Efficient Traffic Routing with Temporal Fusion Transformers: Addressing Urban Congestion Challenges
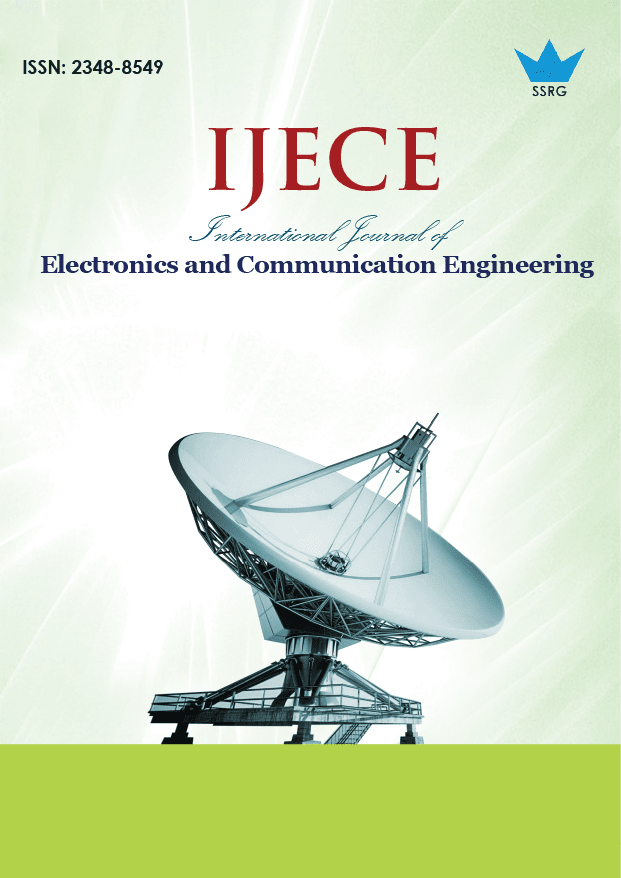
International Journal of Electronics and Communication Engineering |
© 2024 by SSRG - IJECE Journal |
Volume 11 Issue 11 |
Year of Publication : 2024 |
Authors : Sreelekha M, Midhunchakkaravarthy Janarthanan |
How to Cite?
Sreelekha M, Midhunchakkaravarthy Janarthanan, "Efficient Traffic Routing with Temporal Fusion Transformers: Addressing Urban Congestion Challenges," SSRG International Journal of Electronics and Communication Engineering, vol. 11, no. 11, pp. 196-212, 2024. Crossref, https://doi.org/10.14445/23488549/IJECE-V11I11P117
Abstract:
The exponential increase in urban population necessitates the emergence of transportation systems that are both effective and sustainable, using the potential modern technology. The issue of dynamic traffic flow significantly impedes the movement of vehicles. Traffic congestion is a critical issue affecting urban mobility and efficiency in cities worldwide, with Bangalore no exception. This study addresses the challenge of leveraging advanced predictive analytics and intelligent transport systems to manage traffic congestion. The proposed research aims to address the limitations of traditional traffic management strategies by integrating the Temporal Fusion Transformer (TFT) model into an Intelligent Transport System (ITS) framework. The research employs rigorous data preprocessing techniques to leverage extensive data from multiple online map service providers and traffic monitoring platforms, spanning from January 1, 2019, to December 31, 2023. The TFT model forecasts traffic congestion with notable precision, achieving a Mean Absolute Error (MAE) of 0.39, Mean Squared Error (MSE) of 0.30, Root Mean Squared Error (RMSE) of 0.55, Mean Absolute Percentage Error (MAPE) of 7.2%, and an R-squared (R²) value of 0.87. The outcomes obtained clearly illustrate the model’s superior accuracy and efficacy. Integrating TFT predictions into the ITS framework enhances real-time traffic control by improving the timings of traffic signals, recommending alternative routes, and improving incident management. This proactive approach significantly reduces traffic congestion and enhances travel efficiency, substantially advancing urban traffic management solutions.
Keywords:
Temporal Fusion Transformer, Intelligent Transport System, Traffic congestion, Traffic volume, Smart city.
References:
[1] Vito Albino, Umberto Berardi, and Rosa Maria Dangelico, “Smart Cities: Definitions, Dimensions, Performance, and Initiatives,” Journal of Urban Technology, vol. 22, no. 1, pp. 3-21, 2015.
[CrossRef] [Google Scholar] [Publisher Link]
[2] Roopa Ravish, and Shanta Ranga Swamy, “Intelligent Traffic Management: A Review of Challenges, Solutions, and Future Perspectives,” Transport and Telecommunication Journal, vol. 22, no. 2, pp. 163-182, 2021.
[CrossRef] [Google Scholar] [Publisher Link]
[3] Wesley E. Marshall, and Eric Dumbaugh, “Revisiting the Relationship between Traffic Congestion and the Economy: A Longitudinal Examination of US Metropolitan Areas,” Transportation, vol. 47, pp. 275-314, 2020.
[CrossRef] [Google Scholar] [Publisher Link]
[4] Ghosh Banishree, Intelligent Mobility for Minimizing the Impact of Traffic Incidents on Transportation Networks, 1st ed., Augmented Intelligence Toward Smart Vehicular Applications, CRC Press, pp. 175-194, 2020.
[Google Scholar] [Publisher Link]
[5] Guofa Li et al., “Influence of Traffic Congestion on Driver Behavior in Post-Congestion Driving,” Accident Analysis & Prevention, vol. 141, 2020.
