Predicting Water Quality Parameters in Mahseer Fish Farming Using Machine Learning Techniques
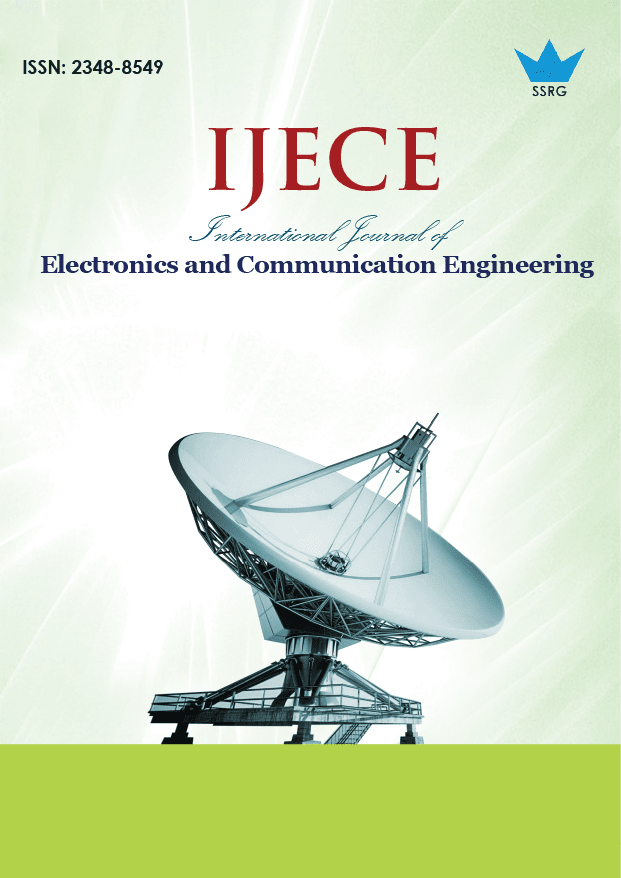
International Journal of Electronics and Communication Engineering |
© 2024 by SSRG - IJECE Journal |
Volume 11 Issue 11 |
Year of Publication : 2024 |
Authors : Nuradin Mohamed Abdikadir, Ahmad Shahidan Abdullah, Husein Osman Abdullahi, Abdikarim Abi Hassan |
How to Cite?
Nuradin Mohamed Abdikadir, Ahmad Shahidan Abdullah, Husein Osman Abdullahi, Abdikarim Abi Hassan, "Predicting Water Quality Parameters in Mahseer Fish Farming Using Machine Learning Techniques," SSRG International Journal of Electronics and Communication Engineering, vol. 11, no. 11, pp. 286-296, 2024. Crossref, https://doi.org/10.14445/23488549/IJECE-V11I11P123
Abstract:
Mahseer fish farming faces challenges in maintaining optimal water quality, essential for fish health and growth. Poor water quality can lead to stress, disease, and mortality, impacting productivity. This study compares Random forest Regression (RF) and Support Vector Regression (SVR) models in predicting water quality parameters, such as pH, dissolved oxygen (DO), and temperature. The RF model outperformed SVR, showing superior accuracy with lower Mean Squared Error (MSE) and Mean Absolute Error (MAE) and higher R-squared values (99% for DO, 98% for temperature, and 95% for pH). RF’s superior performance makes it a reliable tool for tracking water quality trends and fluctuations. Recommendations for enhanced monitoring include extending data turbidity and capturing seasonal and long-term trends, integrating sensors for additional parameters like ammonia and turbidity, and developing a user-friendly mobile app for real-time data and alerts. These improvements aim to support the sustainability and productivity of Mahseer fish farming.
Keywords:
Aquaculture, Machine learning, RF, SVR, Water quality.
References:
[1] Haochen Hou et al., “An Environmental Impact Assessment of Largemouth Bass (Micropterus Salmoides) Aquaculture in Hangzhou, China,” Sustainability (Switzerland), vol. 15, no. 16, pp. 1-13, 2023.
[CrossRef] [Google Scholar] [Publisher Link]
[2] Lahsen Ababouch et al., “Value Chains and Market Access for Aquaculture Products,” Journal of the World Aquaculture Society, vol. 54, no. 2, pp. 527-553, 2023.
[CrossRef] [Google Scholar] [Publisher Link]
[3] I. Mulyani et al., “The Ecological Analysis of the Habitat of Semah Fish (Tor Tambroides, Bleeker 1854) in the Kampar Kanan Hulu River Koto Kampar Hulu District, Kampar Regency, Riau Province,” IOP Conference Series: Earth and Environmental Science, 11th International and National Seminar on Fisheries and Marine Science, Pekanbaru, Indonesia, vol. 1118, pp. 1-8, 2022.
[CrossRef] [Google Scholar] [Publisher Link]
[4] Peda Gopi Arepalli, and Jairam Naik Khetavath, “An IoT Framework for Quality Analysis of Aquatic Water Data Using Time-Series Convolutional Neural Network,” Environmental Science and Pollution Research, vol. 30, pp. 125275-125294, 2023.
[CrossRef] [Google Scholar] [Publisher Link]
[5] Harsh Dadhaneeya, Prabhat K. Nema, and Vinkel Kumar Arora, “Internet of Things in Food Processing and its Potential in Industry 4.0 Era: A Review,” Trends in Food Science and Technology, vol. 139, 2023.
[CrossRef] [Google Scholar] [Publisher Link]
[6] Abu Taher Tamim et al., “Development of IoT Based Fish Monitoring System for Aquaculture,” Intelligent Automation & Soft Computing, vol. 32, no. 1, pp. 55-71, 2022.
