Enhancing Student Academic Performance Forecasting in Technical Education: A Cutting-edge Hybrid Fusion Method
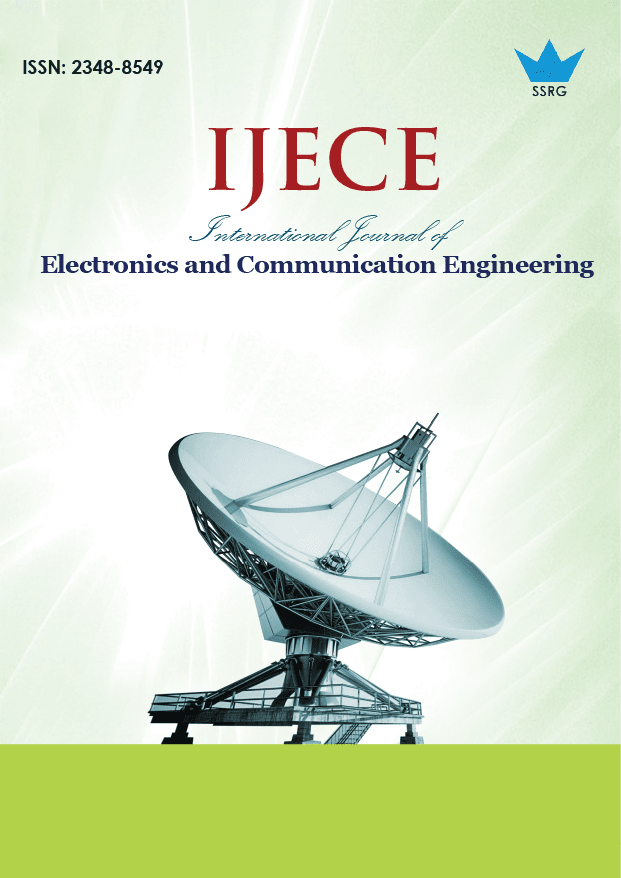
International Journal of Electronics and Communication Engineering |
© 2024 by SSRG - IJECE Journal |
Volume 11 Issue 12 |
Year of Publication : 2024 |
Authors : K. Rajesh Kannan, K. T. Meena Abarna, S. Vairachilai |
How to Cite?
K. Rajesh Kannan, K. T. Meena Abarna, S. Vairachilai, "Enhancing Student Academic Performance Forecasting in Technical Education: A Cutting-edge Hybrid Fusion Method," SSRG International Journal of Electronics and Communication Engineering, vol. 11, no. 12, pp. 146-153, 2024. Crossref, https://doi.org/10.14445/23488549/IJECE-V11I12P114
Abstract:
Forecasting early-stage student performance within higher education is important to the academic community, offering a proactive framework to mitigate student attrition. However, gauging and prognosticating students' achievements in the Indian context are beset by formidable challenges due to the vast student populace and the deeply entrenched educational system. Each institution in India employs distinct criteria to assess student progress, lacking a standardized mechanism to oversee and appraise developmental trajectories. The past decade has witnessed diverse exploration of machine learning methodologies in educational research. Nonetheless, student performance prediction grapples with substantial obstacles, particularly when contending with imbalanced datasets. This research work adopts a dual-phase methodology to grapple with this quandary. Initially, conventional classification algorithms are deployed on a dataset encompassing the academic journeys of 4424 students. Subsequently, innovative hybrid machine learning (ML) algorithms are harnessed to yield more refined prognostications. The outcomes furnished by the proposed model furnish a platform for informed early decision-making of the advancement of higher education institutions. This streamlines the prediction of students' performance and empowers the educational domain to tackle these challenges with a more robust and insightful approach.
Keywords:
Academic performance, Cross-validation, Artificial Intelligence, Hybrid ML algorithms.
References:
[1] Reynold A. Rustia et al., “Predicting Student's Board Examination Performance Using Classification Algorithms,” ICSCA '18: Proceedings of the 2018 7th International Conference on Software and Computer Applications, Kuantan, Malaysia, pp. 233-237, 2018.
