An Effective Segmentation of MRI Images Combining Threshold and Hybrid Particle Swarm Optimization (HPSO-T) for Lung, Bone and Brain (LBB)
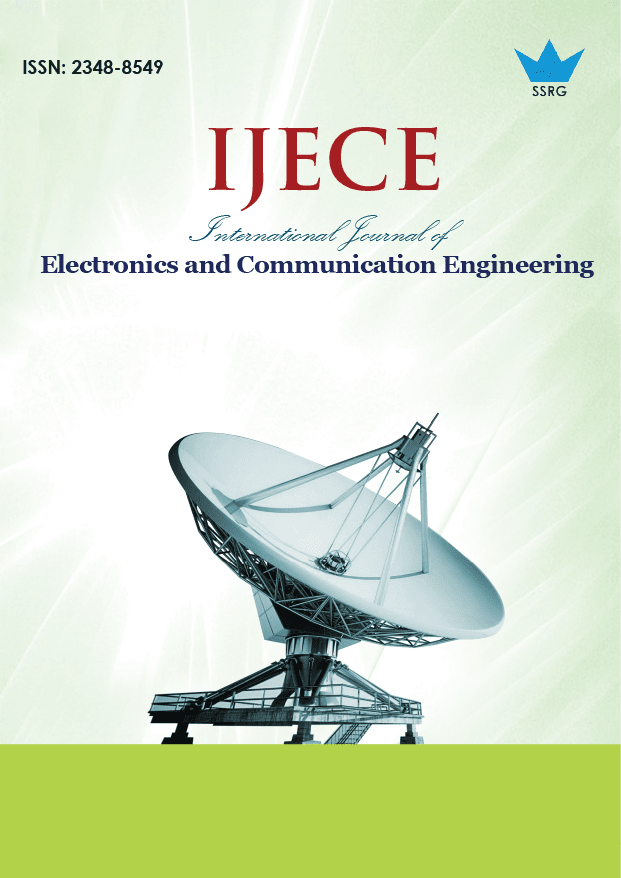
International Journal of Electronics and Communication Engineering |
© 2024 by SSRG - IJECE Journal |
Volume 11 Issue 12 |
Year of Publication : 2024 |
Authors : N. Raghapriya, N. Aswini, G. Savitha |
How to Cite?
N. Raghapriya, N. Aswini, G. Savitha, "An Effective Segmentation of MRI Images Combining Threshold and Hybrid Particle Swarm Optimization (HPSO-T) for Lung, Bone and Brain (LBB)," SSRG International Journal of Electronics and Communication Engineering, vol. 11, no. 12, pp. 92-99, 2024. Crossref, https://doi.org/10.14445/23488549/IJECE-V11I12P109
Abstract:
Segmentation in medical imaging is one of the fundamental problems in image processing. Perceptual completion and recognition during picture segmentation are the issues with image segmentation. Machine vision-based image threshold segmentation is an essential detecting tool. The issue of time consumption arises with the traditional threshold picture segmentation method. However, optimization techniques can help to resolve these problems. An effective optimization technique is needed to determine the ideal threshold. The thresholding will become more computationally intensive with increasing thresholds. This research proposed Hybrid Particle Swarm Optimization with Thresholding (HPSO-T) technique used for image segmentation to assess the MRI medical Image for detecting and managing various tumors in Lung, Brain and Bone-(LBB). This work extracts the MRI scan pictures using the LBB data acquired from the Kaggle website. The suggested segmentation methodology outperforms the other two segmentation approaches in the market with a Dice Index of 0.93.
Keywords:
Segmentation, MRI images, LBB, HPSO-T, Optimization.
References:
[1] Mohamed H. Merzban, and Mahmoud Elbayoumi, “Efficient Solution of Otsu Multilevel Image Thresholding: A Comparative Study,” Expert Systems with Applications, vol. 116, pp. 299–309, 2019.
[CrossRef] [Google Scholar] [Publisher Link]
[2] Zhu Liangkuan et al., “Forest Canopy Image Segmentation Based on Improved 3D Otsu Method,” Computer Engineering, vol. 45, no. 1, 2019.
[CrossRef] [Publisher Link]
[3] Lei Xiangxiao et al., “Research on Image Segmentation based on Equivalent 3-D Entropy and Whale Optimization Algorithm,” Computer Engineering, vol. 45, no. 4, pp. 217-222, 2019.
[CrossRef] [Publisher Link]
[4] Nabanita Mahata, and Jamuna Kanta Sing, “A Novel Fuzzy Clustering Algorithm by Minimizing Global and Spatially Constrained Likelihood-based Local Entropies for Noisy 3D Brain MR Image Segmentation,” Applied Soft Computing Journal, vol. 90, 2020.
[CrossRef] [Google Scholar] [Publisher Link]
[5] Xiaofeng Yue, and Hongbo Zhang, “Modified Hybrid Bat Algorithm with Genetic Crossover Operation and Smart Inertia Weight for Multilevel Image Segmentation,” Applied Soft Computing Journal, vol. 90, 2020.
[CrossRef] [Google Scholar] [Publisher Link]
[6] Himanshu Mittal, and Mukesh Saraswat, “An Optimum Multi-level Image Thresholding Segmentation using Non-local Means 2D Histogram and Exponential Kbest Gravitational Search Algorithm,” Engineering Applications of Artificial Intelligence, vol. 71, pp. 226–235, 2018.
