Beyond Static: A Satellite Image Change Detection Using Absolute Convolutional Prior Fusion (AC-PF) Approach
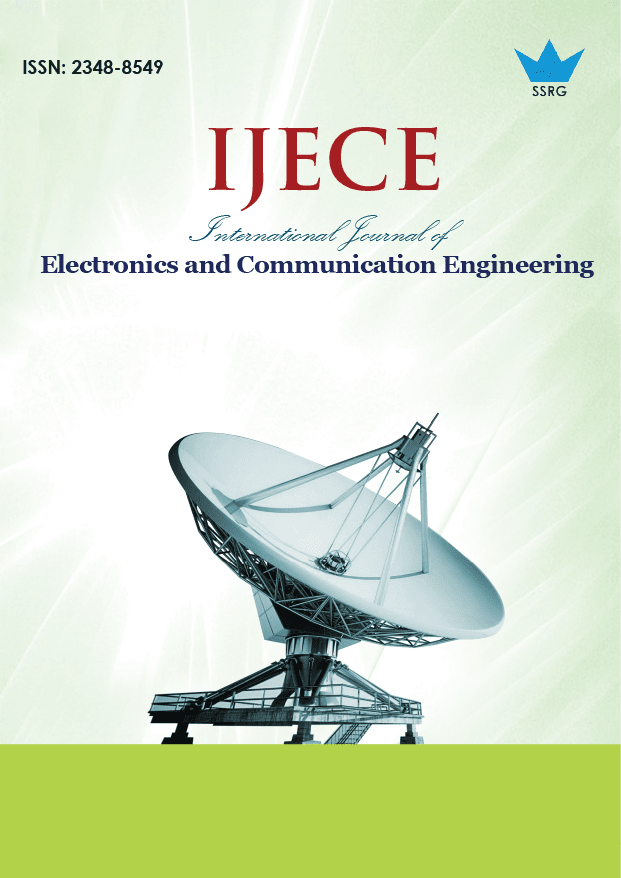
International Journal of Electronics and Communication Engineering |
© 2024 by SSRG - IJECE Journal |
Volume 11 Issue 11 |
Year of Publication : 2024 |
Authors : Aiswarya Jeevan, S Amala Shanthi |
How to Cite?
Aiswarya Jeevan, S Amala Shanthi, "Beyond Static: A Satellite Image Change Detection Using Absolute Convolutional Prior Fusion (AC-PF) Approach," SSRG International Journal of Electronics and Communication Engineering, vol. 11, no. 11, pp. 272-285, 2024. Crossref, https://doi.org/10.14445/23488549/IJECE-V11I11P122
Abstract:
Urbanization is a dynamic process marked by rapid and intricate land use, infrastructure, and population distribution transformations. Monitoring these changes for sustainable urban development, resource management, and disaster readiness is imperative. Satellite image Change Detection (CD) has emerged as a potent tool for comprehensively and efficiently evaluating urban changes over time. Techniques for CD facilitate identifying, characterising, and quantifying modifications in land cover, land use, and natural phenomena. This capability is pivotal for environmental monitoring, resource management, and disaster response. Detecting changes in urban expansion, deforestation, agricultural practices, and natural disasters contributes to informed decision-making and sustainable development. Whether it is gradual urban expansion or sudden infrastructural developments, the ability to detect changes offers valuable insights into the patterns and drivers of urban growth. Integrating remote sensing technologies and advanced image processing techniques has remarkably enhanced the accuracy and efficiency of CD in urban environments. These methods enable the identification of land cover changes, such as converting green spaces to built-up areas or adjusting transportation networks. This paper introduces an effective CD model that incorporates a deep learning approach. The proposed architecture is directly inspired by the U-Net model, adapted into the AC-PF while considering the available training data. Finally, the Dice similarity score is computed for a specific image compared to the ground truth images and the corresponding input images.
