IoT-Enhanced Machine Learning for Intelligent Energy Optimization and Predictive Management
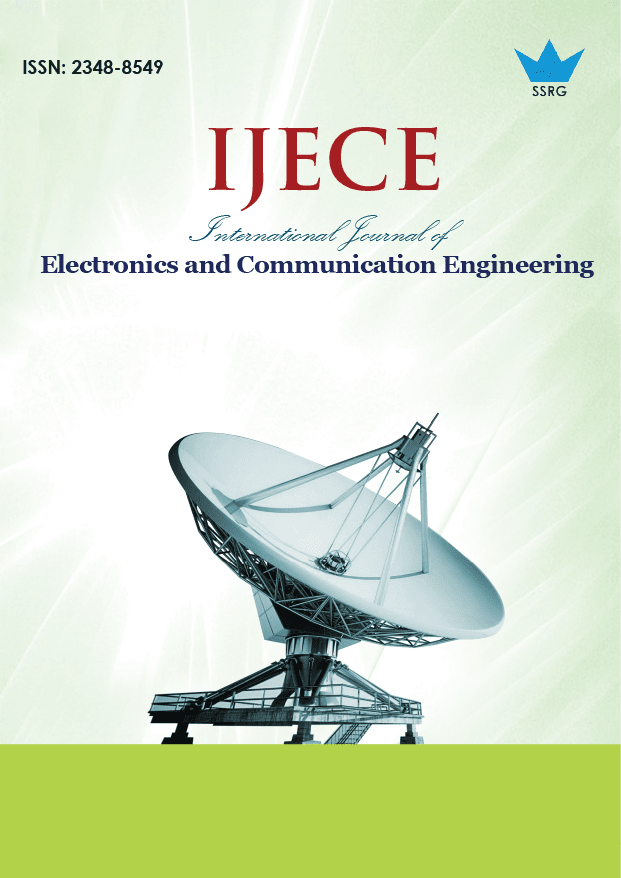
International Journal of Electronics and Communication Engineering |
© 2024 by SSRG - IJECE Journal |
Volume 11 Issue 11 |
Year of Publication : 2024 |
Authors : D. Rajalakshmi, K. Sudharson, R. Akhil Nair, M.A. Starlin |
How to Cite?
D. Rajalakshmi, K. Sudharson, R. Akhil Nair, M.A. Starlin, "IoT-Enhanced Machine Learning for Intelligent Energy Optimization and Predictive Management," SSRG International Journal of Electronics and Communication Engineering, vol. 11, no. 11, pp. 168-178, 2024. Crossref, https://doi.org/10.14445/23488549/IJECE-V11I11P115
Abstract:
IoT and machine learning systems are changing the energy management landscape since they make it possible to understand and analyze data with great detail. In this work, we develop EnerSense, a novel architecture that integrates IoT functionalities for smart meter data extraction with state-of-the-art Machine Learning techniques to manage energy consumption and project energy load. This model is based on the hybrid model, Random Forest and AutoRegressive Integrated Moving Average (RF-ARIMA), and it has an accuracy of 96% in determining the consumption behavior and investigating the outliers. Our framework enables wireless IoT integration and real-time data tracking for effective energy management while reducing cost regimes. Substantial empirical tests show about 20% energy wastage reduction, proving that the system can further improve energy efficiency. This solution enables utility companies to be equipped with meaningful energy usage strategies, presenting a cost-effective structure that optimizes resource use by meeting energy needs promptly and enhancing smart energy systems.
Keywords:
Smart meters, Wireless IoT, Machine Learning, Energy utilization optimization, Anomaly detection.
References:
[1] Elham Eskandarnia et al., ”Deep Learning Techniques for Smart Meter Data Analytics: A Review,” SN Computer Science, vol. 3, 2022.
[CrossRef] [Google Scholar] [Publisher Link]
[2] Lucas Pereira, Donovan Costa, and Miguel Ribeiro, “A Residential Labeled Dataset for Smart Meter Data Analytics,” Scientific Data, vol. 9, no. 1, pp. 1-11, 2022.
