Optimizing Breast Cancer Recurrence Forecasting Using ANOVA Feature Selection and GRU Models
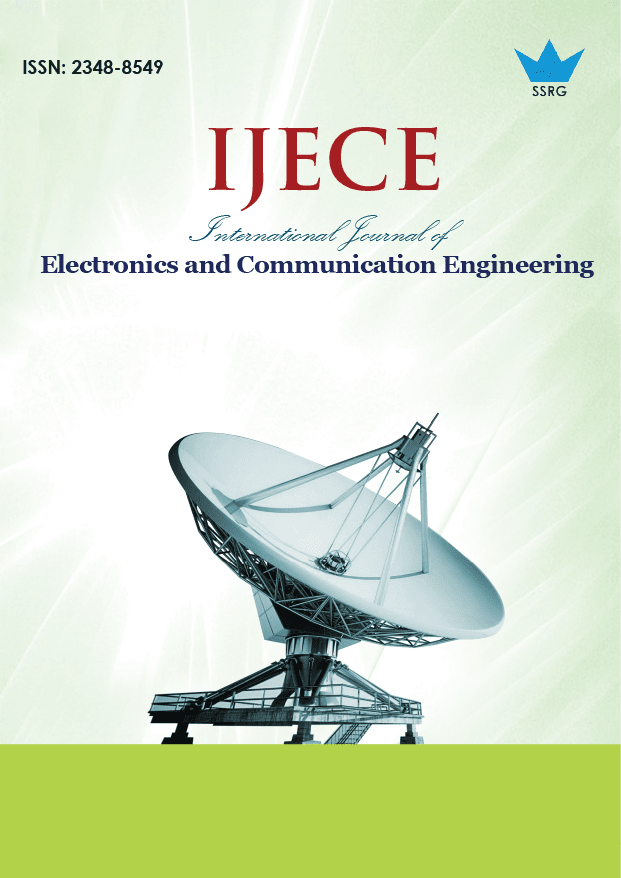
International Journal of Electronics and Communication Engineering |
© 2024 by SSRG - IJECE Journal |
Volume 11 Issue 11 |
Year of Publication : 2024 |
Authors : Arathi Chandran R, V. Mary Amala Bai |
How to Cite?
Arathi Chandran R, V. Mary Amala Bai, "Optimizing Breast Cancer Recurrence Forecasting Using ANOVA Feature Selection and GRU Models," SSRG International Journal of Electronics and Communication Engineering, vol. 11, no. 11, pp. 213-227, 2024. Crossref, https://doi.org/10.14445/23488549/IJECE-V11I11P118
Abstract:
The challenge of breast cancer recurrence remains a critical concern, prompting the need for effective predictive models that improve patient outcomes. This study introduces a novel prediction model, addressing common issues like complex model structures, high-dimensional data, and class imbalance. The model combines a Gated Recurrent Unit (GRU) with Analysis of Variance (ANOVA)-based feature selection to boost accuracy and reliability. Using the Wisconsin Breast Cancer (WBC) dataset, the study applies preprocessing techniques to enhance data quality. ANOVA is employed to select relevant features, which are input into the GRU model. The GRU’s multi-layer architecture successfully identifies complex patterns in the data. The model achieves impressive results, with a mean accuracy of 96.49%, precision of 97.04%, recall of 96.67%, and an F1-score of 96.67%. The confusion matrix and ROC curve analyses also validate the model’s performance in predicting recurrence. This GRU-ANOVA approach is promising to improve breast cancer recurrence predictions, offering critical insights for clinical decision-making and patient care.
Keywords:
Breast cancer recurrence prediction, Gated Recurrent Unit, Analysis of Variance (ANOVA), Feature optimization, Wisconsin Breast Cancer (WBC) dataset .
References:
[1] O. Mohammad Mehdi Owrang, Ginger Schwarz, and Fariba Jafari Horestani, “Prediction of Breast Cancer Recurrence with Machine Learning,” Encyclopedia of Information Science and Technology, Sixth Edition, pp. 1-33, 2024.
[CrossRef] [Google Scholar] [Publisher Link]
[2] Amal Alzu’bi et al., “Predicting the Recurrence of Breast Cancer Using Machine Learning Algorithms,” Multimedia Tools and Applications, vol. 80, no. 9, pp. 13787-13800, 2021.
[CrossRef] [Google Scholar] [Publisher Link]
[3] Shi-Jer Lou et al., “Machine Learning Algorithms to Predict Recurrence within 10 Years after Breast Cancer Surgery: A Prospective Cohort Study,” Cancers, vol. 12, no. 12, pp. 1-16, 2020.
[CrossRef] [Google Scholar] [Publisher Link]
[4] Noreen Fatima et al., “Prediction of Breast Cancer, Comparative Review of Machine Learning Techniques, and their Analysis,” IEEE Access, vol. 8, pp. 150360-150376, 2020.
[CrossRef] [Google Scholar] [Publisher Link]
[5] Björn Stenkvist et al., “Predicting Breast Cancer Recurrence,” Cancer, vol. 50, no. 12, pp. 2884-2893, 1982.
[CrossRef] [Google Scholar] [Publisher Link]
[6] Donald Courtney et al., “Breast Cancer Recurrence: Factors Impacting Occurrence and Survival,” Irish Journal of Medical Science, vol. 191, pp. 2501-2510, 2022.
