A Novel Multimodal Framework for Early Detection of Alzheimer’s Disease Using Deep Learning
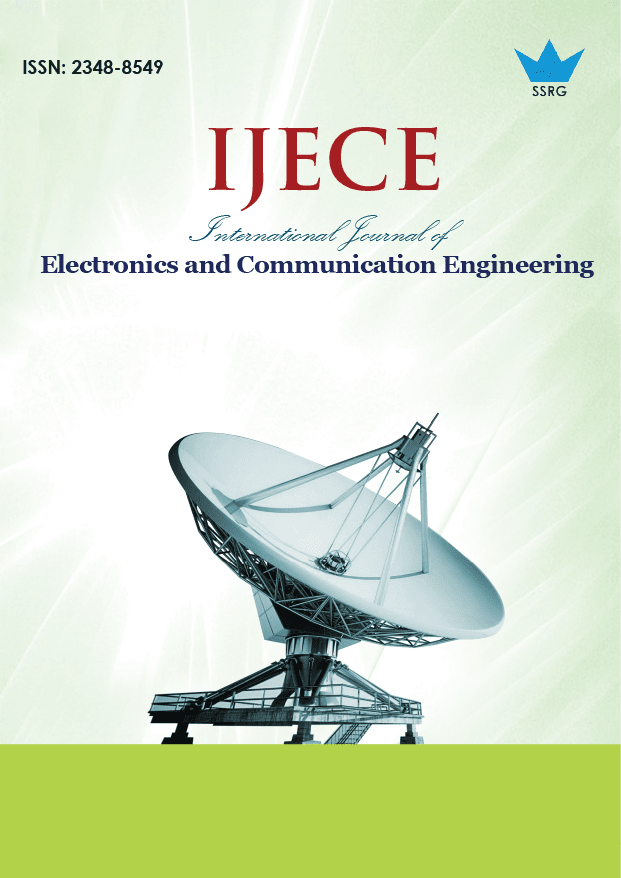
International Journal of Electronics and Communication Engineering |
© 2024 by SSRG - IJECE Journal |
Volume 11 Issue 11 |
Year of Publication : 2024 |
Authors : Tatwadarshi P. Nagarhalli, Sanket Patil, Vishal Pande, Uday Aswalekar, Prafulla Patil |
How to Cite?
Tatwadarshi P. Nagarhalli, Sanket Patil, Vishal Pande, Uday Aswalekar, Prafulla Patil, "A Novel Multimodal Framework for Early Detection of Alzheimer’s Disease Using Deep Learning," SSRG International Journal of Electronics and Communication Engineering, vol. 11, no. 11, pp. 12-25, 2024. Crossref, https://doi.org/10.14445/23488549/IJECE-V11I11P102
Abstract:
Alzheimer’s Disease (AD) is a progressive neurodegenerative disorder that poses significant challenges in its early diagnosis, often leading to delayed treatment and poorer outcomes for patients. Traditional diagnostic methods, typically reliant on single data modalities, fall short of capturing the multifaceted nature of the disease. In this paper, we propose a novel multimodal framework for the early detection of AD that integrates data from three primary sources: MRI imaging, cognitive assessments, and biomarkers. This framework employs Convolutional Neural Networks (CNN) for analyzing MRI images and Long Short-Term Memory (LSTM) networks for processing cognitive and biomarker data. The system enhances diagnostic accuracy and reliability by aggregating results from these distinct modalities using advanced techniques like weighted averaging, even in incomplete data. The multimodal approach not only improves the robustness of the detection process but also enables the identification of AD at its earliest stages, offering a significant advantage over conventional methods. The integration of biomarkers and cognitive tests is particularly crucial, as these can detect Alzheimer's long before the onset of clinical symptoms, thereby facilitating earlier intervention and potentially altering the course of the disease. This research demonstrates that the proposed framework has the potential to revolutionize the early detection of AD, paving the way for more timely and effective treatments.
Keywords:
Alzheimer’s Disease (AD) Detection, Early detection of AD, Multimodal framework, MRI imaging, Cognitive assessment, Biomarkers, Machine learning, Deep learning.
References:
[1] Alzheimer's Disease and Related Dementias (AD/ADRD), ASPE. [Online]. Available: https://aspe.hhs.gov/collaborations-committees-advisory-groups/napa/what-ad-adrd
[2] Alzheimer's Disease, Mayo Clinic. [Online]. Available: https://www.mayoclinic.org/diseases-conditions/alzheimers-disease/symptoms-causes/syc-20350447
[3] “2021 Alzheimer’s Disease Facts and Figures,” Alzheimer's Association, vol. 17, no. 3, pp. 327-406, 2021.
