The Role of Recent Datasets in Network Threat Classification and Intrusion Detection Systems
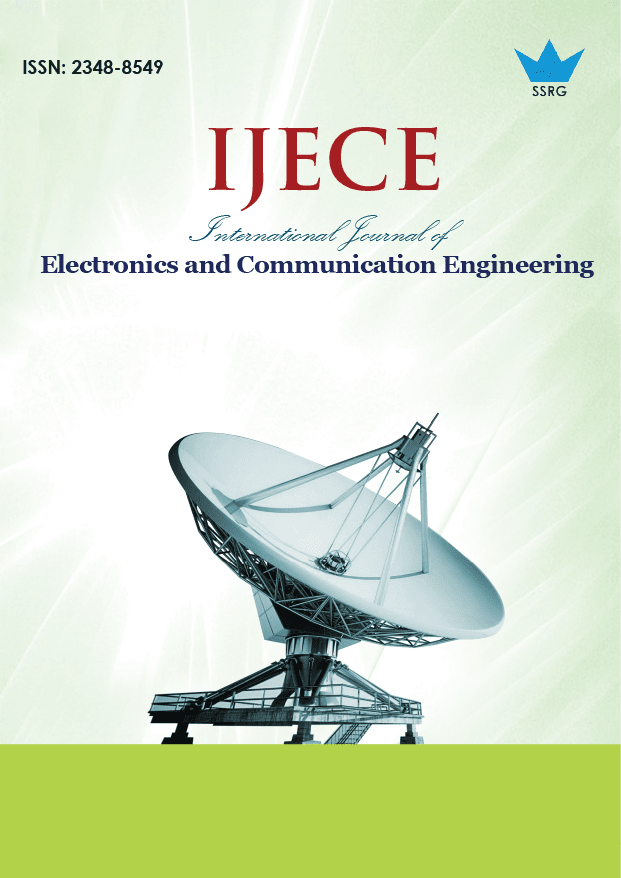
International Journal of Electronics and Communication Engineering |
© 2024 by SSRG - IJECE Journal |
Volume 11 Issue 11 |
Year of Publication : 2024 |
Authors : Priya Dasarwar |
How to Cite?
Priya Dasarwar, "The Role of Recent Datasets in Network Threat Classification and Intrusion Detection Systems," SSRG International Journal of Electronics and Communication Engineering, vol. 11, no. 11, pp. 179-195, 2024. Crossref, https://doi.org/10.14445/23488549/IJECE-V11I11P116
Abstract:
As our reliance upon gadgets and technology grows, one of the most important challenges of the twenty-first century is developing safe networks, systems, and applications. The complexity of today's networks and services is growing, and with it, so too are the risks that individuals and businesses must manage. Researchers have created a variety of anomaly detection solutions in order to mitigate the impact of these threats; nevertheless, current methods find it difficult to keep updated with the constantly changing nature of modern architectures and associated threats, including zero-day attacks. This research addresses existing dataset weaknesses and research gaps and their implications for advancing Network Intrusion Detection Systems (NIDS) and the rise in complex attacks. For that purpose, the current paper presents researchers with a survey of well-known datasets UNSW-NB15, RPL NIDS-17 and N_BaIoT-18 and an analysis of their utilization, associated network hazards and various detection approaches. Current IDS research is highlighted in the manuscript.
Keywords:
Data Set, Intrusion Detection, N_BaIoT-18, RPLNIDS-17, UNSW-NB15.
References:
[1] Hanan Hindy et al., “A Taxonomy of Network Threats and the Effect of Current Datasets on Intrusion Detection Systems,” IEEE Access, vol. 8, pp. 104650-104675, 2020.
[CrossRef] [Google Scholar] [Publisher Link]
[2] Markus Ring et al., “A Survey of Network-based Intrusion Detection Data Sets,” Computers & Security, vol. 86, pp. 147-167, 2019.
[CrossRef] [Google Scholar] [Publisher Link]
[3] Priya R. Maidamwar, Mahip M. Bartere, and Prasad P. Lokulwar, Intrusion Detection Systems in IoT: Techniques, Datasets, and Challenges, 1st ed., Computing Technologies and Applications, pp. 1-40, 2021.
[Google Scholar] [Publisher Link]
[4] Eduardo K. Viegas, Altair O. Santin, and Luiz S. Oliveira, “Toward A Reliable Anomaly-Based Intrusion Detection in Real-World Environments,” Computer Networks, vol. 127, pp. 200-216, 2017.
