision Based Object Tracking Control for a Movable Robot Head,” IFAC-PapersOnLine, vol. 49, no. 5, pp. 155-162, 2016.
[CrossRef] [Google Scholar] [Publisher Link]
[3] Prabha Adhikari, “Geriatric Health Care in India - Unmet Needs and the Way Forward,” Architecture Medical Health Science, vol. 5, no. 1, pp. 112-114, 2017.
[CrossRef] [Google Scholar] [Publisher Link]
[4] Akash Bapat, Enrique Dunn, and Jan-Michael Frahm, “Towards Kilo-Hertz 6-DoF Visual Tracking Using an Egocentric Cluster of Rolling Shutter Cameras,” IEEE Transactions on Visualization and Computer Graphics, vol. 22, no. 11, pp. 2358-2367, 2016.
[CrossRef] [Google Scholar] [Publisher Link]
[5] Alexandre Campeau-Lecours et al., “Kinova Modular Robot Arms for Service Robotics Applications,” International Journal of Robotics Applications and Technologies, vol. 5, no. 2, pp. 49-71, 2017.
[CrossRef] [Google Scholar] [Publisher Link]
[6] Ali Bou Nassif et al., “Speech Recognition Using Deep Neural Networks: A Systematic Review,” IEEE Access, vol. 7, pp. 19143-19165, 2019.
[CrossRef] [Google Scholar] [Publisher Link]
[7] Amin Atrash et al., “Development and Validation of a Robust Speech Interface for Improved Human-Robot Interaction,” International Journal of Social Robotics, vol. 1, no. 4, pp. 345-356, 2009.
[CrossRef] [Google Scholar] [Publisher Link]
[8] Anchal A. Solio, and Siddharth A. Ladhake, “A Review of Query Image in Content-Based Image Retrieval,” International Journal of Advanced Research in Computer Engineering and Technology, vol. 2, no. 4, pp. 1619-1622, 2013.
[Google Scholar]
[9] James A. Anderson, An Introduction to Neural Networks, Cambridge: MIT Press, pp. 1-650, 1995.
[Google Scholar] [Publisher Link]
[10] Archana Kale, and Shefali Sonavane, “Optimal Feature Subset Selection for Fuzzy Extreme Learning Machine Using Genetic Algorithm with Multilevel Parameter Optimization,” Proceeding of the IEEE International Conference on Signal and Image Processing Applications, Kuching, Malaysia, pp. 445-450, 2017.
[CrossRef] [Google Scholar] [Publisher Link]
[11] Aung Myat San, Wut Yi Win, and Saint Saint Pyone, “ANFIS-Based Visual Pose Estimation of Uncertain Robotic Arm Using Two Uncalibrated Cameras,” International Journal of Wireless Communications and Mobile Computing, vol. 6, no.1, pp. 20-30, 2018.
[CrossRef] [Google Scholar] [Publisher Link]
[12] Ronald T. Azuma, “A Survey of Augmented Reality,” Presence: Teleoperators and Virtual Environments, vol. 6, pp. 355-385, 1997.
[Google Scholar] [Publisher Link]
[13] R. Azuma et al., “Recent Advances in Augmented Reality,” IEEE Computer Graphics and Applications, vol. 21, no. 6, pp. 34-47, 2001.
[CrossRef] [Google Scholar] [Publisher Link]
[14] Aaron Bangor, Philip T. Kortum, and James T. Miller, “The System Usability Scale (Sus): An Empirical Evaluation,” International Journal of Human-Computer Interaction, vol. 24, no. 6, pp. 574-594, 2008.
[CrossRef] [Google Scholar] [Publisher Link]
[15] Barbora Zahradnikova, Sona Duchovicova, and Peter Schreiber, “Image Mining: Review and New Challenges,” International Journal of Advanced Computer Science and Applications, vol. 6, no. 7, pp. 242-246, 2015.