[CrossRef] [Publisher Link]
[4] K.S. Shaji et al., “The Dementia India Report 2010: Prevalence, Impact, Costs and Services for Dementia,” Alzheimer’s and Related Disorders Society of India, Report, pp. 1-84, 2010.
[Publisher Link]
[5] Michael W. Weiner et al., “The Alzheimer's Disease Neuroimaging Initiative 3: Continued Innovation for Clinical Trial Improvement,” Alzheimer's & Dementia, vol. 13, no. 5, pp. 561-571, 2017.
[CrossRef] [Google Scholar] [Publisher Link]
[6] Giovanni B. Frisoni et al., “The Clinical Use of Structural MRI in Alzheimer Disease,” Nature Reviews Neurology, vol. 6, no. 2, pp. 67-77, 2010.
[CrossRef] [Google Scholar] [Publisher Link]
[7] Marilyn S. Albert et al., “The Diagnosis of Mild Cognitive Impairment due to Alzheimer's Disease: Recommendations From the National Institute on Aging-Alzheimer's Association Workgroups on Diagnostic Guidelines for Alzheimer's Disease,” The Journal of the Alzheimer's Association, vol. 7, no. 3, pp. 270-279, 2011.
[CrossRef] [Google Scholar] [Publisher Link]
[8] Clifford R. Jack Jr et al., “NIA-AA Research Framework: Toward a Biological Definition of Alzheimer's Disease,” The Journal of the Alzheimer's Association, vol. 14, no. 4, pp. 535-562, 2018.
[CrossRef] [Google Scholar] [Publisher Link]
[9] Saman Sarraf et al., “DeepAD: Alzheimer’s Disease Classification via Deep Convolutional Neural Networks Using MRI and FMRI,” Biorxiv, pp. 1-32, 2017.
[CrossRef] [Google Scholar] [Publisher Link]
[10] Clifford R. Jack Jr et al., “Tracking Pathophysiological Processes in Alzheimer's Disease: An Updated Hypothetical Model of Dynamic Biomarkers,” The Lancet Neurology, vol. 12, no. 2, pp. 207-216, 2013.
[CrossRef] [Google Scholar] [Publisher Link]
[11] Michael W. Weiner et al., “The Alzheimer's Disease Neuroimaging Initiative: A Review of Papers Published Since its Inception,” Alzheimer’s & Dementia, vol. 9, no. 5, pp. e111-e194, 2013.
[CrossRef] [Google Scholar] [Publisher Link]
[12] Hadeer A. Helaly, Mahmoud Badawy, and Amira Y. Haikal, “Deep Learning Approach for Early Detection of Alzheimer’s Disease,” Cognitive Computation, vol. 14, pp. 1711-1727, 2022.
[CrossRef] [Google Scholar] [Publisher Link]
[13] Julia Krüger et al., “Voxel-Based Morphometry in Single Subjects without a Scanner-Specific Normal Database Using a Convolutional Neural Network,” European Radiology, vol. 34, pp. 3578-3587, 2024.
[CrossRef] [Google Scholar] [Publisher Link]
[14] Muhammad Irfan, Seyed Shahrestani, and Mahmoud Elkhodr, “Early Detection of Alzheimer's Disease Using Cognitive Features: A Voting-Based Ensemble Machine Learning Approach,” IEEE Engineering Management Review, vol. 51, no. 1, pp. 16-25, 2023.
[CrossRef] [Google Scholar] [Publisher Link]
[15] Kulvinder Panesar, and María Beatriz Pérez Cabello de Alba, “Natural Language Processing-Driven Framework for the Early Detection of Language and Cognitive Decline,” Language and Health, vol. 1, no. 2, pp. 20-35, 2023.
[CrossRef] [Google Scholar] [Publisher Link]
[16] Ellen Grober et al., “Free and Cued Selective Reminding Identifies Very Mild Dementia in Primary Care,” Alzheimer Disease & Associated Disorders, vol. 24, no. 3, pp. 284-290, 2010.
[CrossRef] [Google Scholar] [Publisher Link]
[17] R.C. Petersen et al., “Prevalence of Mild Cognitive Impairment is Higher in Men: The Mayo Clinic Study of Aging,” Neurology, vol. 75, no. 10, pp. 889-897, 2010.