[CrossRef] [Google Scholar] [Publisher Link]
[5] Nour Moustafa, and Jill Slay, “A Hybrid Feature Selection for Network Intrusion Detection Systems: Central Points,” arXiv, pp. 5-13, 2015.
[CrossRef] [Google Scholar] [Publisher Link]
[6] Hossein Gharaee, and Hamid Hosseinvand, “A New Feature Selection IDS Based on Genetic Algorithm and SVM,” 8th International Symposium on Telecommunications, Tehran, Iran, pp. 139-144, 2016.
[CrossRef] [Google Scholar] [Publisher Link]
[7] Chowdhury Md. Nasimuzzaman, Ken Ferens, and Mike Ferens, “Network Intrusion Detection using Machine Learning,” Proceedings of the International Conference on Security and Management, pp. 30-35, 2016.
[Google Scholar] [Publisher Link]
[8] Deval Bhamare et al., “Feasibility of Supervised Machine Learning for Cloud Security,” International Conference on Information Science and Security, Pattaya, Thailand, pp. 1-5, 2016.
[CrossRef] [Google Scholar] [Publisher Link]
[9] Mirza M. Baig, Mian M. Awais, and El-Sayed M. El-Alfy, “A Multiclass Cascade of Artificial Neural Network for Network Intrusion Detection,” Journal of Intelligent & Fuzzy Systems, vol. 32, no. 4, pp. 2875-2883, 2017.
[CrossRef] [Google Scholar] [Publisher Link]
[10] Mustapha Belouch, Salah El Hadaj, and Mohamed Idhammad, “A Two-Stage Classifier Approach using RepTree Algorithm for Network Intrusion Detection,” International Journal of Advanced Computer Science and Applications, vol. 8, no. 6, pp. 389-394, 2017.
[CrossRef] [Google Scholar] [Publisher Link]
[11] Malek Al-Zewairi, Sufyan Almajali, and Arafat Awajan, “Experimental Evaluation of a Multi-Layer Feed-Forward Artificial Neural Network Classifier for Network Intrusion Detection System,” IEEE International Conference on New Trends in Computing Sciences, Amman, Jordan, pp. 167-172, 2017.
[CrossRef] [Google Scholar] [Publisher Link]
[12] Hebatallah Mostafa Anwer, Mohamed Farouk, and Ayman Abdel-Hami, “A Framework for Efficient Network Anomaly Intrusion Detection with Features Selection,” 9th International Conference on Information and Communication Systems, Irbid, Jordan, pp. 157-162, 2018.
[CrossRef] [Google Scholar] [Publisher Link]
[13] M.A. Mithun Aravind, and V.K.G. Kalaiselvi, “Design of an Intrusion Detection System Based on Distance Feature Using Ensemble Classifier,” 4th International Conference on Signal Processing, Communications and Networking, Chennai, India, pp. 1-6, 2017.
[CrossRef] [Google Scholar] [Publisher Link]
[14] Mohamed Idhammad, Karim Afdel, and Mustapha Belouch, “DoS Detection Method based on Artificial Neural Networks,” International Journal of Advanced Computer Science and Applications, vol. 8, no. 4, pp. 465-471, 2017.
[CrossRef] [Google Scholar] [Publisher Link]
[15] Vajiheh Hajisalem, and Shahram Babaie, “A Hybrid Intrusion Detection System Based on ABC-AFS Algorithm for Misuse and Anomaly Detection,” Computer Network, vol. 136, pp. 37-50, 2018.
[CrossRef] [Google Scholar] [Publisher Link]
[16] Sayantan Guha, Stephen S. Yau, and Arun Balaji Buduru, “Attack Detection in Cloud Infrastructures Using Artificial Neural Network with Genetic Feature Selection,” IEEE 14th Intl Conf on Dependable, Autonomic and Secure Computing, 14th International Conference on Pervasive Intelligence and Computing, 2nd International Conference on Big Data Intelligence and Computing and Cyber Science and Technology Congress, Auckland, New Zealand, pp. 414-419, 2016.
[CrossRef] [Google Scholar] [Publisher Link]
[17] Muhammad Hilmi Kamarudin et al., “A LogitBoost-Based Algorithm for Detecting Known and Unknown Web Attacks,” IEEE Access, vol. 5, pp. 26190-26200, 2017.