[CrossRef] [Google Scholar] [Publisher Link]
[3] Zhiyi Chen et al., “Control and Optimisation of Power Grids Using Smart Meter Data: A Review,” Sensors, vol. 23, no. 4, pp. 1-26, 2023.
[CrossRef] [Google Scholar] [Publisher Link]
[4] Maksymilian KochaĆski, Katarzyna Korczak, and Tadeusz Skoczkowski, “Technology Innovation System Analysis of Electricity Smart Metering in the European Union,” Energies, vol. 13, no. 4, pp. 1-25, 2020.
[CrossRef] [Google Scholar] [Publisher Link]
[5] Yi Wang et al., “Review of Smart Meter Data Analytics: Applications, Methodologies, and Challenges,” IEEE Transactions on Smart Grid, vol. 10, no. 3, pp. 3125-3148, 2018.
[CrossRef] [Google Scholar] [Publisher Link]
[6] Satya Jayadev Pappu et al., “Identifying Topology of Low Voltage Distribution Networks Based on Smart Meter Data,” IEEE Transactions on Smart Grid, vol. 9, no. 5, pp. 5113-5122, 2017.
[CrossRef] [Google Scholar] [Publisher Link]
[7] Zafar A. Khan, and Dilan Jayaweera, “Smart Meter Data Based Load Forecasting and Demand Side Management in Distribution Networks With Embedded PV Systems,” IEEE Access, vol. 8, pp. 2631-2644, 2019.
[CrossRef] [Google Scholar] [Publisher Link]
[8] Efrain Bernal Alzate et al., “Distribution System State Estimation to Support Coordinated Voltage-Control Strategies by Using Smart Meters,” IEEE Transactions on Power Systems, vol. 34, no. 6, pp. 5198-5207, 2019.
[CrossRef] [Google Scholar] [Publisher Link]
[9] Hong-Yen Tran, Jiankun Hu, and Hemanshu R. Pota, “Smart Meter Data Obfuscation with a Hybrid Privacy-Preserving Data Publishing Scheme Without a Trusted Third Party,” IEEE Internet of Things Journal, vol. 9, no. 17, pp. 16080-16095, 2022. [CrossRef] [Google Scholar] [Publisher Link]
[10] Yang Zhang, Tao Huang, and Ettore Francesco Bompard, “Big Data Analytics in Smart Grids: A Review,” Energy Informatics, vol. 1, pp. 1-24, 2018.
[CrossRef] [Google Scholar] [Publisher Link]
[11] Yi Wang et al., “A Novel Data Analytical Approach for False Data Injection Cyber-Physical Attack Mitigation in Smart Grids,” IEEE Access, vol. 5, pp. 26022-26033, 2017.
[CrossRef] [Google Scholar] [Publisher Link]
[12] Mohammad Navid Fekri, Katarina Grolinger, and Syed Mir, “Distributed Load Forecasting Using Smart Meter Data: Federated Learning with Recurrent Neural Networks,” International Journal of Electrical Power & Energy Systems, vol. 137, 2022. [CrossRef] [Google Scholar] [Publisher Link]
[13] R. Mathumitha, P. Rathika, and K. Manimala, “Intelligent Deep Learning Techniques for Energy Consumption Forecasting in Smart Buildings: A Review,” Artificial Intelligence Review, vol. 57, pp. 1-33, 2024.
[CrossRef] [Google Scholar] [Publisher Link]
[14] Hamdi A. Al-Jamimi et al., “Advancements in Household Load Forecasting: Deep Learning Model with Hyperparameter Optimization,” Electronics, vol. 12, no. 24, pp. 1-27, 2023.
[CrossRef] [Google Scholar] [Publisher Link]
[15] Kuo-Yang Wu et al., “Dynamic Energy Management Strategy of a Solar-and-Energy Storage-Integrated Smart Charging Station,” Applied Sciences, vol. 14, no. 3, pp. 1-17, 2024.
[CrossRef] [Google Scholar] [Publisher Link]
[16] Rohit Gupta, and Krishna Teerth Chaturvedi, “Adaptive Energy Management of Big Data Analytics in Smart Grids,” Energies, vol. 16, no. 16, pp. 1-19, 2023.