[CrossRef] [Google Scholar] [Publisher Link]
[6] Elias C. Eze et al., “Advances in Vehicular Ad-Hoc Networks (VANETs): Challenges and Road-Map for Future Development,” International Journal of Automation and Computing, vol. 13, pp. 1-18, 2016.
[CrossRef] [Google Scholar] [Publisher Link]
[7] K. Ramesh, A. Lakshna, and P.N. Renjith, “Smart Traffic Congestion Model in IoT - A Review,” 2020 4th International Conference on Electronics, Communication and Aerospace Technology (ICECA), Coimbatore, India, pp. 651-658, 2020. [CrossRef] [Google Scholar] [Publisher Link]
[8] J. Prakash et al., “A Vehicular Network Based Intelligent Transport System for Smart Cities Using Machine Learning Algorithms,” Scientific Reports, vol. 14, no. 1, pp. 1-16, 2024.
[CrossRef] [Google Scholar] [Publisher Link]
[9] Abdullahi Chowdhury et al., “IoT - Based Emergency Vehicle Services in Intelligent Transportation System,” Sensors, vol. 23, no. 11, pp. 1-17, 2023.
[CrossRef] [Google Scholar] [Publisher Link]
[10] Sura Mahmood Abdullah et al., “Optimizing Traffic Flow in Smart Cities: Soft GRU-Based Recurrent Neural Networks for Enhanced Congestion Prediction Using Deep Learning,” Sustainability, vol. 15, no. 7, pp. 1-21, 2023.
[CrossRef] [Google Scholar] [Publisher Link]
[11] Muhammad Saleem et al., “Smart Cities: Fusion-Based Intelligent Traffic Congestion Control System for Vehicular Networks Using Machine Learning Techniques,” Egyptian Informatics Journal, vol. 23, no. 3, pp. 417-426, 2022.
[CrossRef] [Google Scholar] [Publisher Link]
[12] Sharmila Majumdar et al., “Congestion Prediction for Smart Sustainable Cities Using IoT and Machine Learning Approaches,” Sustainable Cities and Society, vol. 64, 2021.
[CrossRef] [Google Scholar] [Publisher Link]
[13] G. Kothai et al., “A New Hybrid Deep Learning Algorithm for Prediction of Wide Traffic Congestion in Smart Cities,” Wireless Communications and Mobile Computing, vol. 2021, no. 1, pp. 1-13, 2021.
[CrossRef] [Google Scholar] [Publisher Link]
[14] Ayesha Atta et al., “An Adaptive Approach: Smart Traffic Congestion Control System,” Journal of King Saud University-Computer and Information Sciences, vol. 32, no. 9, pp. 1012-1019, 2020.
[CrossRef] [Google Scholar] [Publisher Link]
[15] A. Ata et al., “Modelling Smart Road Traffic Congestion Control System Using Machine Learning Techniques,” Neural Network World, vol. 29, no. 2, pp. 99-110, 2019.
[CrossRef] [Google Scholar] [Publisher Link]
[16] S. Muthuramalingam et al., “IoT Based Intelligent Transportation System (Iot-ITS) for Global Perspective: A Case Study,” Internet of Things and Big Data Analytics for Smart Generation, pp. 279-300, 2019.
[CrossRef] [Google Scholar] [Publisher Link]
[17] Sen Zhang et al., “Deep Autoencoder Neural Networks for Short-Term Traffic Congestion Prediction of Transportation Networks,” Sensors, vol. 19, no. 10, pp. 1-19, 2019.
[CrossRef] [Google Scholar] [Publisher Link]
[18] Derya Soydaner, “Attention Mechanism in Neural Networks: Where It Comes and Where It Goes,” Neural Computing and Applications, vol. 34, no. 16, pp. 13371-13385, 2022.
[CrossRef] [Google Scholar] [Publisher Link]
[19] Sepp Hochreiter, and Jürgen Schmidhuber, “Long Short-Term Memory,” Neural Computation, vol. 9, no. 8, pp. 1735-1780, 1997.
[CrossRef] [Google Scholar] [Publisher Link]
[20] Bryan Lim et al., “Temporal Fusion Transformers for Interpretable Multi-Horizon Time Series Forecasting,” International Journal of Forecasting, vol. 37, no. 4, pp. 1748-1764, 2021.
[CrossRef] [Google Scholar] [Publisher Link]
[21] Yann N. Dauphin et al., “Language Modeling with Gated Convolutional Networks,” Proceedings of the 34th International Conference on Machine Learning, Sydney NSW Australia, vol. 70, pp. 933-941, 2017.
[Google Scholar] [Publisher Link]
[22] Jimmy Lei Ba, Jamie Ryan Kiros, and Geoffrey E. Hinton, “Layer Normalization,” Arxiv, pp. 1-14, 2016.
[CrossRef] [Google Scholar] [Publisher Link]
[23] Djork-Arné Clevert, Thomas Unterthiner, and Sepp Hochreiter, “Fast and Accurate Deep Network Learning by Exponential Linear Units (ELUs),” Arxiv, pp. 1-14, 2015.
[CrossRef] [Google Scholar] [Publisher Link]
[24] Ashish Vaswani et al., “Attention is All You Need,” Proceedings of the 31st International Conference on Neural Information Processing Systems, Long Beach California USA, pp. 6000-6010, 2017.
[Google Scholar] [Publisher Link]