[CrossRef] [Google Scholar] [Publisher Link]
[18] Nour Moustafa, Jill Slay, and Gideon Creech, “Novel Geometric Area Analysis Technique for Anomaly Detection using Trapezoidal Area Estimation on Large-scale Networks,” IEEE Transactions on Big Data, vol. 5, no. 4, pp. 481-494, 2019.
[CrossRef] [Google Scholar] [Publisher Link]
[19] Khoi Khac Nguyen et al., “Cyberattack Detection in Mobile Cloud Computing: A Deep Learning Approach,” IEEE Wireless Communications and Networking Conference, Barcelona, Spain, pp. 1-6, 2018.
[CrossRef] [Google Scholar] [Publisher Link]
[20] Rifkie Primartha, and Bayu Adhi Tama, “Anomaly Detection using Random Forest: A Performance Revisited,” International Conference on Data and Software Engineering, Palembang, Indonesia, pp. 1-6, 2017.
[CrossRef] [Google Scholar] [Publisher Link]
[21] Sana Siddiqui, Muhammad Salman Khan, and Ken Ferens, “Multiscale Hebbian Neural Network for Cyber Threat Detection,” International Joint Conference on Neural Networks, Anchorage, AK, USA, pp. 1427-1434, 2017.
[CrossRef] [Google Scholar] [Publisher Link]
[22] K. Nahiyan et al., “A Multi-agent Based Cognitive Approach to Unsupervised Feature Extraction and Classification for Network Intrusion Detection,” International Conference on Advances on Applied Cognitive Computing, 2017.
[Google Scholar]
[23] Bipraneel Roy, and Hon Cheung, “A Deep Learning Approach for Intrusion Detection in Internet of Things using Bi-Directional Long Short- Term Memory Recurrent Neural Network,” 28th International Telecommunication Network and Applications Conference, Sydney, NSW, Australia, pp. 1-6, 2018.
[CrossRef] [Google Scholar] [Publisher Link]
[24] Nour Moustafa, Benjamin Turnbull, and Kim-Kwang Raymond Choo, “An Ensemble Intrusion Detection Technique based on proposed Statistical Flow Features for Protecting Network Traffic of Internet of Things,” IEEE Internet of Things Journal, vol. 6, no. 3, pp. 4815-4830, 2019.
[CrossRef] [Google Scholar] [Publisher Link]
[25] Bayu Adhi Tama, and Kyung-Hyune Rhee, “Attack Classification Analysis of IoT Network via Deep Learning Approach,” Research Briefs on Information & Communication Technology Evolution, vol. 3, pp. 150-158, 2017.
[CrossRef] [Google Scholar] [Publisher Link]
[26] Mustapha Belouch, Salah El Hadaj, and Mohamed Idhammad, “Performance Evaluation of Intrusion Detection Based on Machine Learning Using Apache Spark,” Procedia Computer Science, vol. 127, pp. 1-6, 2018.
[CrossRef] [Google Scholar] [Publisher Link]
[27] Yiyun Zhou et al., “Deep Learning Approach for Cyberattack Detection,” IEEE Conference on Computer Communications Workshops, Honolulu, HI, USA, pp. 262-267, 2018.
[CrossRef] [Google Scholar] [Publisher Link]
[28] Nour Moustafa et al., “Collaborative Anomaly Detection Framework for handling Big Data of Cloud Computing,” Military Communications and Information Systems Conference, Canberra, ACT, Australia, pp. 1-6, 2017.
[CrossRef] [Google Scholar] [Publisher Link]
[29] Muna AL-Hawawreh, Nour Moustafa, and Elena Sitnikova, “Identification of Malicious Activities in Industrial Internet of Things Based on Deep Learning Models,” Journal of Information Security and Application, vol. 41, pp. 1-11, 2018.
[CrossRef] [Google Scholar] [Publisher Link]
[30] Nour Moustafa et al., “A New Threat Intelligence Scheme for Safeguarding Industry 4.0 Systems,” IEEE Access, vol. 6, pp. 32910-32924, 2018.
[CrossRef] [Google Scholar] [Publisher Link]
[31] Valentina Timčenko, and Slavko Gajin, “Ensemble Classifiers for Supervised Anomaly Based Network Intrusion Detection,” 13th IEEE International Conference on Intelligent Computer Communication and Processing, Cluj-Napoca, Romania, pp. 13-19, 2017.