[CrossRef] [Google Scholar] [Publisher Link]
[2] Hanan Abdullah Mengash, “Using Data Mining Techniques to Predict Student Performance to Support Decision Making in University Admission Systems,” IEEE Access, vol. 8, pp. 55462-55470, 2020.
[CrossRef] [Google Scholar] [Publisher Link]
[3] Siti Dianah Abdul Bujang et al., “Imbalanced Classification Methods for Student Grade Prediction: A Systematic Literature Review,” IEEE Access, vol. 11, pp. 1970-1989, 2023.
[CrossRef] [Google Scholar] [Publisher Link]
[4] Ramin Ghorbani, and Rouzbeh Ghousi, “Comparing Different Resampling Methods in Predicting Students’ Performance Using Machine Learning Techniques,” IEEE Access, vol. 8, pp. 67899-67911, 2020.
[CrossRef] [Google Scholar] [Publisher Link]
[5] Akhilesh P. Patil, Karthik Ganesan, and Anita Kanavalli, “Effective Deep Learning Model to Predict Student Grade Point Averages,” 2017 IEEE International Conference on Computational Intelligence and Computing Research (ICCIC), Coimbatore, India, pp. 1-6, 2017.
[CrossRef] [Google Scholar] [Publisher Link]
[6] Praveena Chakrapani, and D. Chitradevi, “Academic Performance Prediction Using Machine Learning: A Comprehensive & Systematic Review,” 2022 International Conference on Electronic Systems and Intelligent Computing (ICESIC), Chennai, India, pp. 335-340, 2022.
[CrossRef] [Google Scholar] [Publisher Link]
[7] Matloob Khushi et al., “A Comparative Performance Analysis of Data Resampling Methods on Imbalance Medical Data,” IEEE Access, vol. 9, pp. 109960-109975, 2021.
[CrossRef] [Google Scholar] [Publisher Link]
[8] Raza Hasan et al., “Predicting Student Performance in Higher Educational Institutions Using Video Learning Analytics and Data Mining Techniques,” Applied Sciences, vol. 10, no. 11, pp. 1-20, 2020.
[CrossRef] [Google Scholar] [Publisher Link]
[9] Christian Fischer et al., “Mining Big Data in Education: Affordances and Challenges,” Review of Research in Education, vol. 44, no. 1, pp. 130-160, 2020.
[CrossRef] [Google Scholar] [Publisher Link]
[10] Aishwarya Suresh, H.S. Sushma Rao, and Vinayak Hegde, “Academic Dashboard—Prediction of Institutional Student Dropout Numbers Using a Naive Bayesian Algorithm,” Computing and Network Sustainability, vol. 12, pp. 73-82, 2017.
[CrossRef] [Google Scholar] [Publisher Link]
[11] Ivan Nunes da Silva et al., Artificial Neural Network Architectures and Training Processes, Artificial Neural Networks : A Practical Course, Springer International Publishing, pp. 21-28, 2017.
[CrossRef] [Google Scholar] [Publisher Link]
[12] Valentim Realinho et al., “Predicting Student Dropout and Academic Success,” Data, vol. 7, no. 11, pp. 1-17, 2022.
[CrossRef] [Google Scholar] [Publisher Link]
[13] Juan D. Rodriguez, Aritz Perez, and Jose A. Lozano, “Sensitivity Analysis of k-Fold Cross Validation in Prediction Error Estimation,” IEEE Transactions on Pattern Analysis and Machine Intelligence, vol. 32, no. 3, pp. 569-575, 2010.
[CrossRef] [Google Scholar] [Publisher Link]
[14] Oleg Uzhga-Rebrov, and Peter Grabusts, “Comparative Evaluation of Four Methods for Exploratory Data Analysis,” 2021 62nd International Scientific Conference on Information Technology and Management Science of Riga Technical University (ITMS), Riga, Latvia, pp. 1-5, 2021.
[CrossRef] [Google Scholar] [Publisher Link]
[15] Shahzad Ali Khan, and Zeeshan Ali Rana “Evaluating Performance of Software Defect Prediction Models Using Area Under Precision-Recall Curve (AUC-PR),” 2019 2nd International Conference on Advancements in Computational Sciences (ICACS), Lahore, Pakistan, pp. 1-6, 2019.
[CrossRef] [Google Scholar] [Publisher Link]