[CrossRef] [Google Scholar] [Publisher Link]
[17] Francisco Martínez-Álvarez et al., “A Survey on Data Mining Techniques Applied to Electricity-Related Time Series Forecasting,” Energies, vol. 8, no. 11, pp. 13162-13193, 2015.
[CrossRef] [Google Scholar] [Publisher Link]
[18] Zbigniew Leonowicz, and Michal Jasinski, “Machine Learning and Data Mining Applications in Power Systems,” Energies, vol. 15, no. 5, pp. 1-2, 2022.
[CrossRef] [Google Scholar] [Publisher Link]
[19] Hemn Barzan Abdalla, “A Brief Survey on Big Data: Technologies, Terminologies and Data-Intensive Applications,” Journal of Big Data, vol. 9, pp. 1-36, 2022.
[CrossRef] [Google Scholar] [Publisher Link]
[20] Konstantinos Demertzis et al., “Communication Network Standards for Smart Grid Infrastructures,” Network, vol. 1, no. 2, pp. 132-145, 2021.
[CrossRef] [Google Scholar] [Publisher Link]
[21] Ali Emrouznejad et al., “Analysis of Smart Meter Data with Machine Learning for Implications Targeted towards Residents,” International Journal of Urban Planning and Smart Cities, vol. 4, no. 1, pp. 1-22, 2023.
[CrossRef] [Google Scholar] [Publisher Link]
[22] M. Ravinder, and Vikram Kulkarni, “Intrusion Detection in Smart Meters Data Using Machine Learning Algorithms: A Research Report,” Frontiers in Energy Research, vol. 11, pp. 1-7, 2023.
[CrossRef] [Google Scholar] [Publisher Link]
[23] S.M. Sulaiman et al., “Smart Meter Data Analytics for Load Prediction Using Extreme Learning Machines and Artificial Neural Networks,” 2019 IEEE International Conference on Clean Energy and Energy Efficient Electronics Circuit for Sustainable Development, Krishnankoil, India, pp. 1-4, 2019.
[CrossRef] [Google Scholar] [Publisher Link]
[24] Behzad Najafi et al., “Building Characterization through Smart Meter Data Analytics: Determination of the Most Influential Temporal and Importance-in-Prediction Based Features,” Energy and Buildings, vol. 234, 2021.
[CrossRef] [Google Scholar] [Publisher Link]
[25] Xiufeng Liu et al., “Smart Meter Data Analytics: Systems, Algorithms, and Benchmarking,” ACM Transactions on Database Systems, vol. 42, no. 1, pp. 1-39, 2016.
[CrossRef] [Google Scholar] [Publisher Link]
[26] Seonghoon Jang, and Seung-Jung Shin, “Deep Learning-Based Smart Meter Wattage Prediction Analysis Platform,” International Journal of Advanced Smart Convergence, vol. 9, no. 4, pp. 173-178, 2020.
[CrossRef] [Google Scholar] [Publisher Link]
[27] Rudy Yulianto et al., “Exploratory Data Analysis for Building Energy Meters Using Machine Learning,” Journal of Telecommunication Electronics and Control Engineering, vol. 5, no. 2, pp. 144-57, 2023.
[CrossRef] [Google Scholar] [Publisher Link]
[28] Heba Allah Sayed, Ashraf William, and Adel Mounir Said, “Smart Electricity Meter Load Prediction in Dubai Using MLR, ANN, RF, and ARIMA,” Electronics, vol. 12, no. 2, pp. 1-25, 2023.
[CrossRef] [Google Scholar] [Publisher Link]
[29] Somalee Mitra, Basab Chakraborty, and Pabitra Mitra, “Smart Meter Data Analytics Applications for Secure, Reliable and Robust Grid System: Survey and Future Directions,” Energy, vol. 289, 2024.
[CrossRef] [Google Scholar] [Publisher Link]
[30] Taifeng Chen, and Chunbo Liu, “Soft Computing Based Smart Grid Fault Detection Using Computerised Data Analysis with Fuzzy Machine Learning Model,” Sustainable Computing: Informatics and Systems, vol. 41, 2024.
[CrossRef] [Google Scholar] [Publisher Link]