[CrossRef] [Google Scholar] [Publisher Link]
[32] Nour Moustafa, Gaurav Misra, and Jill Slay, “Generalized Outlier Gaussian Mixture technique based on Automated Association Features for Simulating and Detecting Web Application Attacks,” IEEE Transactions on Sustainable Computing, vol. 6, no. 2, pp. 245-256, 2018.
[CrossRef] [Google Scholar] [Publisher Link]
[33] Tian Yingjie et al., “Ramp Loss One-Class Support Vector Machine; A Robust and Effective Approach To Anomaly Detection Problems,” Journal Neorocomputing, vol. 310, pp. 223-235, 2018.
[CrossRef] [Google Scholar] [Publisher Link]
[34] Mukrimah Nawir et al., “Performances of Machine Learning Algorithms for Binary Classification of Network Anomaly Detection System,” 1st International Conference on Big Data and Cloud Computing, pp. 1-8, 2017.
[CrossRef] [Google Scholar] [Publisher Link]
[35] Hung Nguyen Viet et al., “Using Deep Learning Model for Network Scanning Detection,” Proceedings of the 4th International Conference on Frontiers of Educational Technologies, pp. 117-121, 2018.
[CrossRef] [Google Scholar] [Publisher Link]
[36] Souhail Meftah, Tajjeeddine Rachidi, and Nasser Assem, “Network Based Intrusion Detection Using the UNSW-NB15 Dataset,” International Journal of Computing and Digital Systems, vol. 8, no. 5, pp. 477-487, 2019.
[CrossRef] [Google Scholar]
[37] Ramy Elhefnawy, Hassan Abounaser, and Amr Badr, “A Hybrid Nested Genetic-Fuzzy Algorithm Framework for Intrusion Detection and Attacks,” IEEE Access, vol. 8, pp. 98218-98233, 2020.
[CrossRef] [Google Scholar] [Publisher Link]
[38] Abhishek Verma, and Virender Ranga, “Evaluation of Network Intrusion Detection Systems for RPL Based 6LoWPAN Networks in IoT,” Wireless Personal Communications, vol. 108, pp. 1571-1594, 2019.
[CrossRef] [Google Scholar] [Publisher Link]
[39] Abhishek Verma, and Virender Ranga, “ELNIDS: Ensemble Learning based Network Intrusion Detection System for RPL based Internet of Things,” 4th International Conference on Internet of Things: Smart Innovation and Usages, Ghaziabad, India, pp. 1-6, 2019.
[CrossRef] [Google Scholar] [Publisher Link]
[40] P. Jaya Prakash, and B. Lalitha, “A Novel Intrusion Detection System for RPL Based IoT Networks with Bio-Inspired Feature Selection and Ensemble Classifier,” Research Square, pp. 1-23, 2021.
[CrossRef] [Google Scholar] [Publisher Link]
[41] George Simoglou et al., “Intrusion Detection Systems for RPL Security: A Comparative Analysis,” Computers & Security, vol. 104, 2021.
[CrossRef] [Google Scholar] [Publisher Link]
[42] Musa Osman et al., “Artificial Neural Network Model for Decreased Rank Attack Detection in RPL Based on IoT Networks,” International Journal of Network Security, vol. 23, no. 3, pp. 496-503, 2021.
[CrossRef] [Google Scholar] [Publisher Link]
[43] Abhishek Verma, and Virender Ranga, “Mitigation of DIS Flooding Attacks in RPL-based 6LoWPAN Networks,” Emerging Telecommunications Technologies, vol. 31, no. 2, 2020.
[CrossRef] [Google Scholar] [Publisher Link]
[44] Sarumathi Murali, and Abbas Jamalipour, “A Lightweight Intrusion Detection for Sybil Attack under Mobile RPL in the Internet of Things,” IEEE Internet of Things Journal, vol. 7, no. 1, pp. 379-388, 2020.
[CrossRef] [Google Scholar] [Publisher Link]
[45] John Foley, Naghmeh Moradpoor, and Henry Ochenyi “Employing a Machine Learning Approach to Detect Combined Internet of Things Attacks against Two Objective Functions Using a Novel Dataset,” Security and Communication Networks, vol. 2020, no. 1, pp. 1-17, 2020.
[CrossRef] [Google Scholar] [Publisher Link]
[46] Abhishek Verma, and Virender Ranga, “Machine Learning Based Intrusion Detection Systems for IoT Applications,” Wireless Personal Communications, vol. 111, pp. 2287-2310, 2020.
