Variable Kernel Feature Fusion and Transfer Learning for Pap Smear Image-Based Cervical Cancer Classification
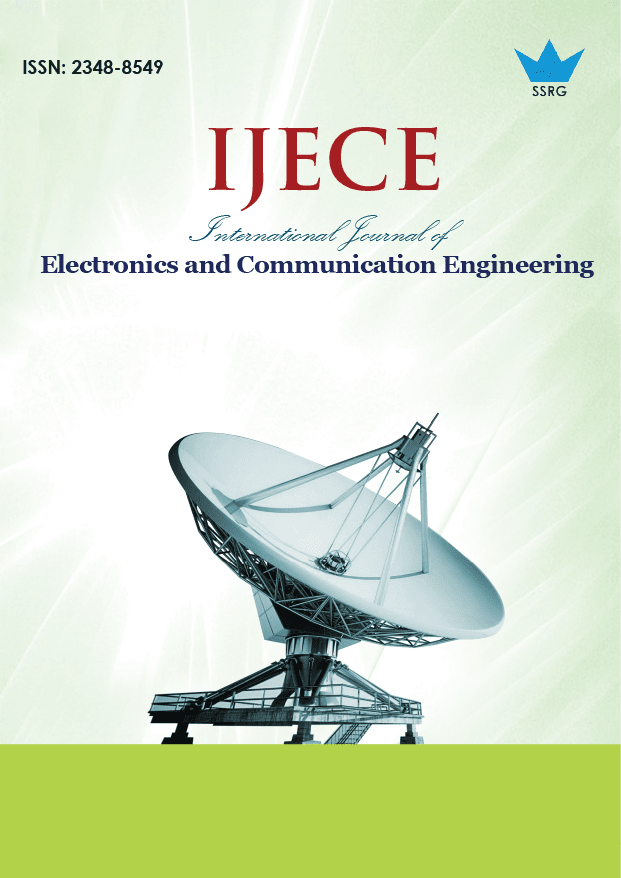
International Journal of Electronics and Communication Engineering |
© 2024 by SSRG - IJECE Journal |
Volume 11 Issue 11 |
Year of Publication : 2024 |
Authors : Priya S A, V Mary Amala Bai |
How to Cite?
Priya S A, V Mary Amala Bai, "Variable Kernel Feature Fusion and Transfer Learning for Pap Smear Image-Based Cervical Cancer Classification," SSRG International Journal of Electronics and Communication Engineering, vol. 11, no. 11, pp. 228-243, 2024. Crossref, https://doi.org/10.14445/23488549/IJECE-V11I11P119
Abstract:
Cervical cancer, a malignant tumour that forms in the cervix, significantly contributes to cancer-related mortality among women globally, making early diagnosis crucial for effective treatment. Pap smear images, which are microscopic images of cervical cells, are commonly used for the detection of abnormal cells that may lead to cervical cancer. This study introduces a novel classification approach, the Variable Kernel Feature Fusion-CNN (VKFF-CNN), which improves classification performance by fusing multi-scale features using convolutional layers with 3x3, 4x4, and 5x5 kernels. This architecture captures a diverse set of features, enhancing the ability of the model to accurately classify cervical cells. With an average accuracy of 98.03%, precision of 97.83%, recall of 97.11%, and an F1 score of 98.23%, the VKFF-CNN exhibited outstanding outcomes on the Herlev Pap Smear dataset. These results demonstrate that VKFF-CNN significantly outperforms traditional machine learning models. The model’s confusion matrix indicated fewer misclassifications, underscoring its robustness and effectiveness. Including batch normalization and the softmax activation function further enhanced the model’s stability and accurate classification. Overall, VKFF-CNN presents a promising advancement for automated cervical cancer screening, providing highly accurate and reliable detection.
Keywords:
Cervical Cancer, Herlev Pap Smear Dataset, Variable Kernel Feature Fusion-CNN, Multi-Scale Feature Extraction, Softmax activation.
References:
[1] P.B. Shanthi, K.S. Hareesha, and Ranjini Kudva, “Automated Detection and Classification of Cervical Cancer using Pap Smear Microscopic Images: A Comprehensive Review and Future Perspectives,” Engineered Science, vol. 19, pp. 20-41, 2022.
[CrossRef] [Google Scholar] [Publisher Link]
[2] Cervical Cancer, World Health Organization, 2024. [Online]. Available: https://www.who.int/news-room/fact-sheets/detail/cervical-cancer
[3] Aabid Shariff et al., “Automated Image Analysis for High-Content Screening and Analysis,” SLAS DISCOVERY: Advancing the Science of Drug Discovery, vol. 15, no. 7, pp. 726-734, 2010.
