Insights from Machine Learning Models: Sentiment Trends on X (Formerly Twitter)
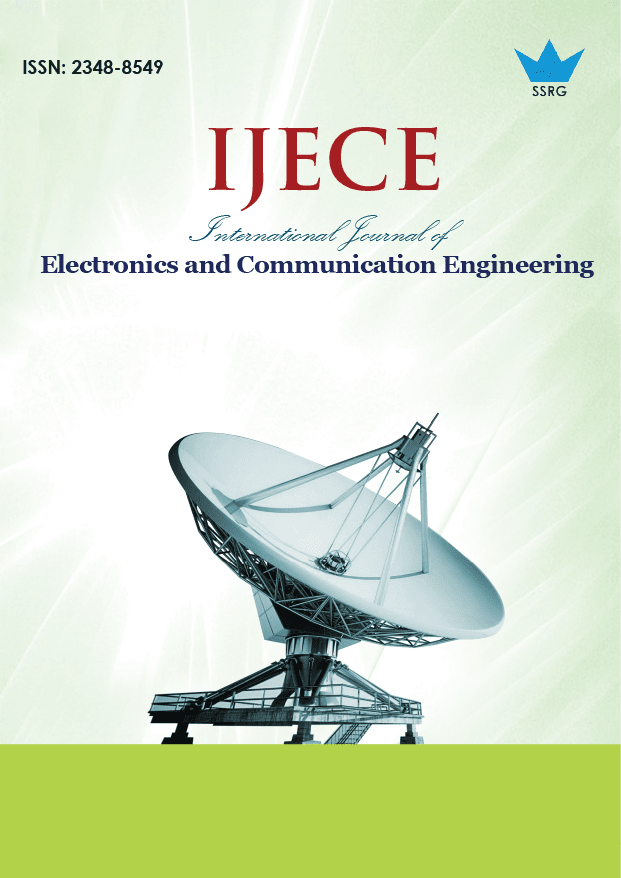
International Journal of Electronics and Communication Engineering |
© 2024 by SSRG - IJECE Journal |
Volume 11 Issue 12 |
Year of Publication : 2024 |
Authors : Poorva Agrawal, Charvi Kumar, Somesh Nagar, Saumya Deshmukh |
How to Cite?
Poorva Agrawal, Charvi Kumar, Somesh Nagar, Saumya Deshmukh, "Insights from Machine Learning Models: Sentiment Trends on X (Formerly Twitter)," SSRG International Journal of Electronics and Communication Engineering, vol. 11, no. 12, pp. 154-163, 2024. Crossref, https://doi.org/10.14445/23488549/IJECE-V11I12P115
Abstract:
X (formerly Twitter) has long been a platform that allows users to share their thoughts and beliefs and vent their more negative feelings on a plethora of subjects. In an age dominated by social media, where people online lay their emotions and opinions bare, the ability to utilize natural language processing methods to extract and assess sentiments from tweets has become crucial. Using machine learning models like Random Forest Classifier, Logistic Regression, and Naïve Bayes, which produced encouraging findings, the study technique includes data gathering, preprocessing, feature extraction, and sentiment categorization. After performing a thorough research of sentiment analysis of tweets, the paper delves into possible ramifications from a national security and surveillance perspective.
Keywords:
Social-media, Sentiments, Emotions, Machine learning, Analysis, Natural language processing, Random Forest, Naïve Bayes, Logistic Regression, National security, Surveillance, SDG 16.
References:
[1] Pinkesh Badjatiya et al., “Deep Learning For Hate Speech Detection In Tweets,” Proceedings of the 26th International Conference on World Wide Web Companion, Switzerland, pp. 759-760, 2017.
[CrossRef] [Google Scholar] [Publisher Link]
[2] Mohammad Ehsan Basiri et al., “ABCDM: An Attention-Based Bidirectional CNN-RNN Deep Model for Sentiment Analysis,” Future Generation Computer Systems, vol. 115, pp. 279-294, 2021.
[CrossRef] [Google Scholar] [Publisher Link]
[3] Zhao Jianqiang, Gui Xiaolin, and Zhang Xuejun, “Deep Convolution Neural Networks for Twitter Sentiment Analysis,” IEEE Access, vol. 6, pp. 23253-23260, 2018.
[CrossRef] [Google Scholar] [Publisher Link]
[4] Duyu Tang et al., “Learning Sentiment-Specific Word Embedding For Twitter Sentiment Classification,” Proceedings of the 52nd Annual Meeting of the Association for Computational Linguistics, Baltimore, Maryland, USA, vol. 1, pp. 1555-1565, 2014.
[CrossRef] [Google Scholar] [Publisher Link]
[5] Md Rakibul Hasan, Maisha Maliha, and M. Arifuzzaman, “Sentiment Analysis with NLP on Twitter Data,” 2019 International Conference on Computer, Communication, Chemical, Materials and Electronic Engineering (IC4ME2), Rajshahi, Bangladesh, pp. 1-4, 2019.
[CrossRef] [Google Scholar] [Publisher Link]
[6] Thavavel Vaiyapuri, “Sustainable Artificial Intelligence Based Twitter Sentiment Analysis on COVID-19 Pandemic,” Sustainability, vol. 15, no. 8, pp. 1-15, 2023.