[CrossRef] [Google Scholar] [Publisher Link]
[16] Emanuele Bastianelli et al., “Effective and Robust Natural Language Understanding for Human-Robot Interaction,” European Conference on Artificial Intelligence, pp. 57-62, 2014.
[Google Scholar] [Publisher Link]
[17] Emanuele Bastianelli et al., “Structured Learning for Spoken Language Understanding in Human-Robot Interaction,” The International Journal of Robotics Research, vol. 36, no. 5-7, pp. 660-683, 2017.
[CrossRef] [Google Scholar] [Publisher Link]
[18] A.K. Bejczy, W.S. Kim, and S.C. Venema, “The Phantom Robot: Predictive Displays for Teleoperation with Time Delay,” IEEE International Conference on Robotics and Automation, Cincinnati, OH, USA, vol. 1, pp. 546-551, 1990.
[CrossRef] [Google Scholar] [Publisher Link]
[19] Linda M. Beuscher et al., “Socially Assistive Robots,” Journal of Gerontology Nursing Diagnosis, vol. 43, no. 12, pp. 35-43, 2017.
[CrossRef] [Google Scholar] [Publisher Link]
[20] A. Bicchi, and V. Kumar, “Robotic Grasping and Contact: A Review,” Proceedings 2000 ICRA. Millennium Conference IEEE International Conference on Robotics and Automation. Symposia Proceedings, San Francisco, CA, USA, vol. 1, pp. 348-353, 2000.
[CrossRef] [Google Scholar] [Publisher Link]
[21] Jonathan Bidwell, Alexandra Holloway, and Scott Davidoff, “Measuring Operator Anticipatory Inputs in Response to Time Delay for Teleoperated Human-Robot Interfaces,” Proceedings of the SIGCHI Conference on Human Factors in Computing Systems, New York, NY, USA, pp. 1467-1470, 2014.
[CrossRef] [Google Scholar] [Publisher Link]
[22] Gary M. Bone, Andrew Lambert, and Mark Edwards, “Automated Modeling and Robotic Grasping of Unknown Three-Dimensional Objects,” IEEE International Conference on Robotics and Automation, Pasadena, CA, USA, pp. 292-298, 2008.
[CrossRef] [Google Scholar] [Publisher Link]
[23] Cynthia Breazeal, Designing Sociable Robots, MIT Press, Cambridge, pp. 1-282, 2004.
[Google Scholar] [Publisher Link]
[24] Joost Broekens, Marcel Heerink, and Henk Rosendal, “Assistive Social Robots in Elderly Care: A Review,” Gerontechnology, vol. 8, no. 2, pp. 94-103, 2009.
[CrossRef] [Google Scholar] [Publisher Link]
[25] Charles G. Burgar et al., “Development of Robots for Rehabilitation Therapy: The Palo Alto VA/Stanford Experience,” Journal of Rehabilitation Research and Development, vol. 37, no. 6, pp. 663-673, 2002.
[Google Scholar] [Publisher Link]
[26] Filippo Cavallo et al., “Development of a Socially Believable Multi-Robot Solution from Town to Home,” Cognitive Computation, vol. 6, no. 4, pp. 954-967, 2014.
[CrossRef] [Google Scholar] [Publisher Link]
[27] Jun-Wei Chang et al., “Implementation of an Object-Grasping Robot Arm Using Stereo Vision Measurement and Fuzzy Control,” International Journal Fuzzy System, vol. 17, no. 2, pp. 193-205, 2015.
[CrossRef] [Google Scholar] [Publisher Link]
[28] Changjoo Nam et al., “A Software Architecture for Service Robots Manipulating Objects in Human Environments,” IEEE Access, vol. 8, pp. 117900-17920, 2020.
[CrossRef] [Google Scholar] [Publisher Link]
[29] Chaobin Liu, Yuexiang Yang, and Chuan Tang, “An Improved Method for Multiclass Support Vector Machines,” ACM International Conference on Measuring Technology and Mechatronics Automation, pp. 504-508, 2010.
