Enhanced Diabetes Detection from Foot Plantar Thermographs Using an Attention-Infused InceptionV3 Residual Block
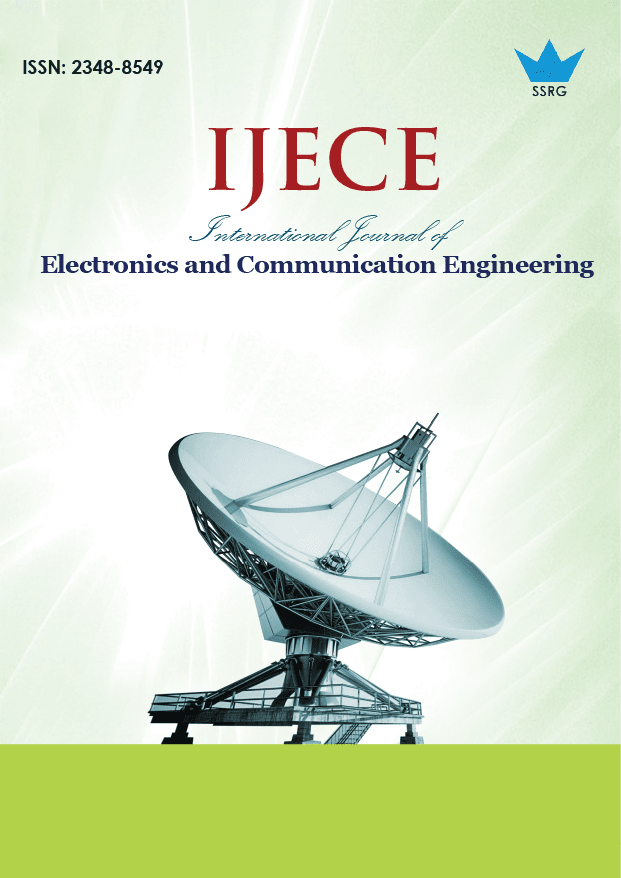
International Journal of Electronics and Communication Engineering |
© 2024 by SSRG - IJECE Journal |
Volume 11 Issue 11 |
Year of Publication : 2024 |
Authors : Nisanth Krishnan, V. Balamurugan |
How to Cite?
Nisanth Krishnan, V. Balamurugan, "Enhanced Diabetes Detection from Foot Plantar Thermographs Using an Attention-Infused InceptionV3 Residual Block," SSRG International Journal of Electronics and Communication Engineering, vol. 11, no. 11, pp. 257-271, 2024. Crossref, https://doi.org/10.14445/23488549/IJECE-V11I11P121
Abstract:
chronic illness, Diabetes Mellitus (DM), occurs due to the inability of the pancreas to produce insulin or to utilize the insulin it produces effectively. People with diabetes have increased risks of developing various life-threatening conditions, resulting in reduced quality of life and increased mortality. Diabetes causes long-term impairment and degradation of many body parts. Early intervention and treatment of diabetes can prevent extreme outcomes such as amputation. Thermography is a non-invasive technique commonly used to detect variations in temperature distribution in the foot region. So, in this study, a hybrid Deep Learning (DL) model incorporating a pretrained inception V3 with custom layers of attention and residual blocks is proposed to detect diabetes from foot plantar thermographic images efficiently. Thermography images of the foot from the foot plantar dataset are utilized in this study with various preprocessing and data augmentation techniques. The proposed model exhibits superior performance when compared to state-of-the-art methods with 95.71% accuracy, 97.85% precision, 93.83% recall, and 95.80 % F1score. In addition to standard evaluation metrics, the performance of the hybrid DL models is measured with Cohen’s kappa and the Area under the Curve (AUC). The outcomes indicate the model’s potential in real-time clinical application, resulting in more effective diabetic detection and management.
Keywords:
Diabetes, Thermography, Deep Learning, InceptionV3, Attention mechanism, Residual block.
References:
[1] Joseph R. Kraft, “Detection of Diabetes Mellitus in Situ (Occult Diabetes),” Laboratory Medicine, vol. 6, no. 2, pp. 10-22, 1975.
[CrossRef] [Google Scholar] [Publisher Link]
[2] Ali Mohebbi et al., “A Deep Learning Approach to Adherence Detection for Type 2 Diabetics,” 39th Annual International Conference of the IEEE Engineering in Medicine and Biology Society, Jeju, Korea (South), pp. 2896-2899, 2017.
[CrossRef] [Google Scholar] [Publisher Link]
[3] Aftab Alam et al., “Unveiling Diabetes: Categories, Genetics, Diagnostics, Treatments, and Future Horizons,” Current Diabetes Reviews, vol. 20, no. 4, pp. 10-22, 2024.
[CrossRef] [Google Scholar] [Publisher Link]
[4] Åke Lernmark, “Type 1 Diabetes,” Clinical Chemistry, vol. 45, no. 8, pp. 1331-1338, 1999.
[CrossRef] [Google Scholar] [Publisher Link]
[5] Rodolfo Valdez, “Detecting Undiagnosed Type 2 Diabetes: Family History as a Risk Factor and Screening Tool,” Journal of Diabetes Science and Technology, vol. 3, no. 4, pp. 722-726, 2009.
