Revolutionizing Bone Fracture Diagnosis: A Deep Learning Approach to X-Ray Image Analysis
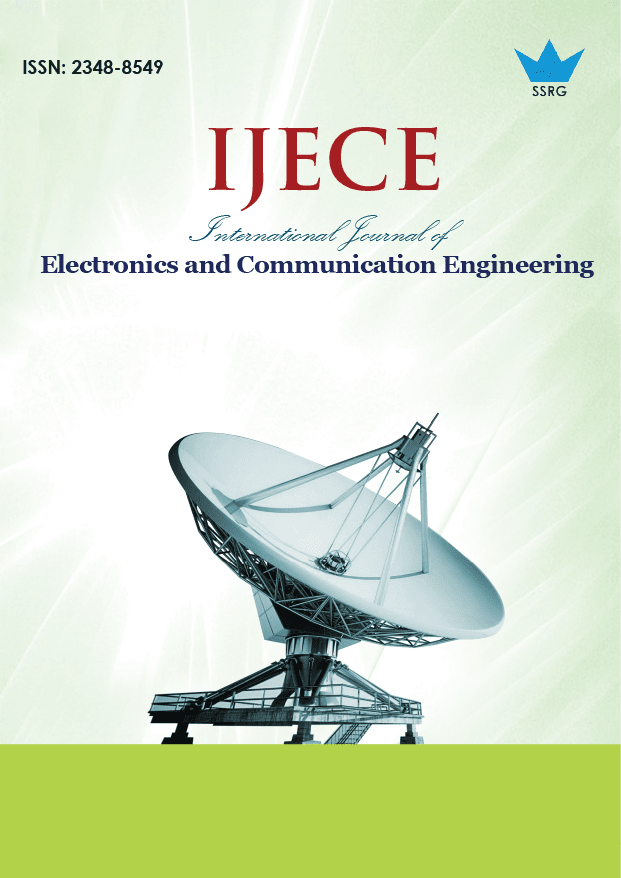
International Journal of Electronics and Communication Engineering |
© 2024 by SSRG - IJECE Journal |
Volume 11 Issue 12 |
Year of Publication : 2024 |
Authors : A. M. Linchu, B. Ben Sujitha |
How to Cite?
A. M. Linchu, B. Ben Sujitha, "Revolutionizing Bone Fracture Diagnosis: A Deep Learning Approach to X-Ray Image Analysis," SSRG International Journal of Electronics and Communication Engineering, vol. 11, no. 12, pp. 219-229, 2024. Crossref, https://doi.org/10.14445/23488549/IJECE-V11I12P120
Abstract:
Bones are the very important part of the human body, which provides body structure and protection for the internal organs. A bone fracture is a widespread scenario for the human body, which can lead to serious complications. Misdiagnosis of fractures is the most common mistake, resulting in treatment delays and permanent impairment. So, timely and accurate fracture detection is critical for proper treatment planning and medical diagnosis. X-ray imaging is a widely used diagnostic tool since manual interpretation is prone to errors. This study proposes an AI enhanced bone detection framework utilizing a bone fracture dataset consisting of 9463 X-ray images of fractured and non-fractured cases. Different preprocessing and data augmentation techniques played a major role in improving the dataset diversity and generalizability. The proposed methodology employs ResNet 50 for the feature extraction, enhancing it with the Bottleneck Attention Module (BAM) with dual attention strategies to refine critical features for effective fracture detection. With an accuracy of 97%, 96.12% precision, recall of 96.70%, and 96.38% F1 score, the suggested model outperformed other models like YOLOv8, Ensemble Model, ResNet50-DenseNet 121, and CNN. The results demonstrated that with improved feature representation and accuracy in bone fracture detection, the proposed model exhibits a valuable tool for enhanced patient care through early intervention and accurate fracture diagnosis.
Keywords:
Bone fracture, X-ray images, Medical diagnosis, Deep learning, Attention mechanism.
References:
[1] Christopher Jerome, Benjamin Hoch, and Cathy S. Carlson, 5 - Skeletal System, Comparative Anatomy and Histology: A Mouse, Rat, and Human Atlas, 2nd ed, Academic Press, pp. 67-88, 2018.
[CrossRef] [Google Scholar] [Publisher Link]
[2] Vassiliki Tzelepi et al., Bone Anatomy, Physiology and Function, Bone Metastases: A Translational and Clinical Approach, 1st ed, Springer Netherlands, pp. 3-30. 2009.
[CrossRef] [Google Scholar] [Publisher Link]
[3] N. L. Fazzalari, “Bone Fracture and Bone Fracture Repair,” Osteoporosis International, vol. 22, pp. 2003-2006, 2011.
[CrossRef] [Google Scholar] [Publisher Link]
[4] Nancy Johari, and Natthan Singh, “Bone Fracture Detection Using Edge Detection Technique”, Soft Computing: Theories and Applications: Proceedings of SoCTA, Springer Singapore, vol. 2, pp. 11-19, 2018.