[CrossRef] [Google Scholar] [Publisher Link]
[30] Yixin Chen, and J.Z. Wang, “Support Vector Learning for Fuzzy Rule-Based Classification Systems,” IEEE Transactions on Fuzzy Systems, vol. 11, no. 6, pp. 716-728, 2003.
[CrossRef] [Google Scholar] [Publisher Link]
[31] Yuehui Chen, Ajith Abraham, and Bo Yang, “Feature Selection and Classification using Flexible Neural Tree,” Neurocomputing, vol. 70, no. 1-3, pp. 305-313, 2006.
[CrossRef] [Google Scholar] [Publisher Link]
[32] Ching-Yu Chiu et al., “A Wireless Steady State Visually Evoked Potential-Based BCI Eating Assistive System,” International Joint Conference on Neural Networks, Anchorage, AK, USA, pp. 3003-3007, 2017.
[CrossRef] [Google Scholar] [Publisher Link]
[33] Chotirat Ann Ratanamahatana, and Dimitrios Gunopulos, “Feature Selection for the Naive Bayesian Classifier Using Decision Trees,” Applied Artificial Intelligence, vol. 17, no. 5-6, pp. 475-487, 2003.
[CrossRef] [Google Scholar] [Publisher Link]
[34] Cheng-Shiu Chung et al., “Performance Evaluation of a Mobile Touchscreen Interface for Assistive Robotic Manipulators: A Pilot Study,” Topics in Spinal Cord Injury Rehabilitation, vol. 23, no. 2, pp. 131-139, 2017.
[CrossRef] [Google Scholar] [Publisher Link]
[35] Dan Ciregan, Ueli Meier, and Jürgen Schmidhuber, “Multi-Column Deep Neural Networks for Image Classification,” IEEE Conference on Computer Vision and Pattern Recognition, Providence, RI, USA, pp. 3642-3649, 2012.
[CrossRef] [Google Scholar] [Publisher Link]
[36] Kurt E. Clothier, and Ying Shang, “A Geometric Approach for Robotic Arm Kinematics with Hardware Design, Electrical Design, and Implementation,” Journal of Robotics, vol. 2010, no. 1, pp. 1-10, 2010.
[CrossRef] [Google Scholar] [Publisher Link]
[37] Ulysse Côté-Allard et al., “Transfer Learning for sEMG Hand Gestures Recognition Using Convolutional Neural Networks,” IEEE International Conference on Systems, Man, and Cybernetics, Banff, AB, Canada, pp. 1663-1668, 2017.
[CrossRef] [Google Scholar] [Publisher Link]
[38] M. Cowling, and R. Sitte, “Analysis of Speech Recognition Techniques for Use in a Nonspeech Sound Recognition System,” Digital Signal Processing for Communication Systems, vol. 703, no. 1, pp. 31-46, 2002.
[Google Scholar]
[39] S. Davis, and P. Mermelstein, “Comparison of Parametric Representations for Monosyllabic Word Recognition in Continuously Spoken Sentences,” IEEE Transactions on Acoustics, Speech and Signal Processing, vol. 28, no. 4, pp. 357-366, 1980.
[CrossRef] [Google Scholar] [Publisher Link]
[40] Steven B. Davis, and Paul Mermelstein, “Comparison of Parametric Representations for Monosyllabic Word Recognition in Continuously Spoken Sentences,” Readings in Speech Recognition, Elsevier, pp. 65-74, 1990.
[CrossRef] [Google Scholar] [Publisher Link]
[41] Deepu Rani, and Monica Goya, “A Research Paper on Content-Based Image Retrieval System Using Improved SVM Technique,” International Journal of Advanced Research in Computer Science and Software Engineering, vol. 4, no. 10, pp. 683-686, 2014.
[Google Scholar]