[CrossRef] [Google Scholar] [Publisher Link]
[7] Ankit Kumar Soni, Multi-Lingual Sentiment Analysis of Twitter Data by Using Classification Algorithms, 2017 Second International Conference on Electrical, Computer and Communication Technologies (ICECCT), Coimbatore, India, pp. 1-5, 2017.
[CrossRef] [Google Scholar] [Publisher Link]
[8] Boppuru Rudra Prathap, and K. Ramesha, “Twitter Sentiment for Analysing Different Types of Crimes,” International Conference on Communication, Computing and Internet of Things (IC3IoT), Chennai, India, pp. 483-488, 2018.
[CrossRef] [Google Scholar] [Publisher Link]
[9] Manish Dhingra, and Rakesh K. Mudgal, “Historical Evolution of Social Media: An Overview,” International Conference on Advances in Engineering Science Management and Technology (ICAESMT), Uttaranchal University, Dehradun, India, pp. 1-8, 2019.
[CrossRef] [Google Scholar] [Publisher Link]
[10] Thomas Aichner et al., “Twenty-Five Years of Social Media: A Review of Social Media Applications and Definitions from 1994 to 2019,” Cyberpsychology, Behavior, and Social Networking, vol. 24, no. 4, pp. 215-222, 2021.
[CrossRef] [Google Scholar] [Publisher Link]
[11] C. Hutto, and Eric Gilbert, “Vader: A Parsimonious Rule-Based Model for Sentiment Analysis of Social Media Text,” Proceedings of the International AAAI Conference on Web and Social Media, vol. 8, no. 1, pp. 216-225, 2014.
[CrossRef] [Google Scholar] [Publisher Link]
[12] Simranpreet Kaur, Pallavi Kaul, and Pooya Moradian Zadeh, “Monitoring the Dynamics of Emotions During COVID-19 Using Twitter Data,” Procedia Computer Science, vol. 177, pp. 423-430, 2020.
[CrossRef] [Google Scholar] [Publisher Link]
[13] Ankita Sharma, and Udayan Ghose, “Sentimental Analysis of Twitter Data With Respect to General Elections in India,” Procedia Computer Science, vol. 173, pp. 325-334, 2020.
[CrossRef] [Google Scholar] [Publisher Link]
[14] Da Li et al., “HEMOS: A Novel Deep Learning-Based Fine-Grained Humor Detecting Method for Sentiment Analysis of Social Media,” Information Processing and Management, vol. 57, no. 6, 2020.
[CrossRef] [Google Scholar] [Publisher Link]
[15] Muhammad Asif et al., “Sentiment Analysis of Extremism in Social Media from Textual Information,” Telematics and Informatics, vol. 48, 2020.
[CrossRef] [Google Scholar] [Publisher Link]
[16] Malliga Subramanian et al., “A Survey On Hate Speech Detection And Sentiment Analysis Using Machine Learning And Deep Learning Models,” Alexandria Engineering Journal, vol. 80, pp. 110-121, 2023.
[CrossRef] [Google Scholar] [Publisher Link]
[17] Anisha P. Rodrigues et al., “Retracted:: Real-Time Twitter Spam Detection and Sentiment Analysis Using Machine Learning and Deep Learning Techniques,” Computational Intelligence and Neuroscience, vol. 2022, pp. 1-15, 2022.
[CrossRef] [Google Scholar] [Publisher Link]
[18] T.M. Saravanan et al., “Machine Learning Algorithm for Sentiment Analysis on Tweets,” 2nd International Conference on Automation, Computing and Renewable Systems (ICACRS), Pudukkottai, India, pp. 1-6, 2023.
[CrossRef] [Google Scholar] [Publisher Link]
[19] Boppuru Rudra Prathap, “Geospatial Crime Analysis and Forecasting with Machine Learning Techniques,” Artificial Intelligence and Machine Learning for EDGE Computing, pp. 87-102, 2022.
[CrossRef] [Google Scholar] [Publisher Link]
[20] Joan Donovan, “How Networked Incitement Fueled the January 6 Capitol Insurrection, Scientific American, 2024. [Online]. Available: https://www.scientificamerican.com/article/jan-6-was-an-example-of-networked-incitement/
[21] Loris Belcastro et al., “Analyzing Voter Behavior on Social Media During The 2020 US Presidential Election Campaign,” Social Network Analysis and Mining, vol. 12, no. 1, pp. 1-16, 2022.
[CrossRef] [Google Scholar] [Publisher Link]
[22] Livio Bioglio, and Ruggero G. Pensa, “Analysis and Classification of Privacy-Sensitive Content in Social Media Posts,” EPJ Data Science, vol. 11, no. 1, pp. 1-24, 2022.
[CrossRef] [Google Scholar] [Publisher Link]
[23] Shinigami, Sentimental Analysis for Tweets, Kaggle, 2021. [Online]. Available: https://www.kaggle.com/datasets/gargmanas/sentimental-analysis-for-tweets
[24] Passionate-Nlp, Twitter - Entity Sentiment Analysis, Kaggle, 2021. [Online]. Available: https://www.kaggle.com/datasets/jp797498e/twitter-entity-sentiment-analysis/data
[25] Ali Toosi, Twitter Sentiment Analysis, Kaggle, 2019. [Online]. Available: https://www.kaggle.com/datasets/arkhoshghalb/twitter-sentiment-analysis-hatred-speech