The Convergence of Deep Learning and ICT: Revolutionizing Student Performance Prediction
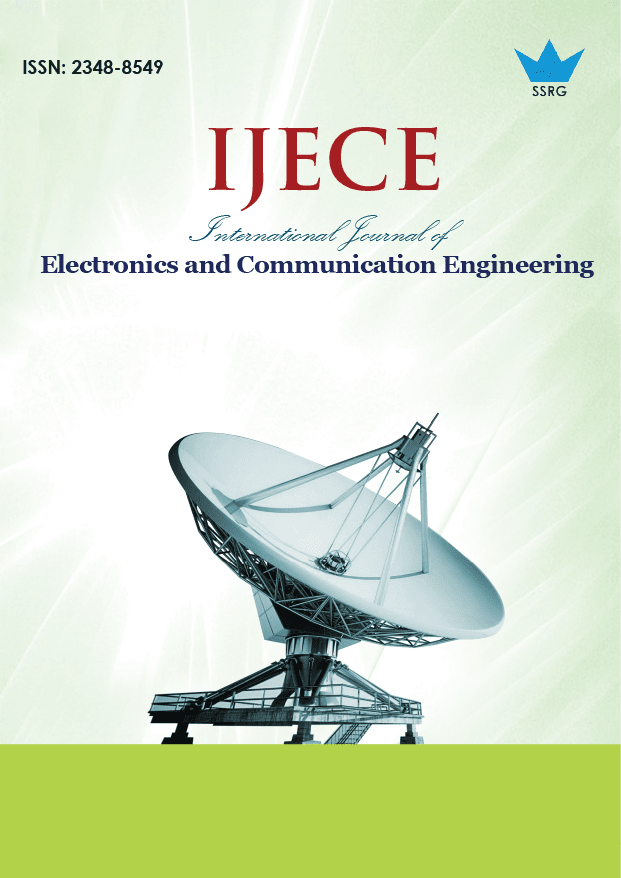
International Journal of Electronics and Communication Engineering |
© 2024 by SSRG - IJECE Journal |
Volume 11 Issue 11 |
Year of Publication : 2024 |
Authors : L. Ashish, G. Anitha |
How to Cite?
L. Ashish, G. Anitha, "The Convergence of Deep Learning and ICT: Revolutionizing Student Performance Prediction," SSRG International Journal of Electronics and Communication Engineering, vol. 11, no. 11, pp. 79-94, 2024. Crossref, https://doi.org/10.14445/23488549/IJECE-V11I11P108
Abstract:
Education includes transferring knowledge, integral judgment, and mature wisdom in addition to teaching and practising certain skills. Empowering educators and policymakers to make creative decisions that develop better student engagement, achievement, and equity in education, which ultimately prepares students for future challenges and opportunities, is crucial. Integrating Information and Communication Technology (ICT) into education boosts the learning capabilities that impact societal development, economic prosperity, and individual growth. This study aims to predict student performance using a Deep Neural Network (DNN) optimized with a feature optimization technique for effectively predicting student outcomes on ICT-enhanced learning. The dataset includes various attributes like gender, language skill, computer knowledge, learning type, location, and subject scores. The study evaluates the effects of ICT-enabled learning and finds critical elements impacting student performance by thoroughly examining the dataset and the neural network application. The proposed DNN employs a sequential architecture with multiple hidden layers with a classification accuracy of 93.0%, precision of 93.21%, recall of 93.0%, and an F1-score of 92.91. These findings highlight the model's high effectiveness in predicting student grades. This research underscores the potential of ICT-enhanced education to improve learning outcomes. It provides a robust predictive tool for early detection of at-risk students, thereby enabling prompt and targeted interventions.
Keywords:
Academic Success, Deep Neural Network, Education, Information and Communication Technology, Learning Type.
References:
[1] Raju Kumar, “Convergence of ICT and Education,” International Journal of Information and Communication Engineering, vol. 2, no. 4, pp. 300-303, 2008.
[Google Scholar]
[2] Pieter Hogenbirk, “ICT in Education: Literacy, Enhancement and Personalization,” UNESCO International Bureau of Education, pp. 1-24, 2016.
[Google Scholar]
[3] Miri Barak, “Transition from Traditional to ICT-Enhanced Learning Environments in Undergraduate Chemistry Courses,” Computers & Education, vol. 48, no. 1, pp. 30-43, 2007.
[CrossRef] [Google Scholar] [Publisher Link]
[4] Samuel-Soma M. Ajibade et al., “Utilization of Ensemble Techniques for Prediction of the Academic Performance of Students,” Journal of Optoelectronics Laser, vol. 41, no. 6, pp. 48-54, 2022.