[CrossRef] [Google Scholar] [Publisher Link]
[7] S. Pare et al., “A New Technique for Multilevel Color Image Thresholding based on Modified Fuzzy Entropy and Lévy Flight Firefly Algorithm,” Computers & Electrical Engineering, vol. 70, pp. 476–495, 2018.
[CrossRef] [Google Scholar] [Publisher Link]
[8] Jiafu Li et al., “Multilevel Thresholding Selection Based on Variational Mode Decomposition for Image Segmentation,” Signal Processing, vol. 147, pp. 80–91, 2018.
[CrossRef] [Google Scholar] [Publisher Link]
[9] K. Manikandan, and B. Sudhakar, “Hybrid Optimization Algorithm for Multi-level Image Thresholding Using Salp Swarm Optimization Algorithm and Ant Colony Optimization,” International Journal of Electrical and Electronic Engineering & Telecommunications, vol. 12, no. 6, pp. 1-13, 2023.
[Google Scholar] [Publisher Link]
[10] Shoffan Saifullah, and Rafał Dreżewski, “Advanced Medical Image Segmentation Enhancement: A Particle-Swarm-Optimization-Based Histogram Equalization Approach,” Applied Sciences, vol. 14, no. 2, pp. 1-26, 2024.
[CrossRef] [Google Scholar] [Publisher Link]
[11] Shadi Mahmoodi Khaniabadi et al., “Comparative Review on Traditional and Deep Learning Methods for Medical Image Segmentation,” 2023 IEEE 14th Control and System Graduate Research Colloquium (ICSGRC), Shah Alam, Malaysia, pp. 45-50, 2023.
[CrossRef] [Google Scholar] [Publisher Link]
[12] Sandra Jardim, Joao António, and Carlos Mora, “Image Thresholding Approaches for Medical Image Segmentation - Short Literature Review,” Procedia Computer Science, vol. 219, pp. 1485-1492, 2023.
[CrossRef] [Google Scholar] [Publisher Link]
[13] Yuncong Feng et al., “A Novel Interval Iterative Multi-Thresholding Algorithm Based on Hybrid Spatial Filter and Region Growing for Medical Brain MR Images,” Applied Sciences, vol. 13, no. 2, pp. 1-18, 2023.
[CrossRef] [Google Scholar] [Publisher Link]
[14] Yuhan Xie et al., “Detect, Grow, Seg: A Weakly Supervision Method for Medical Image Segmentation based on Bounding Box,” Biomedical Signal Processing and Control, vol. 86, part-A, 2023.
[CrossRef] [Google Scholar] [Publisher Link]
[15] Poonam Jaglan, Rajeshwar Dass, and Manoj Duhan, “A Comparative Analysis of Various Image Segmentation Techniques,” Proceedings of 2nd International Conference on Communication, Computing and Networking, 359–374, 2018.
[CrossRef] [Google Scholar] [Publisher Link]
[16] Abdullah Sarhan, Jon Rokne, and Reda Alhajj, “Glaucoma Detection using Image Processing Techniques: A Literature Review,” Computerized Medical Imaging and Graphics, vol. 78, 2019.
[CrossRef] [Google Scholar] [Publisher Link]
[17] Mohamed T. Bennai et al., “A Stochastic Multi-agent Approach for Medical-image Segmentation: Application to Tumor Segmentation in Brain MR Images,” Artificial Intelligence in Medicine, vol. 110, 2020.
[CrossRef] [Google Scholar] [Publisher Link]
[18] Erena Siyoum Biratu et al., “A Survey of Brain Tumor Segmentation and Classification Algorithms,” Journal of Imaging, vol. 7, no. 9, pp. 1-30, 2021.
[CrossRef] [Google Scholar] [Publisher Link]
[19] Erena Siyoum Biratu et al., “Enhanced Region Growing for Brain Tumor MR Image Segmentation,” Journal of Imaging, vol. 7, no. 2, pp. 1-19, 2021.
[CrossRef] [Google Scholar] [Publisher Link]
[20] H.M. Naveen, C. Naveena, and V.N. Manjunath Aradhya, “An Approach for Classification of Lung Nodules,” Tumor Discovery, vol. 2, no. 1, 2023.
[CrossRef] [Google Scholar] [Publisher Link]
[21] Victor Hugo C. de Albuquerque et al., “Special Issue on Bio-inspired Optimization Techniques for Biomedical Data Analysis: Methods and Applications,” Applied Soft Computing, vol. 95, 2020.
[CrossRef] [Google Scholar] [Publisher Link]
[22] Jiawei Guo et al., “A Survey on Image Enhancement for Low-light Images,” Heliyon, vol. 9, no. 4, pp. 1-26, 2023.
[Google Scholar] [Publisher Link]
[23] A. Asuntha, N. Singh, and A. Srinivasan, “PSO, Genetic Optimization and SVM Algorithm used for Lung Cancer Detection,” Journal of Chemical and Pharmaceutical Research, vol. 8, no. 6, pp. 351–359, 2016.
[Google Scholar]
[24] P. Bhuvaneswari, and A. Brintha Therese, “Detection of Cancer in Lung with K-NN Classification using Genetic Algorithm,” International Conference on Nanomaterials and Technologies, vol. 10, pp. 433–440, 2014.
[CrossRef] [Google Scholar] [Publisher Link]