[CrossRef] [Google Scholar] [Publisher Link]
[47] Mohammed Al Qurashi, Constantinos Marios Angelopoulos, and Vasilios Katos, “An Architecture for Resilient Intrusion Detection in IoT Networks,” ICC 2020 - 2020 IEEE International Conference on Communications, Dublin, Ireland, pp. 1-7, 2020.
[CrossRef] [Google Scholar] [Publisher Link]
[48] Gaurav Soni, and R. Sudhakar, “A L-IDS against Dropping Attack to Secure and Improve RPL Performance in WSN Aided IoT,” 7th International Conference on Signal Processing and Integrated Networks, Noida, India, pp. 377-383, 2020.
[CrossRef] [Google Scholar] [Publisher Link]
[49] Arun Kumar Bediya, and Rajendra Kumar, “Real Time DDoS Intrusion Detection and Monitoring Framework in 6LoWPAN for Internet of Things,” IEEE International Conference on Computing, Power and Communication Technologies, Greater Noida, India, pp. 824-828, 2020.
[CrossRef] [Google Scholar] [Publisher Link]
[50] K.N. Ambili, and Jimmy Jose, “TN-IDS for Network Layer Attacks in RPL based IoT Systems,” IACR Cryptology ePrint Archive, pp. 1-24, 2020.
[Google Scholar] [Publisher Link]
[51] Himanshu B. Patel, and Devesh C. Jinwala, “Blackhole Detection in 6LoWPAN Based Internet of Things: An Anomaly Based Approach,” TENCON 2019 - 2019 IEEE Region 10 Conference, Kochi, India, pp. 947-954, 2019.
[CrossRef] [Google Scholar] [Publisher Link]
[52] Sarika Choudhary, and Nishtha Kesswani, “Cluster-Based Intrusion Detection Method for Internet of Things,” IEEE/ACS 16th International Conference on Computer Systems and Applications, Abu Dhabi, United Arab Emirates, pp. 1-8, 2019.
[CrossRef] [Google Scholar] [Publisher Link]
[53] Emre Aydogan et al., “A Central Intrusion Detection System for RPL-Based Industrial Internet of Things,” 15th IEEE International Workshop on Factory Communication Systems, Sundsvall, Sweden, pp. 1-5, 2019.
[CrossRef] [Google Scholar] [Publisher Link]
[54] Ahmed Raoof, Ashraf Matrawy, and Chung-Horng Lung, “Routing Attacks and Mitigation Methods for RPL-Based Internet of Things,” IEEE Communications Surveys & Tutorials, vol. 21, no. 2, pp. 1582-1606, 2019.
[CrossRef] [Google Scholar] [Publisher Link]
[55] Behnam Farzaneh, Mohammad Ali Montazeri, and Shahram Jamali, “An Anomaly-Based IDS for Detecting Attacks in RPL-Based Internet of Things,” 5th International Conference on Web Research, Tehran, Iran, pp. 61-66, 2019.
[CrossRef] [Google Scholar] [Publisher Link]
[56] Snehal Deshmukh-Bhosale, and Santosh S. Sonavane, “A Real-Time Intrusion Detection System for Wormhole Attack in the RPL based Internet of Things,” Procedia Manufacturing, vol. 32, pp. 840-847, 2019.
[CrossRef] [Google Scholar] [Publisher Link]
[57] Mohamad Nazrin Napiah et al., “Compression Header Analyzer Intrusion Detection System (CHA - IDS) for 6LoWPAN Communication Protocol,” IEEE Access, vol. 6, pp. 16623-16638, 2018.
[CrossRef] [Google Scholar] [Publisher Link]
[58] Ashwini Nikam, and Dayan Ambawade, “Opinion Metric Based Intrusion Detection Mechanism for RPL Protocol in IoT,” 3rd International Conference for Convergence in Technology, Pune, India, pp. 1-6, 2018.
[CrossRef] [Google Scholar] [Publisher Link]
[59] Elie Kfoury et al., “A Self Organizing Map Intrusion Detection System for RPL Protocol Attacks,” International Journal of Interdisciplinary Telecommunications and Networking, vol. 11, no. 1, pp. 30-43, 2019.