[CrossRef] [Google Scholar] [Publisher Link]
[18] Kaj Blennow, Harald Hampel, and Henrik Zetterberg, “Biomarkers in Amyloid-β Immunotherapy Trials in Alzheimer’s Disease,” Neurotherapeutics, vol. 39, no. 1, pp. 189-201, 2014.
[CrossRef] [Google Scholar] [Publisher Link]
[19] Harald Hampel et al., “Blood-Based Biomarkers for Alzheimer Disease: Mapping the Road to the Clinic,” Nature Reviews Neurology, vol. 14, no. 11, pp. 639-652, 2018.
[CrossRef] [Google Scholar] [Publisher Link]
[20] Anna Michela Gaeta et al., “Predicting Alzheimer's disease CSF Core Biomarkers: A Multimodal Machine Learning Approach,” Frontiers in Aging Neuroscience, vol. 16, pp. 1-22, 2024.
[CrossRef] [Google Scholar] [Publisher Link]
[21] Zhiqiang Wang et al., “Plasma Neurofilament Light Chain as a Predictive Biomarker for Post-Stroke Cognitive Impairment: A Prospective Cohort Study,” Frontiers in Aging Neuroscience, vol. 13, pp. 1-7, 2021.
[CrossRef] [Google Scholar] [Publisher Link]
[22] Matthias Mann et al., “Artificial Intelligence for Proteomics and Biomarker Discovery,” Cell Systems, vol. 12, no. 8, pp. 759-770, 2021.
[CrossRef] [Google Scholar] [Publisher Link]
[23] Laura M. Winchester et al., “Artificial Intelligence for Biomarker Discovery in Alzheimer's Disease and Dementia,” Alzheimer's & Dementia, vol. 19, no. 12, pp. 5860-5871, 2023.
[CrossRef] [Google Scholar] [Publisher Link]
[24] Heba M. AL-Bermany, and Sura Z. AL-Rashid, “Microarray Gene Expression Data for Detection Alzheimer’s Disease Using K-Means and Deep Learning,” 2021 7th International Engineering Conference “Research & Innovation Amid Global Pandemic" (IEC), Erbil, Iraq, pp. 13-19, 2021.
[CrossRef] [Google Scholar] [Publisher Link]
[25] Muaath Ebrahim AlMansoori et al., “Predicting Early Alzheimer’s with Blood Biomarkers and Clinical Features,” Scientific Reports, vol. 14, no. 1, pp. 1-15, 2024.
[CrossRef] [Google Scholar] [Publisher Link]
[26] Daniel S. Marcus et al., “Open Access Series of Imaging Studies (OASIS): Cross-sectional MRI Data in Young, Middle Aged, Nondemented, and Demented Older Adults,” Journal of Cognitive Neuroscience, vol. 19, no. 9, pp. 1498-1507, 2007. [CrossRef] [Google Scholar] [Publisher Link]
[27] Susanne G. Mueller et al., “The Alzheimer's Disease Neuroimaging Initiative,” Neuroimaging Clinics of North America, vol. 15, no. 4, pp. 869-877, 2005.
[CrossRef] [Google Scholar] [Publisher Link]
[28] T. Pamela J. LaMontagne et al., “OASIS-3: Longitudinal Neuroimaging, Clinical, and Cognitive Dataset for Normal Aging and Alzheimer Disease,” MedRxiv, pp. 1-37, 2019.
[CrossRef] [Google Scholar] [Publisher Link]
[29] Duane L. Beekly et al., “The National Alzheimer's Coordinating Center (NACC) Database: The Uniform Data Set,” Alzheimer Disease & Associated Disorders, vol. 21, no. 3, pp. 249-258, 2007.
[CrossRef] [Google Scholar] [Publisher Link]
[30] David A. Bennett et al., “Overview and Findings from the Religious Orders Study,” Current Alzheimer Research, vol. 9, no. 6, pp. 628-645, 2012.
[CrossRef] [Google Scholar] [Publisher Link]
[31] Leslie M. Shaw et al., “Cerebrospinal Fluid Biomarker Signature in Alzheimer's Disease Neuroimaging Initiative Subjects,” Annals of Neurology, vol. 65, no. 4, pp. 403-413, 2009.
[CrossRef] [Google Scholar] [Publisher Link]