[CrossRef] [Google Scholar] [Publisher Link]
[6] Darcy Barry Carr, and Steven Gabbe, “Gestational Diabetes: Detection, Management, and Implications,” Clinical Diabetes, vol. 16, no. 1, pp. 1-4, 1998.
[Google Scholar] [Publisher Link]
[7] William J. Jeffcoate, and Keith G. Harding, “Diabetic Foot Ulcers,” The Lancet, vol. 361, no. 9368, pp. 1545-1551, 2003.
[Google Scholar] [Publisher Link]
[8] Maria Teresa Verrone Quilici et al., “Risk Factors for Foot Amputation in Patients Hospitalized for Diabetic Foot Infection,” Journal of Diabetes Research, vol. 2016, no. 1, pp. 1-8, 2016.
[CrossRef] [Google Scholar] [Publisher Link]
[9] Sara Ali Sulayman, Asma Rajab Qishqish, and Gofran Fathalla Dayhoom, “Early Detection of Diabetic Foot Using Thermal Imaging and Deep Learning Techniques,” African Journal of Advanced Pure and Applied Sciences, vol. 3, no. 3, pp. 564-567, 2024.
[Google Scholar] [Publisher Link]
[10] Garima Verma, “Leveraging Smart Image Processing Techniques for Early Detection of Foot Ulcers Using a Deep Learning Network,” Polish Journal of Radiology, vol. 89, pp. 368-377, 2024.
[CrossRef] [Google Scholar] [Publisher Link]
[11] Ikramullah Khosa et al., “Automatic Diabetic Foot Ulcer Recognition Using Multi-Level Thermographic Image Data,” Diagnostics, vol. 13, no. 16, pp. 1-19, 2023.
[CrossRef] [Google Scholar] [Publisher Link]
[12] Ilse Anahi Torres et al., “Proposal of a Non-Invasive Measurement of Physical Properties of Tissues in Patients with Diabetic Foot: Measurement Experiences in Diagnosed Patients,” Applied Sciences, vol. 13, no. 4, pp. 1-16, 2023.
[CrossRef] [Google Scholar] [Publisher Link]
[13] Natalia Arteaga-Marrero et al., “State-of-the-Art Features for Early-Stage Detection of Diabetic Foot Ulcers Based on Thermograms,” Biomedicines, vol. 11, no. 12, pp. 1-16, 2023.
[CrossRef] [Google Scholar] [Publisher Link]
[14] Zhenjie Cao et al., “Diabetic Plantar Foot Segmentation in Active Thermography Using a Two-Stage Adaptive Gamma Transform and a Deep Neural Network,” Sensors, vol. 23, no. 20, pp. 1-17, 2023.
[CrossRef] [Google Scholar] [Publisher Link]
[15] J. Yogapriya et al., “Automated Detection of Infection in Diabetic Foot Ulcer Images Using Convolutional Neural Network,” Journal of Healthcare Engineering, vol. 2022, no. 1, pp. 1-12, 2022.
[CrossRef] [Google Scholar] [Publisher Link]
[16] Israel Cruz-Vega et al., “Deep Learning Classification for Diabetic Foot Thermograms,” Sensors, vol. 20, no. 6, pp. 1-22, 2020.
[CrossRef] [Google Scholar] [Publisher Link]
[17] Amith Khandakar et al., “A Novel Machine Learning Approach for Severity Classification of Diabetic Foot Complications Using Thermogram Images,” Sensors, vol. 22, no. 11, pp. 1-23, 2022.
[CrossRef] [Google Scholar] [Publisher Link]
[18] Andrés Anaya-Isaza, and Matha Zequera-Diaz, “Detection of Diabetes Mellitus with Deep Learning and Data Augmentation Techniques on Foot Thermography,” IEEE Access, vol. 10, pp. 59564-59591, 2022.
[CrossRef] [Google Scholar] [Publisher Link]
[19] Vuppala Adithya Sairam, Thermography Images of Diabetic Foot, Kaggle, 2022. [Online]. Available: https://www.kaggle.com/datasets/vuppalaadithyasairam/thermography-images-of-diabetic-foot
[20] Cheng Wang et al., “Pulmonary Image Classification Based on Inception-v3 Transfer Learning Model,” IEEE Access, vol. 7, pp. 146533-146541, 2019.
[CrossRef] [Google Scholar] [Publisher Link]
[21] Paolo Arena et al., “Image Processing for Medical Diagnosis Using CNN,” Nuclear Instruments and Methods in Physics Research Section A: Accelerators, Spectrometers, Detectors and Associated Equipment, vol. 497, no. 1, pp. 174-178, 2003.
[CrossRef] [Google Scholar] [Publisher Link]
[22] Xiang Li et al., “Deep Learning Attention Mechanism in Medical Image Analysis: Basics and Beyonds,” International Journal of Network Dynamics and Intelligence, vol. 2, no. 1, pp. 1-24, 2023.
[CrossRef] [Google Scholar] [Publisher Link]
[23] Zahra Tabatabaei et al., “Residual Block Convolutional Auto Encoder in Content-Based Medical Image Retrieval,” IEEE 14th Image, Video, and Multidimensional Signal Processing Workshop, Nafplio, Greece, pp. 1-5, 2022.
[CrossRef] [Google Scholar] [Publisher Link]