[CrossRef] [Google Scholar] [Publisher Link]
[7] Mallika Siva Donepudi et al., “Breast Cancer Statistics and Markers,” Journal of Cancer Research and Therapeutics, vol. 10, no. 3, pp. 506-511, 2014.
[CrossRef] [Google Scholar] [Publisher Link]
[8] Mahmoud Hussein, Mohammed Elnahas, and Arabi Keshk, “A Framework for Predicting Breast Cancer Recurrence,” Expert Systems with Applications, vol. 240, 2024.
[CrossRef] [Google Scholar] [Publisher Link]
[9] Ying Liu et al., “Clinical Decision Support Tool for Breast Cancer Recurrence Prediction Using SHAP Value in Cooperative Game Theory,” Heliyon, vol. 10, no. 2, pp. 1-11, 2024.
[CrossRef] [Google Scholar] [Publisher Link]
[10] Lorena González-Castro et al., “Machine Learning Algorithms to Predict Breast Cancer Recurrence Using Structured and Unstructured Sources from Electronic Health Records,” Cancers, vol. 15, no. 10, pp. 1-16, 2023.
[CrossRef] [Google Scholar] [Publisher Link]
[11] Frederick M. Howard et al., “Integration of Clinical Features and Deep Learning on Pathology for the Prediction of Breast Cancer Recurrence Assays and Risk of Recurrence,” NPJ Breast Cancer, vol. 9, no. 1, pp. 1-6, 2023.
[CrossRef] [Google Scholar] [Publisher Link]
[12] Lixuan Zeng et al., “The Innovative Model Based on Artificial Intelligence Algorithms to Predict Recurrence Risk of Patients with Postoperative Breast Cancer,” Frontiers in Oncology, vol. 13, pp. 1-12, 2023.
[CrossRef] [Google Scholar] [Publisher Link]
[13] Nermin Abdelhakim Othman, Manal A. Abdel-Fattah, and Ahlam Talaat Ali, “A Hybrid Deep Learning Framework with Decision-Level Fusion for Breast Cancer Survival Prediction,” Big Data and Cognitive Computing, vol. 7, no. 1, pp. 1-16, 2023.
[CrossRef] [Google Scholar] [Publisher Link]
[14] Lulu Wang, “Microwave Imaging and Sensing Techniques for Breast Cancer Detection,” Micromachines, vol. 14, no. 7, pp. 1-29, 2023.
[CrossRef] [Google Scholar] [Publisher Link]
[15] G. Rajasekaran, and C. Sunitha Ram, “Breast Cancer Prediction Based on Feature Extraction Using Hybrid Methodologies,” International Journal of Soft Computing and Engineering, vol. 13, no. 2, pp. 20-28, 2023.
[CrossRef] [Publisher Link]
[16] Ziyu Su et al., “BCR-Net: A Deep Learning Framework to Predict Breast Cancer Recurrence from Histopathology Images,” PLOS ONE, vol. 18, no. 4, pp. 1-22, 2023.
[CrossRef] [Google Scholar] [Publisher Link]
[17] Yuhua Yao et al., “ICSDA: A Multi-Modal Deep Learning Model to Predict Breast Cancer Recurrence and Metastasis Risk by Integrating Pathological, Clinical and Gene Expression Data,” Briefings in Bioinformatics, vol. 23, no. 6, pp. 1-10, 2022.
[CrossRef] [Google Scholar] [Publisher Link]
[18] T.P. Latchoumi, T.P. Ezhilarasi, and K. Balamurugan, “Bio-Inspired Weighed Quantum Particle Swarm Optimization and Smooth Support Vector Machine Ensembles for Identification of Abnormalities in Medical Data,” SN Applied Sciences, vol. 1, no. 10, pp. 1-10, 2019.
[CrossRef] [Google Scholar] [Publisher Link]
[19] Jelmar Quist et al., “Random Forest Modelling of High-Dimensional Mixed-Type Data for Breast Cancer Classification,” Cancers, vol. 13, no. 5, pp. 1-15, 2021.
[CrossRef] [Google Scholar] [Publisher Link]
[20] Vincent Peter C. Magboo, and Ma. Sheila A. Magboo, “Machine Learning Classifiers on Breast Cancer Recurrences,” Procedia Computer Science, vol. 192, pp. 2742-2752, 2021.
[CrossRef] [Google Scholar] [Publisher Link]
[21] Leila Ghasem Ahmad et al., “Using Three Machine Learning Techniques for Predicting Breast Cancer Recurrence,” Journal of Health & Medical Informatics, vol. 4, no. 2, pp. 1-3, 2013.
[CrossRef] [Google Scholar] [Publisher Link]
[22] Jimin Guo et al., “Revealing Determinant Factors for Early Breast Cancer Recurrence by Decision Tree,” Information Systems Frontiers, vol. 19, pp. 1233-1241, 2017.
[CrossRef] [Google Scholar] [Publisher Link]
[23] Annemieke Witteveen et al., “Comparison of Logistic Regression and Bayesian Networks for Risk Prediction of Breast Cancer Recurrence,” Medical Decision Making, vol. 38, no. 7, pp. 822-833, 2018.
[CrossRef] [Google Scholar] [Publisher Link]
[24] Meha Desai, and Manan Shah, “An Anatomization on Breast Cancer Detection and Diagnosis Employing Multi-Layer Perceptron Neural Network (MLP) and Convolutional Neural Network (CNN),” Clinical eHealth, vol. 4, pp. 1-11, 2021.
[CrossRef] [Google Scholar] [Publisher Link]