Keywords:
Change detection, Convolutional neural network, Deep learning, Image processing, Remote sensing, Satellite imagery, Urban monitoring.
References:
[1] R.C. Luo, and M.G. Kay, “Multisensor Integration and Fusion in Intelligent Systems,” IEEE Transactions on Systems, Man, and Cybernetics, vol. 19, no. 5, pp. 901-931, 1989.
[CrossRef] [Google Scholar] [Publisher Link]
[2] Y. Tzeng, S. Chiu, and K. Chen, “Automatic Change Detections from SAR Images Using Fractal Dimension,” IEEE International Symposium on Geoscience and Remote Sensing, Denver, CO, USA, pp.759-762, 2006.
[CrossRef] [Google Scholar] [Publisher Link]
[3] Lisa Gottesfeld Brown, “A Survey of Image Registration Techniques,” ACM Computing Surveys, vol. 24, no. 4, pp. 325-376, 1992.
[CrossRef] [Google Scholar] [Publisher Link]
[4] B. Qiu et al., “Multi-Block PCA Method for Image Change Detection,” 12th International Conference on Image Analysis and Processing, Mantova, Italy, pp. 385-390, 2003.
[CrossRef] [Google Scholar] [Publisher Link]
[5] R.J. Radke et al., “Image Change Detection Algorithms: A Systematic Survey,” IEEE Transactions on Image Processing, vol. 14, no. 3, pp.294-307, 2005.
[CrossRef] [Google Scholar] [Publisher Link]
[6] Ashbindu Singh, “Digital Change Detection Techniques Using Remotely-Sensed Data,” International Journal of Remote Sensing, vol. 10, no. 6, pp. 989-1003, 1989.
[CrossRef] [Google Scholar] [Publisher Link]
[7] Jordi Inglada, and Grgoire Mercier, “A New Statistical Similarity Measure for Change Detection in Multitemporal SAR Images and Its Extension to Multiscale Change Analysis,” IEEE Transactions on Geoscience and Remote Sensing, vol. 45, no. 5, pp. 1432-1445, 2007.
[CrossRef] [Google Scholar] [Publisher Link]
[8] Ebrahim Ghaderpour, and Tijana Vujadinovic, “Change Detection within Remotely Sensed Satellite Image Time Series via Spectral Analysis,” Remote Sensing, vol. 12, no. 23, pp. 1-27, 2020.
[CrossRef] [Google Scholar] [Publisher Link]
[9] Jie Chen et al., “DASNet: Dual Attentive Fully Convolutional Siamese Networks for Change Detection in High-Resolution Satellite Images,” IEEE Journal of Selected Topics in Applied Earth Observations and Remote Sensing, vol. 14, pp. 1194-1206, 2021.
[CrossRef] [Google Scholar] [Publisher Link]
[10] Xiuwei Zhang et al., “DifUnet++: A Satellite Images Change Detection Network Based on Unet++ and Differential Pyramid,” IEEE Geoscience and Remote Sensing Letters, vol. 19, pp. 1-5, 2022.
[CrossRef] [Google Scholar] [Publisher Link]
[11] Caijun Ren et al., “Unsupervised Change Detection in Satellite Images with Generative Adversarial Network,” IEEE Transactions on Geoscience and Remote Sensing, vol. 59, no. 12, pp. 10047-10061, 2021.
[CrossRef] [Google Scholar] [Publisher Link]
[12] Ekaterina Kalinicheva et al., “Unsupervised Change Detection Analysis in Satellite Image Time Series Using Deep Learning Combined With Graph-Based Approaches,” IEEE Journal of Selected Topics in Applied Earth Observations and Remote Sensing, vol. 13, pp. 1450-1466, 2020.
[CrossRef] [Google Scholar] [Publisher Link]
[13] Xuan Hou et al., “High-Resolution Triplet Network with Dynamic Multiscale Feature for Change Detection on Satellite Images,” ISPRS Journal of Photogrammetry and Remote Sensing, vol. 177, pp. 103-115, 2021.