[Google Scholar] [Publisher Link]
[5] Siti Dianah Abdul Bujang et al., “Multiclass Prediction Model for Student Grade Prediction Using Machine Learning,” IEEE Access, vol. 9, pp. 95608-95621, 2021.
[CrossRef] [Google Scholar] [Publisher Link]
[6] Ahmet Göçen, and Mehmet Akın Bulut, “Teaching Ethics in Teacher Education: ICT-Enhanced, Case-Based and Active Learning Approach with Continuous Formative Assessment,” Journal of Academic Ethics, vol. 22, pp. 447-465, 2024.
[CrossRef] [Google Scholar] [Publisher Link]
[7] Fayiza Parveen et al., “The Role of Information and Communication Technology at the Intermediate level in District Sialkot,” International Journal of Human and Society, vol. 4, no. 1, pp. 287-300, 2024.
[Google Scholar] [Publisher Link]
[8] Éva Karl et al., “Supporting the Pedagogical Evaluation of Educational Institutions with the Help of the WTCAi System,” Acta Polytechnica Hungarica, vol. 21, no. 3, pp. 125-142, 2024.
[Google Scholar] [Publisher Link]
[9] Vuong Thi Hoan, “An Exploratory Study on the Questionnaire Measuring EFL Teachers' Attitudes towards Information and Communication Technology (ICT) Integration in Primary School Classrooms,” EduLingua, vol. 9, no. 1, pp. 53-74, 2023.
[CrossRef] [Google Scholar] [Publisher Link]
[10] Stanislav Avsec, “Leveraging Systems Thinking, Engagement, and Digital Competencies to Enhance First-Year Architecture Students’ Achievement in Design-Based Learning,” Sustainability, vol. 15, no. 20, pp. 1-33, 2023.
[CrossRef] [Google Scholar] [Publisher Link]
[11] Amine Jaouadi, and Abderrahmane Maaradji, “ICT & Generative Artificial Intelligence Powered Hybrid Model for Future Education,” CADMUS, vol. 5, no. 3, pp. 61-75, 2024.
[Google Scholar] [Publisher Link]
[12] Marieke Versteijlen, and Arjen E.J. Wals, “Developing Design Principles for Sustainability-Oriented Blended Learning in Higher Education,” Sustainability, vol. 15, no. 10, pp. 1-25, 2023.
[CrossRef] [Google Scholar] [Publisher Link]
[13] Muntasir Hoq, Peter Brusilovsky, and Bita Akram, “Analysis of an Explainable Student Performance Prediction Model in an Introductory Programming Course,” Proceedings of the 16th International Conference on Educational Data Mining, pp. 79-90, 2023.
[CrossRef] [Google Scholar] [Publisher Link]
[14] Yudish Teshal Badal, and Roopesh Kevin Sungkur, “Predictive Modelling and Analytics of Students’ Grades Using Machine Learning Algorithms,” Education and Information Technologies, vol. 28, no. 3027-3057, 2023.
[CrossRef] [Google Scholar] [Publisher Link]
[15] Fatima Ahmed Al-azazi, and Mossa Ghurab, “ANN-LSTM: A Deep Learning Model for Early Student Performance Prediction in MOOC,” Heliyon, vol. 9, no. 4, pp. 1-16, 2023.
[CrossRef] [Google Scholar] [Publisher Link]
[16] Essa Alhazmi, and Abdullah Sheneamer, “Early Predicting of Students Performance in Higher Education,” IEEE Access, vol. 11, pp. 27579-27589, 2023.
[CrossRef] [Google Scholar] [Publisher Link]
[17] Rajan Saluja, Munishwar Rai, and Rashmi Saluja, “Designing New Student Performance Prediction Model Using Ensemble Machine Learning,” Journal of Autonomous Intelligence, vol. 6, no. 1, pp. 1-12, 2023.
[CrossRef] [Google Scholar] [Publisher Link]
[18] Shah Hussain, and Muhammad Qasim Khan, “Student-Performulator: Predicting Students’ Academic Performance at Secondary and Intermediate Level Using Machine Learning,” Annals of Data Science, vol. 10, pp. 637-655, 2023.
[CrossRef] [Google Scholar] [Publisher Link]
[19] Claudia Galarce-Miranda, Diego Gormaz-Lobos, and Hanno Hortsch, “An Analysis of Students' Perceptions of the Educational Use of ICTs and Educational Technologies during the Online Learning,” International Journal of Engineering Pedagogy, vol. 12, no. 2, pp. 62-74, 2022.
[CrossRef] [Google Scholar] [Publisher Link]
[20] Raghad Alshabandar et al., “Students Performance Prediction in Online Courses Using Machine Learning Algorithms,” 2020 International Joint Conference on Neural Networks, Glasgow, UK, pp. 1-7, 2020.
[CrossRef] [Google Scholar] [Publisher Link]