[CrossRef] [Google Scholar] [Publisher Link]
[4] Ewert Bengtsson, and Patrik Malm, “Screening for Cervical Cancer using Automated Analysis of PAP‐Smears,” Computational and Mathematical Methods in Medicine, vol. 2014, no. 1, pp. 1-12, 2014.
[CrossRef] [Google Scholar] [Publisher Link]
[5] Aslı Gençtav, Selim Aksoy, and Sevgen Önder, “Unsupervised Segmentation and Classification of Cervical Cell Images,” Pattern Recognition, vol. 45, no. 12, pp. 4151-4168, 2012.
[CrossRef] [Google Scholar] [Publisher Link]
[6] Jeovany Martínez-Mesa et al., “Inequalities in Pap Smear Screening for Cervical Cancer in Brazil,” Preventive Medicine, vol. 57, no. 4, pp. 366-371, 2013.
[CrossRef] [Google Scholar] [Publisher Link]
[7] Chuin-Mu Wang et al., “New Methods for Image De-Noising and Edge Enhancement in Cervical Smear Images Segmentation,” International Journal of Computer, Consumer and Control, vol. 2, no. 1, pp. 1-9, 2013.
[Google Scholar]
[8] Vasuja Devi Midasala et al., “MFEUsLNet: Skin Cancer Detection and Classification using Integrated AI with Multilevel Feature Extraction-Based Unsupervised Learning,” Engineering Science and Technology, an International Journal, vol. 51, pp. 1-17, 2024.
[CrossRef] [Google Scholar] [Publisher Link]
[9] Uday Pratap Singh Parmar et al., “Artificial Intelligence (AI) for Early Diagnosis of Retinal Diseases,” Medicina, vol. 60, no. 4, pp. 1-15, 2024.
[CrossRef] [Google Scholar] [Publisher Link]
[10] Arohee Sinha, and Tarun Kumar, “Enhancing Medical Diagnostics: Integrating AI for Precise Brain Tumour Detection,” Procedia Computer Science, vol. 235, pp. 456-467, 2024.
[CrossRef] [Google Scholar] [Publisher Link]
[11] I. Onur Sigirci, Abdulkadir Albayrak, and Gokhan Bilgin, “Detection of Mitotic Cells in Breast Cancer Histopathological Images Using Deep Versus Handcrafted Features,” Multimedia Tools and Applications, vol. 81, pp. 13179-13202, 2022.
[CrossRef] [Google Scholar] [Publisher Link]
[12] Muhammad Fazal Ijaz, and Marcin Woźniak, “Recent Advances in Deep Learning and Medical Imaging for Cancer Treatment,” Cancers, vol. 16, no. 4, pp. 1-5, 2024.
[CrossRef] [Google Scholar] [Publisher Link]
[13] Mohammad Zafer Khaliki, and Muhammet Sinan Başarslan, “Brain Tumor Detection from Images and Comparison with Transfer Learning Methods and 3-Layer CNN,” Scientific Reports, vol. 14, no. 1, pp. 1-10, 2024.
[CrossRef] [Google Scholar] [Publisher Link]
[14] Hanife Göker, “Detection of Cervical Cancer from Uterine Cervix Images Using Transfer Learning Architectures,” Eskişehir Technical University Journal of Science and Technology A-Applied Sciences and Engineering, vol. 25, no. 2, pp. 222-239, 2024.
[CrossRef] [Google Scholar] [Publisher Link]
[15] Emmanuel Ahishakiye, and Fredrick Kanobe, “Optimizing Cervical Cancer Classification through Transfer Learning and Kernel Methods: Analyzing the Performance of Deep Gaussian Processes and Support Vector Machines on Pap Smear Image Data,” Research Square, pp. 1-15, 2024.
[CrossRef] [Google Scholar] [Publisher Link]
[16] Sher Lyn Tan et al., “Cervical Cancer Classification from Pap Smear Images Using Deep Convolutional Neural Network Models,” Interdisciplinary Sciences: Computational Life Sciences, vol. 16, pp. 16-38, 2024.
[CrossRef] [Google Scholar] [Publisher Link]
[17] Bhaswati Singha Deo et al., “CerviFormer: A Pap Smear‐Based Cervical Cancer Classification Method Using Cross‐Attention and Latent Transformer,” International Journal of Imaging Systems and Technology, vol. 34, no. 2, 2024.