[CrossRef] [Google Scholar] [Publisher Link]
[60] Faiza Medjek et al., “A Trust-Based Intrusion Detection System for Mobile RPL Based Networks,” IEEE International Conference on Internet of Things (iThings) and IEEE Green Computing and Communications (GreenCom) and IEEE Cyber, Physical and Social Computing (CPSCom) and IEEE Smart Data (SmartData), Exeter, UK, pp. 735-742, 2017.
[CrossRef] [Google Scholar] [Publisher Link]
[61] Minalini Goyal, and Maitreyee Dutta, “Intrusion Detection of Wormhole Attack in IoT: A Review,” International Conference on Circuits and Systems in Digital Enterprise Technology, Kottayam, India, pp. 1-5, 2018.
[CrossRef] [Google Scholar] [Publisher Link]
[62] Furkan Yusuf Yavuz, Devrim Ünal, and Ensar Gül, “Deep Learning for Detection of Routing Attacks in the Internet of Things,” International Journal of Computational Intelligence Systems, vol. 12, pp. 39-58, 2018.
[CrossRef] [Google Scholar] [Publisher Link]
[63] R. Darwin, “Implementation of Advanced IDS in Contiki for Highly Secured Wireless Sensor Network,” International Journal of Applied Engineering Research, vol. 13, no. 6, pp. 4214-4218, 2018.
[Google Scholar] [Publisher Link]
[64] Fatma Gara et al., “An Intrusion Detection System for Selective Forwarding Attack in IPv6-based Mobile WSNs,” 13th International Wireless Communications and Mobile Computing Conference, Valencia, Spain, pp. 276-281, 2017.
[CrossRef] [Google Scholar] [Publisher Link]
[65] Anhtuan Le et al., “A Specification-Based IDS for Detecting Attacks on RPL-Based Network Topology,” Information, vol. 7, no. 2, pp. 1-19, 2016.
[CrossRef] [Google Scholar] [Publisher Link]
[66] Ting Miao, Ramnath Teja Chekka, and Ki-Hyung Kim, “GIDPS: A Game Theory-Based IDPS for RPL-Networked Low Power Lossy Networks with Energy Limitation,” Sixth International Conference on Ubiquitous and Future Networks, Shanghai, China, pp. 278-283, 2014.
[CrossRef] [Google Scholar] [Publisher Link]
[67] Anhtuan Le et al., “Specification-Based IDS for Securing RPL from Topology Attacks,” IFIP Wireless Days, Niagara Falls, ON, Canada, pp. 1-3, 2011.
[CrossRef] [Google Scholar] [Publisher Link]
[68] Erdem Canbalaban, and Sevil Sen, “A Cross-Layer Intrusion Detection System for RPL-Based Internet of Things,” Ad-Hoc, Mobile, and Wireless Networks, pp. 214-227, 2020.
[CrossRef] [Google Scholar] [Publisher Link]
[69] Behnam Farzaneh et al., “A New Method for Intrusion Detection on RPL Routing Protocol Using Fuzzy Logic,” 6th International Conference on Web Research, Tehran, Iran, pp. 245-250, 2020.
[CrossRef] [Google Scholar] [Publisher Link]
[70] Alexander Vodyaho et al., “Data Collection Technology for Ambient Intelligence Systems in Internet of Things,” Electronics, vol. 9, no. 11, pp. 1-26, 2020.
[CrossRef] [Google Scholar] [Publisher Link]
[71] Matija Stevanovic, and Jens Myrup Pedersen, “On the Use of Machine Learning for Identifying Botnet Network Traffic,” Journal of Cyber Security and Mobility, vol. 4, pp. 1-32, 2015.
[CrossRef] [Google Scholar] [Publisher Link]
[72] Ahmad Azab, Mamoun Alazab, and Mahdi Aiash, “Machine Learning Based Botnet Identification Traffic,” IEEE Trustcom/BigDataSE/ISPA, Tianjin, China, pp. 1788-1794, 2016.
[CrossRef] [Google Scholar] [Publisher Link]
[73] Sean Miller, and Curtis Busby-Earle, “The Role of Machine Learning In Botnet Detection,” 11th International Conference for Internet Technology and Secured Transactions, Barcelona, Spain, pp. 359-364, 2016.
[CrossRef] [Google Scholar] [Publisher Link]
[74] Qianru Zhou et al., “An Assessment of Intrusion Detection using Machine Learning on Traffic Statistical Data,” TechRxiv, vol. 14, no. 8, pp. 1-10, 2015.
