A Novel Feature Extraction Classifier for Hateful, Offense, and Neutral Content on X
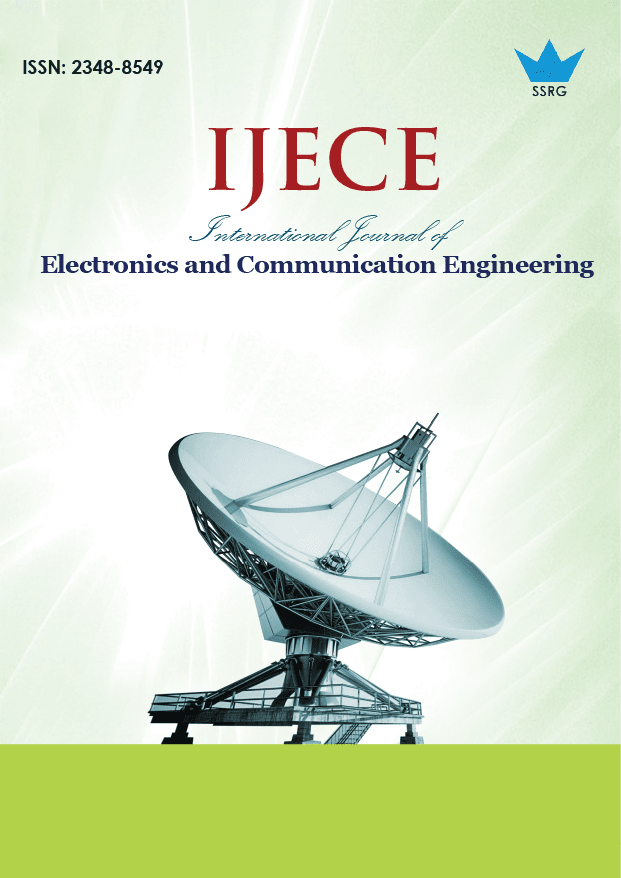
International Journal of Electronics and Communication Engineering |
© 2024 by SSRG - IJECE Journal |
Volume 11 Issue 12 |
Year of Publication : 2024 |
Authors : Anjani Kumar |
How to Cite?
Anjani Kumar, "A Novel Feature Extraction Classifier for Hateful, Offense, and Neutral Content on X," SSRG International Journal of Electronics and Communication Engineering, vol. 11, no. 12, pp. 164-170, 2024. Crossref, https://doi.org/10.14445/23488549/IJECE-V11I12P116
Abstract:
With the rapid growth of online social networks, content censorship remains controversial, dividing people into two groups, one supporting hateful content and the other supporting neutral content. This paper addresses the problem of classifying a tweet as hateful, offensive, or neutral content, which uses Term Frequency Inverse Document Frequencies (TFIDFs) for feature extraction. It uses the X dataset to train the proposed classifier model, and the results show that Gaussian Naive Bayes is the best-performing model after hyperparameter tuning of TFIDF features.
Keywords:
IDF, X, Hate speech, Offensive, Data cleaning.
References:
[1] Pete Burnap, and Matthew L. Williams, “Cyber Hate Speech on Twitter: An Application of Machine Classification and Statistical Modeling for Policy and Decision Making,” Policy and Internet, vol. 7, no. 2, pp. 223-242, 2015.
[CrossRef] [Google Scholar] [Publisher Link]
[2] Hajime Watanabe, Mondher Bouazizi, and Tomoaki Ohtsuki, “Hate Speech on Twitter: A Pragmatic Approach to Collect Hateful and Offensive Expressions and Perform Hate Speech Detection,” IEEE Access, vol. 6, pp. 13825-13835, 2018.
[CrossRef] [Google Scholar] [Publisher Link]
[3] William Warner, and Julia Hirschberg, “Detecting Hate Speech on the World Wide Web,” Proceedings of the 2012 Workshop on Language in Social Media (LSM 2012), Montreal, Canada, pp. 19-26, 2012.
[Google Scholar] [Publisher Link]
[4] Nemanja Djuric et al., “Hate Speech Detection with Comment Embeddings,” WWW '15 Companion: Proceedings of the 24th International Conference on World Wide Web, New York, United States, pp. 29-30, 2015.
[CrossRef] [Google Scholar] [Publisher Link]
[5] Georgios K. Pitsilis, Heri Ramampiaro, and Helge Langseth, “Effective Hate-Speech Detection in Twitter Data Using Recurrent Neural Networks,” Applied Intelligence, vol. 48, no. 12, pp. 4730-4742, 2018.
