Early Phase, Multi Diseases Detection, Using AI & Intelligent Hybrid Supervised Machine Learning Classifier Model
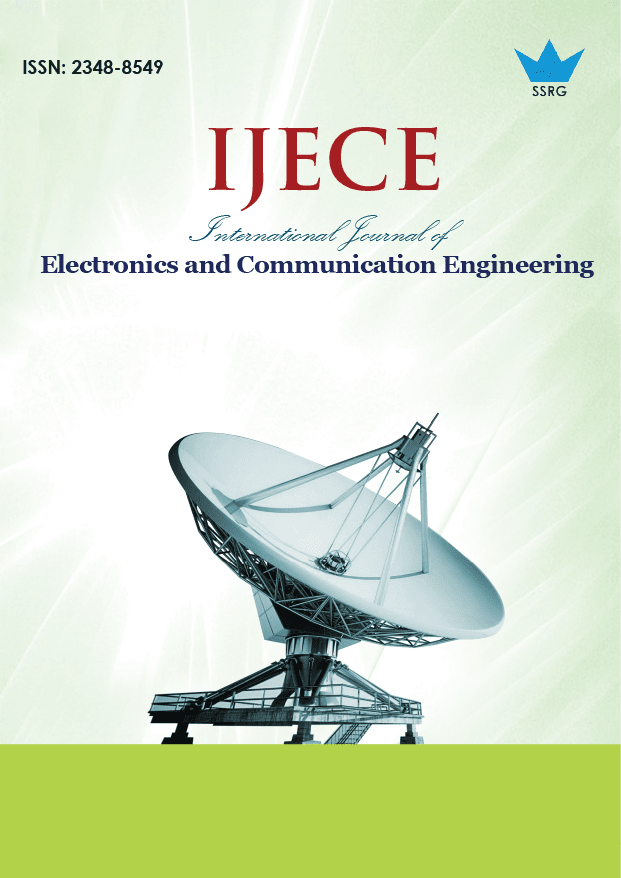
International Journal of Electronics and Communication Engineering |
© 2024 by SSRG - IJECE Journal |
Volume 11 Issue 11 |
Year of Publication : 2024 |
Authors : Anandkumar A. Sutariya, Dushyantsinh B. Rathod |
How to Cite?
Anandkumar A. Sutariya, Dushyantsinh B. Rathod, "Early Phase, Multi Diseases Detection, Using AI & Intelligent Hybrid Supervised Machine Learning Classifier Model," SSRG International Journal of Electronics and Communication Engineering, vol. 11, no. 11, pp. 45-53, 2024. Crossref, https://doi.org/10.14445/23488549/IJECE-V11I11P105
Abstract:
The healthcare industry generates vast amounts of data processed using specific techniques. Data mining is key to the healthcare industry's illness prediction process. The patient should be required to undergo a number of tests in order to diagnose the condition. The two main organ diseases that affect humans are kidney and liver diseases. Regardless, the two well-known conditions affecting people nowadays are Chronic Kidney Disease (CKD) and Chronic Liver Disease (CLD). A small number of people pass away these days due to chronic liver and kidney diseases. These are the most common illnesses on the earth and are real infections. In addition, the progressive degeneration of the kidney and liver is referred to as chronic kidney disease and chronic liver disease, respectively. A promising technique that aids in early disease identification and may support medical practitioners in their decision-making is machine learning. This study examines data mining techniques that can be applied to forecast conditions such as liver and kidney disorders. Using a dataset for training and testing, we compute the precision of machine learning calculations for predicting kidney and liver infection. We found that the proposed hybrid classifier model, K-Nearest Neighbor, Naïve Bayesian, and Decision Tree methods all had superior accuracy. This research aims to develop a framework to more accurately forecast a patient's risk of developing kidney and liver problems. The suggested methodology yielded higher results than other methods under consideration, scoring 99.24% and 99.96 for CKD and CLD, respectively. Furthermore, data pick-up and other attribute evaluators have been included to show the framework's tall execution with the fewest possible features.
Keywords:
Chronic Kidney Disease, Chronic Liver Disease, Classification, Prediction, Hybrid classifier model, K-Nearest Neighbor, Decision Tree, Bayesian classifier, Machine Learning and AI techniques.
References:
[1] Saurabh Pal, “Prediction for Chronic Kidney Disease by Categorical and Non Categorical Attributes Using Different Machine Learning Algorithms,” Multimedia Tools and Applications, vol. 82, pp. 41253-41266, 2023.
[CrossRef] [Google Scholar] [Publisher Link]
[2] Hira Khalid et al., “Machine Learning Hybrid Model for the Prediction of Chronic Kidney Disease,” Computational Intelligence and Neuroscience, vol. 2023, no. 1, pp. 1-14, 2023.