[CrossRef] [Google Scholar] [Publisher Link]
[75] K.V. Pradeepthi, and Arputharaj Kannan, “Detection of Botnet traffic by using Neuro-fuzzy based Intrusion Detection,” Tenth International Conference on Advanced Computing, Chennai, India, pp. 118-123, 2018.
[CrossRef] [Google Scholar] [Publisher Link]
[76] Yair Meidan et al., “N-BaIoT—Network-Based Detection of IoT Botnet Attacks Using Deep Autoencoders,” IEEE Pervasive Computing, vol. 17, no. 3, pp. 12-22, 2018.
[CrossRef] [Google Scholar] [Publisher Link]
[77] Jadel Alsamiri, and Khalid Alsubhi, “Internet of Things Cyber Attacks Detection using Machine Learning,” International Journal of Advanced Computer Science and Applications, vol. 10, no. 12, pp. 627-634, 2019.
[CrossRef] [Google Scholar] [Publisher Link]
[78] Nour Moustafa, Benjamin Turnbull, and Kim-Kwang Raymond Choo, “An Ensemble Intrusion Detection Technique Based on Proposed Statistical Flow Features for Protecting Network Traffic of Internet of Things,” IEEE Internet of Things Journal, vol. 6, no. 3, pp. 4815-4830, 2019.
[CrossRef] [Google Scholar] [Publisher Link]
[79] Duc C. Le, and Nur Zincir-Heywood, “Learning from Evolving Network Data for Dependable Botnet Detection,” 15th International Conference on Network and Service Management, Halifax, NS, Canada, pp. 1-5, 2019.
[CrossRef] [Google Scholar] [Publisher Link]
[80] Reem Alhajri, and Rachid Zagrouba, and Fahd Al-Haidari, “Survey for Anomaly Detection of IoT Botnets Using Machine Learning Auto-Encoders,” International Journal of Applied Engineering Research, vol. 14, no. 10, pp. 2417-2421, 2019.
[Google Scholar] [Publisher Link]
[81] Zainab Al-Othman, Mouhammd Alkasassbeh, and Sherenaz AL-Haj Baddar, “A State-of-the-Art Review on IoT Botnet Attack Detection,” arXiv, pp. 1-6, 2020.
[CrossRef] [Google Scholar] [Publisher Link]
[82] V. Kanimozhi, and Thangavel Prem Jacob, “Artificial Intelligence Outflanks All Other Machine Learning Classifiers in Network Intrusion Detection System on the Realistic Cyber Dataset CSE-CIC-IDS2018 Using Cloud Computing,” ICT Express, vol. 7, no. 3, pp. 366-370, 2021.
[CrossRef] [Google Scholar] [Publisher Link]
[83] Mustafa Alshamkhany et al., “Botnet Attack Detection using Machine Learning,” 14th International Conference on Innovations in Information Technology(IIT), Al Ain, United Arab Emirates, pp. 203-208, 2020.
[CrossRef] [Google Scholar] [Publisher Link]
[84] A. Sankaran et al., “Botnet Detection Using Machine Learning,” International Research Journal of Engineering and Technology, vol. 7, no. 7, pp. 5116-5121, 2020.
[Google Scholar] [Publisher Link]
[85] MohammadNoor Injadat, Abdallah Moubayed, and Abdallah Shami, “Detecting Botnet Attacks in IoT Environments: An Optimized Machine Learning Approach,” 32nd International Conference on Microelectronics, Aqaba, Jordan, pp. 1-4, 2020.
[CrossRef] [Google Scholar] [Publisher Link]
[86] Aaya F. Jabbar, and Imad J. Mohammed, “Development of an Optimized Botnet Detection Framework based on Filters of Features and Machine Learning Classifiers using CICIDS2017 Dataset,” IOP Conference Series: Materials Science and Engineering, vol. 928, pp. 1-13, 2020.
[CrossRef] [Google Scholar] [Publisher Link]
[87] Shreehar Joshi, and Eman Abdelfattah, “Efficiency of Different Machine Learning Algorithms on the Multivariate Classification of IoT Botnet Attacks,” 11th IEEE Annual Ubiquitous Computing, Electronics & Mobile Communication Conference, New York, NY, USA, pp. 517-521, 2020.
[CrossRef] [Google Scholar] [Publisher Link]
[88] Jiyeon Kim et al., “Feature Analysis of IoT Botnet Attacks based on RNN and LSTM,” International Journal of Engineering Trends and Technology, vol. 68, no. 4, pp. 43-47, 2020.