[CrossRef] [Google Scholar] [Publisher Link]
[6] Njagi Dennis Gitari et al., “A Lexicon-Based Approach for Hate Speech Detection,” International Journal of Multimedia and Ubiquitous Engineering, vol. 10, no. 4, pp. 215-230, 2015.
[CrossRef] [Google Scholar] [Publisher Link]
[7] Chikashi Nobata et al., “Abusive Language Detection in Online User Content,” Proceedings of the 25th International Conference on World Wide Web, Switzerland, pp. 145-153, 2016.
[CrossRef] [Google Scholar] [Publisher Link]
[8] Irene Kwok, and Yuzhou Wang, “Locate the Hate: Detecting Tweets Against Blacks,” In Proceedings of the AAAI Conference on Artificial Intelligence, vol. 27, no. 1, pp. 1621-1622, 2013.
[CrossRef] [Google Scholar] [Publisher Link]
[9] Garima Koushik, K. Rajeswari, and Suresh Kannan Muthusamy, “Automated Hate Speech Detection on Twitter,” 2019 5th International Conference on Computing, Communication, Control and Automation (ICCUBEA), Pune, India, pp. 1-4, 2019.
[CrossRef] [Google Scholar] [Publisher Link]
[10] Tommi Gröndahl et al., “All You Need is “love”: Evading Hate Speech Detection,” AISec '18: Proceedings of the 11th ACM Workshop on Artificial Intelligence and Security, New York, United States, pp. 2-12, 2018.
[CrossRef] [Google Scholar] [Publisher Link]
[11] Ziqi Zhang, David Robinson, and Jonathan Tepper, “Detecting Hate Speech on Twitter Using a Convolution-GRU Based Deep Neural Network,” The Semantic Web: 15th International Conference, ESWC 2018, Heraklion, Crete, Greece, vol. 10843, pp 745-760, 2018.
[CrossRef] [Google Scholar] [Publisher Link]
[12] Wenjie Yin, and Arkaitz Zubiaga, “Towards Generalisable Hate Speech Detection: A Review on Obstacles and Solutions,” PeerJ Computer Science, vol. 7, pp. 1-38, 2021.
[CrossRef] [Google Scholar] [Publisher Link]
[13] Dan Nelson, Gradient Boosting Classifiers in Python with Scikit-Learn, Stack Abuse, 2023, [Online]. Available: https://stackabuse.com/gradient-boosting-classifiers-in-python-with-scikit-learn/
[14] Hemant Kumar Soni, Sanjiv Sharma, and G. R. Sinha, Text and Social Media Analytics for Fake News and Hate Speech Detection, 1st ed., A Chapman and Hall Book, CRC Press, pp. 1-324, 2024.
[Google Scholar] [Publisher Link]
[15] Supriya Raheja, “Analysis of Psychological Distress during COVID-19 among Professionals,” International Journal of Software Innovation (IJSI), vol. 10, no. 1, pp.1-17, 2022.
[CrossRef] [Google Scholar] [Publisher Link]
[16] Anjum, and Rahul Katarya, “Hate Speech, Toxicity Detection in Online Social Media: A Recent Survey of State of The Art and Opportunities,” International Journal of Information Security, vol. 23, pp. 577-608, 2024.
[CrossRef] [Google Scholar] [Publisher Link]
[17] Patil Sushma, “Text Mining - A Comparative Review of Twitter Sentiments Analysis,” Recent Advances in Computer Science and Communications, vol. 17, no. 1, pp. 21-37, 2024.
[CrossRef] [Google Scholar] [Publisher Link]
[18] Chenxuan Yang et al., “Timothy Barnett, Analysis of First Responder-Involved Traffic Incidents By Mining News Reports,” Accident Analysis & Prevention, vol. 192, 2023.
[CrossRef] [Google Scholar] [Publisher Link]
[19] Abdullah Havolli, Arianit Maraj and Lorik Fetahu, ”Building A Content-Based Recommendation Engine Model Using Adamic Adar Measure; A Netflix Case Study,” 11th Mediterranean Conference on Embedded Computing (MECO), Budva, Montenegro, pp. 1-8, 2022.
[CrossRef] [Google Scholar] [Publisher Link]
[20] Pinkesh Badjatiya et al., “Deep Learning for Hate Speech Detection in Tweets,” WWW '17 Companion: Proceedings of the 26th International Conference on World Wide Web Companion, Switzerland, pp. 759-760, 2017.
[CrossRef] [Google Scholar] [Publisher Link]