[CrossRef] [Google Scholar] [Publisher Link]
[5] Dhahir Basim Mohammed, Hameed Imad Hadi, and Jaber Ahmed Radee, “Prospective and Retrospective Study of Fractures According to Trauma Mechanism and Type of Bone Fracture,” Research Journal of Pharmacy and Technology, vol. 10, no. 11, pp. 3810-3818, 2017.
[CrossRef] [Google Scholar] [Publisher Link]
[6] Muhammet Emin Sahin, “Image Processing and Machine Learning‐Based Bone Fracture Detection and Classification using X‐Ray Images,” International Journal of Imaging Systems and Technology, vol. 33, no. 3, pp. 853-865, 2023.
[CrossRef] [Google Scholar] [Publisher Link]
[7] Amal Alshahrani, and Alaa Alsairafi, “Bone Fracture Classification Using Convolutional Neural Networks from X-Ray Images,” Engineering, Technology & Applied Science Research, vol. 14, no. 5, pp. 16640-16645, 2024.
[CrossRef] [Google Scholar] [Publisher Link]
[8] A. Tahir et al., “Enhancing Diagnosis: Ensemble Deep Learning Model for Fracture Detection Using X-Ray Images,” Clinical Radiology, vol. 79, no. 11, pp. e1394-e1402, 2024.
[CrossRef] [Google Scholar] [Publisher Link]
[9] M Abhijeeth Thaarakaraam, M Manideep Reddy, and Vaidehi Vijayakumar, “CNN Based Bone Fracture Detection for Medical Imaging Using Resnet-50, International Journal of Technical Research & Science, pp. 216-22, 2024.
[Google Scholar] [Publisher Link]
[10] Yangling Ma, and Yixin Luo, “Bone Fracture Detection through the Two-Stage System of Crack-Sensitive Convolutional Neural Network,” Informatics in Medicine Unlocked, vol. 22, 2021.
[CrossRef] [Google Scholar] [Publisher Link]
[11] Mengxuan Wang et al., “Parallelnet: Multiple Backbone Network for Detection Tasks on Thigh Bone Fracture,” Multimedia Systems, vol. 27, pp. 1091-1100, 2021.
[CrossRef] [Google Scholar] [Publisher Link]
[12] Shahnaj Parvin, and Abdur Rahman, “A Real-Time Human Bone Fracture Detection and Classification from Multi-Modal Images Using Deep Learning Technique,” Applied Intelligence, vol. 54, pp. 9269-9285, 2024.
[CrossRef] [Google Scholar] [Publisher Link]
[13] Aymen Saad, Usman Ullah Sheikh, and Mortada Sabri Moslim, “Developing Convolutional Neural Network for Recognition of Bone Fractures in X-ray Images,” Advances in Science and Technology Research Journal, vol. 18, no, 4, pp. 228-237, 2024.
[CrossRef] [Google Scholar] [Publisher Link]
[14] Puja Dey et al., “Hybrid Deep Transfer Learning Framework for Humerus Fracture Detection and Classification from X-ray Images,” 2024 4th International Conference on Intelligent Technologies (CONIT), Bangalore, India, pp. 1-6, 2024.
[CrossRef] [Google Scholar] [Publisher Link]
[15] Sai Charan Medaramatla et al., “Detection of Hand Bone Fractures in X-ray Images Using Hybrid Yolo Nas,” IEEE Access, vol. 12, no. 57661-57673, 2024.
[CrossRef] [Google Scholar] [Publisher Link]
[16] Santoshachandra Rao Karanam, Y. Srinivas, and S. Chakravarty, “A Supervised Approach to Musculoskeletal Imaging Fracture Detection and Classification Using Deep Learning Algorithms,” Computer Assisted Methods in Engineering and Science, vol. 30, no. 3, pp. 369-385, 2023.
[CrossRef] [Google Scholar] [Publisher Link]
[17] Mohamed A. Kassem et al., “Explainable Transfer Learning‐Based Deep Learning Model for Pelvis Fracture Detection,” International Journal of Intelligent Systems, vol. 2023, no. 1, pp. 1-10, 2023.
[CrossRef] [Google Scholar] [Publisher Link]
[18] Madona B. Sahaai et al., “ResNet-50 Based Deep Neural Network Using Transfer Learning for Brain Tumor Classification,” AIP Conference Proceedings, vol. 2463, no. 1, 2022.
[CrossRef] [Google Scholar] [Publisher Link]
[19] Kosrat Dlshad Ahmed, and Roojwan Hawezi, “Detection of Bone Fracture Based on Machine Learning Techniques,” Measurement: Sensors, vol. 27, pp. 1-6, 2023.
[CrossRef] [Google Scholar] [Publisher Link]
[20] Brett Koonce, Convolutional Neural Networks with Swift for Tensorflow: Image Recognition and Dataset Categorization, Apress, pp. 1-245, 2021.
[CrossRef] [Google Scholar] [Publisher Link]
[21] Fanyi Wang, Haotian Hu, and Cheng Shen, “BAM: A Balanced Attention Mechanism for Single Image Super Resolution,” Electrical Engineering and Systems Science: Image and Video Processing, arXiv, pp. 1-8, 2021.
[CrossRef] [Google Scholar] [Publisher Link]