[CrossRef] [Google Scholar] [Publisher Link]
[3] Zahid Ullah, and Mona Jamjoom, “Early Detection and Diagnosis of Chronic Kidney Disease Based on Selected Predominant Features,” Journal of Healthcare Engineering, vol. 2023, no. 1, pp. 1-9, 2023.
[CrossRef] [Google Scholar] [Publisher Link]
[4] Ariful Islam, Ziaul Hasan Majumder, and Alomgeer Hussein, “Chronic Kidney Disease Prediction Based on Machine Learning Algorithms,” Journal of Pathology Informatics, vol. 14, pp. 1-12, 2023.
[CrossRef] [Google Scholar] [Publisher Link]
[5] Dibaba Adeba Debal, and Tilahun Melak Sitote, “Chronic Kidney Disease Prediction using Machine Learning Techniques,” Journal of Big Data, vol. 9, pp. 1-19, 2022.
[CrossRef] [Google Scholar] [Publisher Link]
[6] Venugopal Reddy Modhugua, and Sivakumar Ponnusamy, “Comparative Analysis of Machine Learning Algorithms for Liver Disease Prediction: SVM, Logistic Regression, and Decision Tree,” Asian Journal of Research in Computer Science, vol. 17, no. 6, pp. 188-201, 2024.
[CrossRef] [Google Scholar] [Publisher Link]
[7] Ahmet Ercan Topcu, Ersin Elbasi, and Yehia Ibrahim Alzoubi, “Machine Learning-Based Analysis and Prediction of Liver Cirrhosis,” 2024 47th International Conference on Telecommunications and Signal Processing, Prague, Czech Republic, pp. 191-194, 2024.
[CrossRef] [Google Scholar] [Publisher Link]
[8] Mandakini Priyadarshani Behera et al., “A Hybrid Machine Learning Algorithm for Heart and Liver Disease Prediction Using Modified Particle Swarm Optimization with Support Vector Machine,” Procedia Computer Science, vol. 218, pp. 818-827, 2023.
[CrossRef] [Google Scholar] [Publisher Link]
[9] Abdul Quadir et al., “Enhanced Preprocessing Approach Using Ensemble Machine Learning Algorithms for Detecting Liver Disease,” Biomedicines, vol. 11, no. 2, pp. 1-23, 2023.
[CrossRef] [Google Scholar] [Publisher Link]
[10] Ruhul Amin et al., “Prediction of Chronic Liver Disease Patients Using Integrated Projection Based Statistical Feature Extraction with Machine Learning Algorithms,” Informatics in Medicine Unlocked, vol. 36, pp. 1-11, 2023.
[CrossRef] [Google Scholar] [Publisher Link]
[11] J. Snegha et al., “Chronic Kidney Disease Prediction Using Data Mining,” 2020 International Conference on Emerging Trends in Information Technology and Engineering (ic-ETITE), Vellore, India, pp. 1-5, 2020.
[CrossRef] [Google Scholar] [Publisher Link]
[12] Rahul Gupta et al., “Performance Analysis of Machine Learning Classifier for Predicting Chronic Kidney Disease,” 2020 International Conference for Emerging Technology (INCET), Belgaum, India, pp. 1-4, 2020.
[CrossRef] [Google Scholar] [Publisher Link]
[13] Shubham Vashisth, Ishika Dhall, and Shipra Saraswat, “Chronic Kidney Disease (CKD) Diagnosis using Multi-Layer Perceptron Classifier,” 2020 10th International Conference on Cloud Computing, Data Science & Engineering (Confluence), Noida, India, pp. 346-350, 2020.
[CrossRef] [Google Scholar] [Publisher Link]
[14] Jerlin Rubini Lambert, Pramila Arulanthu, and Eswaran Perumal, “Identification of Nominal Attributes for Intelligent Classification of Chronic Kidney Disease using Optimization Algorithm,” 2020 International Conference on Communication and Signal Processing (ICCSP), Chennai, India, pp. 119-125, 2020.
[CrossRef] [Google Scholar] [Publisher Link]
[15] Pramila Arulanthu, and Eswaran Perumal, “Predicting the Chronic Kidney Disease using Various Classifiers,” 2019 4th International Conference on Electrical, Electronics, Communication, Computer Technologies and Optimization Techniques (ICEECCOT), Mysuru, India, pp. 70-75, 2019.
[CrossRef] [Google Scholar] [Publisher Link]
[16] Qiong Bai et al., “Machine Learning to Predict End Stage Kidney Disease in Chronic Kidney Disease,” Scientific Reports, vol. 12, pp. 1-8, 2022.
[CrossRef] [Google Scholar] [Publisher Link]