[CrossRef] [Google Scholar] [Publisher Link]
[14] Hichem Sahbi, Sebastien Deschamps, and Andrei Stoian, “Active Learning for Interactive Satellite Image Change Detection,” Arxiv, pp. 1-9, 2021.
[CrossRef] [Google Scholar] [Publisher Link]
[15] Xin Huang, Yinxia Cao, and Jiayi Li, “An Automatic Change Detection Method for Monitoring Newly Constructed Building Areas Using Time-Series Multi-View High-Resolution Optical Satellite Images,” Remote Sensing of Environment, vol. 244, 2020.
[CrossRef] [Google Scholar] [Publisher Link]
[16] Wenqiang Xi et al., “A Spatiotemporal Cube Model for Analyzing Satellite Image Time Series: Application to Land-Cover Mapping and Change Detection,” Remote Sensing of Environment, vol. 231, 2019.
[CrossRef] [Google Scholar] [Publisher Link]
[17] Kaiyu Li, Zhe Li, and Sheng Fang, “Siamese NestedUNet Networks for Change Detection of High Resolution Satellite Image,” Proceedings of the 2020 1st International Conference on Control, Robotics and Intelligent System, pp. 42-48, 2021.
[CrossRef] [Google Scholar] [Publisher Link]
[18] Ran Jing, Zhaoning Gong, and Hongliang Guan, “Land Cover Change Detection with VHR Satellite Imagery Based on Multi-Scale SLIC-CNN and SCAE Features,” IEEE Access, vol. 8, pp. 228070-228087, 2020.
[CrossRef] [Google Scholar] [Publisher Link]
[19] Yi Zhang et al., Coarse-to-Fine Satellite Images Change Detection Framework via Boundary-Aware Attentive Network,” Sensors, vol. 20, no. 23, pp. 1-21, 2020.
[CrossRef] [Google Scholar] [Publisher Link]
[20] Ahram Song, Yongil Kim, and Youkyung Han, “Uncertainty Analysis for Object-Based Change Detection in Very High-Resolution Satellite Images Using Deep Learning Network,” Remote Sensing, vol. 12, no. 15, pp. 1-26, 2020.
[CrossRef] [Google Scholar] [Publisher Link]
[21] Lukas Kondmann et al., “Spatial Context Awareness for Unsupervised Change Detection in Optical Satellite Images,” IEEE Transactions on Geoscience and Remote Sensing, vol. 60, pp. 1-15, 2022.
[CrossRef] [Google Scholar] [Publisher Link]
[22] Ramen Pal et al., “Very High-Resolution Satellite Image Segmentation Using Variable-Length Multi-Objective Genetic Clustering for Multi-Class Change Detection,” Journal of King Saud University - Computer and Information Sciences, vol. 34, no. 10, pp. 9964-9976, 2022.
[CrossRef] [Google Scholar] [Publisher Link]
[23] Soumik Rakshit, Onera Satellite Change Detection Dataset, Kaggle, 2019. [Online]. Available: https://www.kaggle.com/datasets/soumikrakshit/onera-satellite-change-detection-dataset
[24] Jianxin Wu, “Introduction to Convolutional Neural Networks,” National Key Lab for Novel Software Technology, Nanjing University. China, pp. 1-31, 2017.
[Google Scholar] [Publisher Link]
[25] Xiao-Xia Yin et al., “U-Net-Based Medical Image Segmentation,” Journal of Healthcare Engineering, vol. 2022, no. 1, pp.1-17, 2022.
[CrossRef] [Google Scholar] [Publisher Link]
[26] Hossein Gholamalinezhad, and Hossein Khosravi, “Pooling Methods in Deep Neural Networks, a Review,” Arxiv, pp. 1-16, 2020.
[CrossRef] [Google Scholar] [Publisher Link]