[CrossRef] [Google Scholar] [Publisher Link]
[18] Lenis Wong et al., “Deep Learning and Transfer Learning Methods to Effectively Diagnose Cervical Cancer from Liquid-Based Cytology Pap Smear Images,” International Journal of Online and Biomedical Engineering, vol. 19, no. 4, pp. 77-93, 2023.
[CrossRef] [Google Scholar] [Publisher Link]
[19] Omneya Attallah, “CerCan· Net: Cervical Cancer Classification Model via Multi-Layer Feature Ensembles of Lightweight CNNs and Transfer Learning. Expert Systems with Applications, vol. 229, 2023.
[CrossRef] [Google Scholar] [Publisher Link]
[20] Krishna Prasad Battula, and B. Sai Chandana, “Multi-Class Cervical Cancer Classification Using Transfer Learning-Based Optimized SE-ResNet152 Model in Pap Smear Whole Slide Images,” International Journal of Electrical and Computer Engineering Systems, vol. 14, no. 6, pp. 613-623, 2023.
[CrossRef] [Google Scholar] [Publisher Link]
[21] Madhura Kalbhor et al., “Hybridization of Deep Learning Pre-Trained Models with Machine Learning Classifiers and Fuzzy Min–Max Neural Network for Cervical Cancer Diagnosis,” Diagnostics, vol. 13, no. 7, pp. 1-16, 2023.
[CrossRef] [Google Scholar] [Publisher Link]
[22] Aya Haraz et al., “Deep Learning-Based Technique for the Perception of the Cervical Cancer,” Mansoura Engineering Journal, vol. 48, no. 3, pp. 1-9, 2023.
[CrossRef] [Google Scholar] [Publisher Link]
[23] Lidiya Wubshet Habtemariam, Elbetel Taye Zewde, and Gizeaddis Lamesgin Simegn, “Cervix Type and Cervical Cancer Classification System Using Deep Learning Techniques,” Medical Devices: Evidence and Research, vol. 15, pp. 163-176, 2022.
[CrossRef] [Google Scholar] [Publisher Link]
[24] Geert Litjens et al., “A Survey on Deep Learning in Medical Image Analysis,” Medical Image Analysis, vol. 42, pp. 60-88, 2017.
[CrossRef] [Google Scholar] [Publisher Link]
[25] Yannis Marinakis, Georgios Dounias, and Jan Jantzen, “Pap Smear Diagnosis Using a Hybrid Intelligent Scheme Focusing on Genetic Algorithm Based Feature Selection and Nearest Neighbor Classification,” Computers in Biology and Medicine, vol. 39, no. 1, pp. 69-78, 2009.
[CrossRef] [Google Scholar] [Publisher Link]
[26] Anant R. Bhatt, Amit Ganatra, and Ketan Kotecha, “Cervical Cancer Detection in Pap Smear Whole Slide Images Using ConvNet with Transfer Learning and Progressive Resizing,” PeerJ Computer Science, vol. 7, pp. 1-18, 2021.
[CrossRef] [Google Scholar] [Publisher Link]
[27] tf.keras.preprocessing.image.ImageDataGenerator, TensorFlow. [Online]. Available: https://www.tensorflow.org/api_docs/python/tf/keras/preprocessing/image/ImageDataGenerator
[28] Shadman Sakib et al., “An Overview of Convolutional Neural Network: Its Architecture and Applications,” Computer Science and Mathematics, pp. 1-6, 2019.
[Google Scholar] [Publisher Link]
[29] Sinno Jialin Pan, and Qiang Yang, “A Survey on Transfer Learning,” IEEE Transactions on Knowledge and Data Engineering, vol. 22, no. 10, pp. 1345-1359, 2010.
[CrossRef] [Google Scholar] [Publisher Link]
[30] Mark Sandler et al., “MobileNetV2: Inverted Residuals and Linear Bottlenecks,” 2018 IEEE/CVF Conference on Computer Vision and Pattern Recognition, pp. 4510-4520, 2018.
[CrossRef] [Google Scholar] [Publisher Link]
[31] B. Ashok, and P. Aruna, “Comparison of Feature Selection Methods for Diagnosis of Cervical Cancer Using SVM Classifier,” International Journal of Engineering Research and Applications, vol. 6, no. 1, pp. 94-99, 2016.
[Google Scholar] [Publisher Link]
[32] L. Lavanya Devi, and P. Thirumurugan, “Cervical Cancer Classification from Pap Smear Images Using Modified Fuzzy C Means, PCA, and KNN,” IETE Journal of Research, vol. 68, no. 3, pp. 1591-1598, 2021.
[CrossRef] [Google Scholar] [Publisher Link]