[CrossRef] [Google Scholar] [Publisher Link]
[7] Andrew B. Gallemit, “Water Monitoring and Analysis System: Validating an IoT-Enabled Prototype towards Sustainable Aquaculture,” International Journal of Advanced Multidisciplinary Studies, vol. 3, no. 6, pp. 496-517, 2023.
[Google Scholar] [Publisher Link]
[8] Danial Mohammad Ghazali et al., “Smart IoT Based Monitoring System for Fish Breeding,” Journal of Advanced Research in Applied Mechanics, vol. 104, no. 1, pp. 1-11, 2023.
[CrossRef] [Google Scholar] [Publisher Link]
[9] Illa Iza Suhana Shamsuddin, Zalinda Othman, and Nor Samsiah Sani, “Water Quality Index Classification Based on ML: A Case from the Langat River Basin Model,” Water, vol. 14, no. 19, pp. 1-20, 2022.
[CrossRef] [Google Scholar] [Publisher Link]
[10] Shili Zhao et al., “Application of ML in Intelligent Fish Aquaculture: A Review,” Aquaculture, vol. 540, 2021.
[CrossRef] [Google Scholar] [Publisher Link]
[11] Jianlong Xu et al., “An Alternative to Laboratory Testing: Random Forest-Based Water Quality Prediction Framework for Inland and Nearshore Water Bodies,” Water, vol. 13, no. 22, pp. 1-19, 2021.
[CrossRef] [Google Scholar] [Publisher Link]
[12] Melinda Mei Lin Lau et al., “A Review on the Emerging Asian Aquaculture Fish, the Malaysian Mahseer (Tor tambroides): Current Status and the Way Forward,” Proceedings of the Zoological Society, vol. 74, no. 2, pp. 227-237, 2021.
[CrossRef] [Google Scholar] [Publisher Link]
[13] K.C. Ahmed Jala et al., “Phototrophic Purple Bacteria as Feed Supplement on the Growth, Feed Utilization and Body Compositions of Malaysian Mahseer, Tor Tambroides Juveniles,” Malaysian Science, vol. 45, no. 1, pp. 135-140, 2016.
[Google Scholar] [Publisher Link]
[14] Nur Syuhada Iskandar et al., “Elevated Carbon Dioxide and its Impact on Growth, Blood Properties, and Vertebral Column of Freshwater Fish Mahseer, Tor tambroides Juveniles,” Fishes, vol. 8, no. 6, pp. 1-12, 2023.
[CrossRef] [Google Scholar] [Publisher Link]
[15] Mohammod Kamruzzaman Hossain et al., “Growth Performance, Fatty Acid Profile, Gut, and Muscle Histo-Morphology of Malaysian Mahseer, Tor Tambroides Post Larvae Fed Short-Term Host Associated Probiotics,” Aquaculture and Fisheries, vol. 9, no. 1, pp. 35-45, 2024.
[CrossRef] [Google Scholar] [Publisher Link]
[16] Aidil Ikhwan Redhwan et al., “Mahseer in Malaysia: A Review of Feed for Cultured Tor Tambroides and Tor Tambra,” Bioscience Research, vol. 19, no. SI-1, pp. 349-359, 2022.
[Google Scholar] [Publisher Link]
[17] P. Kirankumar et al., “Smart Monitoring and Water Quality Management in Aquaculture using lOT and ML,” IEEE International Conference on Intelligent Systems, Smart and Green Technologies, Visakhapatnam, India, pp. 32-36, 2021.
[CrossRef] [Google Scholar] [Publisher Link]
[18] Azimbek Khudoyberdiev et al., “Enhanced Water Quality Control Based on Predictive Optimization for Smart Fish Farming,” Computers, Materials and Continua, vol. 75, no. 3, pp. 5471-5499, 2023.
[CrossRef] [Google Scholar] [Publisher Link]
[19] Munesh Singh, Kshira Sagar Sahoo, and Anand Nayyar, “Sustainable IoT Solution for Freshwater Aquaculture Management,” IEEE Sensors Journal, vol. 22, no. 16, pp. 16563-16572, 2022.
[CrossRef] [Google Scholar] [Publisher Link]
[20] Zeenat Shareef, and S.R.N. Reddy, “Design and Development of IoT-Based Framework for Indian Aquaculture,” Intelligent Communication, Control and Devices, Advances in Intelligent Systems and Computing, vol. 989, pp. 195-201, 2020.
[CrossRef] [Google Scholar] [Publisher Link]
[21] Xuxiang Ta, and Yaoguang Wei, “Research on a Dissolved Oxygen Prediction Method for Recirculating Aquaculture Systems Based on a Convolution Neural Network,” Computers and Electronics in Agriculture, vol. 145, pp. 302-310, 2018. [CrossRef] [Google Scholar] [Publisher Link]
[22] Maxime Lafont et al., “Back to the Future: IoT to Improve Aquaculture: Real-Time Monitoring and Algorithmic Prediction of Water Parameters for Aquaculture Needs,” Global IoT Summit, Aarhus, Denmark, pp. 1-6, 2019.
[CrossRef] [Google Scholar] [Publisher Link]
[23] Marzia Ahmed et al., “Analyzing the Quality of Water and Predicting the Suitability for Fish Farming Based on IoT in the Context of Bangladesh,” International Conference on Sustainable Technologies for Industry 4.0, Dhaka, Bangladesh, pp. 1-5, 2019.
[CrossRef] [Google Scholar] [Publisher Link]
[24] Guandong Gao, Ke Xiao, and Miaomiao Chen, “An Intelligent Iot-Based Control and Traceability System to Forecast and Maintain Water Quality in Freshwater Fish Farms,” Computers and Electronics in Agriculture, vol. 166, 2019.
[CrossRef] [Google Scholar] [Publisher Link]