[CrossRef] [Google Scholar] [Publisher Link]
[89] Jiyeon Kim et al., “Intelligent Detection of IoT Botnets Using Machine Learning and Deep Learning,” Applied Sciences, vol. 10, no. 19, pp. 1-22, 2020.
[CrossRef] [Google Scholar] [Publisher Link]
[90] Madhuri Gurunathrao Desai, Yong Shi, and Kun Suo, “IoT Bonet and Network Intrusion Detection using Dimensionality Reduction and Supervised Machine Learning,” 11th IEEE Annual Ubiquitous Computing, Electronics & Mobile Communication Conference, New York, NY, USA, 2020.
[CrossRef] [Google Scholar] [Publisher Link]
[91] Mnahi Alqahtani, Hassan Mathkour, and Mohamed Maher Ben Ismail, “IoT Botnet Attack Detection Based on Optimized Extreme Gradient Boosting and Feature Selection,” Sensors, vol. 20, no. 21, pp. 1-21, 2020.
[CrossRef] [Google Scholar] [Publisher Link]
[92] Alejandro Guerra-Manzanares et al., “MedBIoT: Generation of an IoT Botnet Dataset in a Medium-sized IoT Network,” Proceedings of the 6th International Conference on Information Systems Security and Privacy, Valletta, Malta, vol. 1, pp. 207-218, 2020.
[CrossRef] [Google Scholar] [Publisher Link]
[93] Christian Dietz et al., “Towards Adversarial Resilience in Proactive Detection of Botnet Domain Names by using MTD,” NOMS 2020 - 2020 IEEE/IFIP Network Operations and Management Symposium, Budapest, Hungary, pp. 1-5, 2020.
[CrossRef] [Google Scholar] [Publisher Link]
[94] Hasan Alkahtani, and Theyazn H.H. Aldhyani, “Botnet Attack Detection by Using CNN-LSTM Model for Internet of Things Applications,” Security and Communication Networks, vol. 2021, no. 1, pp. 1-23, 2021.
[CrossRef] [Google Scholar] [Publisher Link]
[95] Rana Faek et al., “Exposing Bot Attacks Using Machine Learning and Flow Level Analysis,” International Conference on Data Science, E-learning and Information Systems, pp. 99-106, 2021.
[CrossRef] [Google Scholar] [Publisher Link]
[96] Amritanshu Pandey et al., “Identification of Botnet Attacks Using Hybrid Machine Learning Models,” Hybrid Intelligent Systems, pp. 249-257, 2019.
[CrossRef] [Google Scholar] [Publisher Link]
[97] P. Jithu et al., “Intrusion Detection System for IoT Botnet Attacks Using Deep Learning,” SN Computer Science, vol. 2, 2021.
[CrossRef] [Google Scholar] [Publisher Link]
[98] Sikha Bagui, Xiaojian Wang, and Subhash Bagui, “Machine Learning Based Intrusion Detection for IoT Botnet,” International Journal of Machine Learning and Computing, vol. 11, no. 6, pp. 399-406, 2021.
[CrossRef] [Google Scholar] [Publisher Link]
[99] Khlood Shinan et al., “Machine Learning-Based Botnet Detection in Software-Defined Network: A Systematic Review,” Symmetry, vol. 13, no. 5, pp. 1-28, 2021.
[CrossRef] [Google Scholar] [Publisher Link]
[100] Han Wang et al., “Non-IID Data Re-Balancing at IoT Edge with Peer-To-Peer Federated Learning for Anomaly Detection,” Proceedings of the 14th ACM Conference on Security and Privacy in Wireless and Mobile Networks, pp. 153-163, 2021. [CrossRef] [Google Scholar] [Publisher Link]
[101] Segun I. Popoola et al., “SMOTE-DRNN: A Deep Learning Algorithm for Botnet Detection in the Internet-of-Things Networks,” Sensors, vol. 21, no. 9, pp. 1-20, 2021.
[CrossRef] [Google Scholar] [Publisher Link]
[102] Erman Özer, Murat İskefiyeli, and Jahongir Azimjonov, “Toward Lightweight Intrusion Detection Systems Using the Optimal and Efficient Feature Pairs of the Bot-IoT 2018 Dataset,” International Journal of Distributed Sensor Networks, vol. 17, no. 10, 2021.
[CrossRef] [Google Scholar] [Publisher Link]