Modelling and Analysis of Magnitude-Squared Wavelet Coherence data for Biomedical Applications
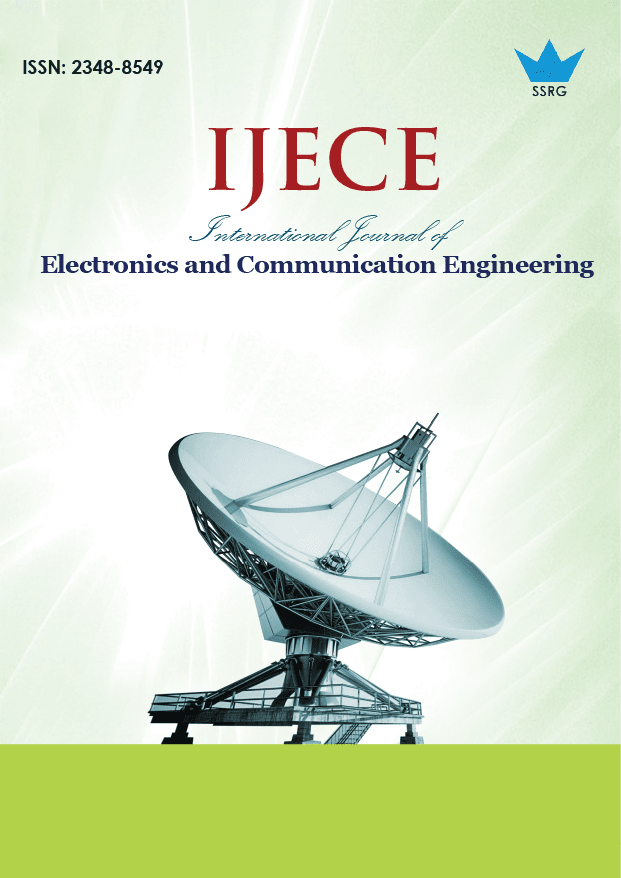
International Journal of Electronics and Communication Engineering |
© 2025 by SSRG - IJECE Journal |
Volume 12 Issue 1 |
Year of Publication : 2025 |
Authors : Polepogu Rajesh, Vaegae Naveen Kumar |
How to Cite?
Polepogu Rajesh, Vaegae Naveen Kumar, "Modelling and Analysis of Magnitude-Squared Wavelet Coherence data for Biomedical Applications," SSRG International Journal of Electronics and Communication Engineering, vol. 12, no. 1, pp. 14-32, 2025. Crossref, https://doi.org/10.14445/23488549/IJECE-V12I1P102
Abstract:
One of the most persistent neurological diseases is epileptic seizures, which have an impact on people’s daily lives by endangering them with frequent seizures. In recent years, seizure detection techniques have been categorized into a number of groups, mainly rational function, empirical mode decomposition, wavelet (time-frequency), time, and frequency. Current diagnostic techniques have focused on developing techniques for electrocardiograms (ECGs) and electroencephalograms (EEGs) due to their noninvasiveness and their capacity to provide repetitive patterns of epileptic-related electrical information. In this work, Power Spectrum Density Estimation (PSDE) is used to examine the magnitude squared wavelet coherence (MSWC) between the ECG and EEG during an epileptic seizure in the typical frequency range of 0–128 Hz. The datasets used in this work are PhysioNet and “N&C-TEC: ECG and EEG files”. Mendeley data are used to collect all signals from epileptic seizure patients aged 3 to 25 at Children’s Hospital Boston at a sampling rate of 512 samples/second. Each signal is comprised of 30 seconds (15,360 samples). The mean values of the MSWC between the ECG and EEG are calculated. According to the measurement, the MSC values between the ECG and EEG signals in epileptic seizure sample-1 are 0.05, 0.1, and 0.4. Similarly, in epileptic seizure sample-2, MSC values are 0.4 and 0.55; in sample-3, MSC values are 0.15 and 0.4, respectively, in the frequency range of 0 to 128 Hz. Finally, the mean of the MSC value between the ECG and EEG for the first sample, second sample, third sample, and fourth sample is less than 0.55. It shows that the coherence value between the circulatory system and the central nervous system is diminished in epileptic seizure patients, i.e., the coherence between the heart and brain is very low in seizure patients.
Keywords:
Electrocardiogram (ECG), Electroencephalogram (EEG), Heart Rate Variability (HRV), Fast Fourier Transform (FFT), Morlet Wavelet, Magnitude Squared Wavelet Coherence (MSWC), Seizures, Epilepsy.
References:
[1] Epilepsy, World Health Organization, 2024. [Online]. Available: https://www.who.int/news-room/fact-sheets/detail/epilepsy
[2] Mary Jane England et al., “Epilepsy Across the Spectrum: Promoting Health and Understanding-A Summary of the Institute of Medicine Report,” Epilepsy & Behavior, vol. 25, no. 2, pp. 266-276, 2012.
[CrossRef] [Google Scholar] [Publisher Link]
[3] Ashok Sharmila, and Purusothaman Geethanjali, “A Review on the Pattern Detection Methods for Epilepsy Seizure Detection from EEG Signals,” Biomedical Engineering, vol. 64, no. 5, pp. 507-517, 2019.
[CrossRef] [Google Scholar] [Publisher Link]
[4] Turky N. Alotaiby et al., “Channel Selection and Seizure Detection using a Statistical Approach,” 2016 5th International Conference on Electronic Devices, Systems and Applications (ICEDSA), Ras Al Khaimah, United Arab Emirates, pp. 1-4, 2016.
[CrossRef] [Google Scholar] [Publisher Link]
[5] Rashmi Anupozu, and Rama Bobba, “Epilepsy – A Comprehensive Review,” International Journal of Pharma Research, vol. 8, no. 11, pp. 375-391, 2019.
[CrossRef] [Google Scholar] [Publisher Link]
[6] P.A. Dekker, “Epilepsy: A Manual for Medical and Clinical Officers,” World Health Organization, pp. 1-124, 2002.
[Google Scholar] [Publisher Link]
[7] Andrea Varsavsky, Iven Mareels, and Mark Cook, Epileptic Seizures and the EEG, Measurement, Models, Detection, and Prediction, CRC Press, pp. 1-370, 2010.
[CrossRef] [Google Scholar] [Publisher Link]
[8] Jerome Engel, Seizures and Epilepsy, Oxford University Press, pp. 1-706, 2013.
[Google Scholar] [Publisher Link]
[9] Zulfi Haneefand, and Atul Maheshwari, A Concise Manual of Epilepsy, Createspace Independent Publishing, pp. 1-182, 2016.
[Publisher Link]
[10] Hirak Kumar Mukhopadhyay et al., “Epilepsy and Its Management: A Review,” Journal of Pharma Science Technology, vol. 1, no. 2, pp. 20-26, 2012.
[Google Scholar]
[11] Hae Won Shin et al., “Review of Epilepsy - Etiology, Diagnostic Evaluation and Treatment,” International Journal of Neurorehabilitation, vol. 1, no. 3, 2014.
[CrossRef] [Google Scholar] [Publisher Link]
[12] Senthil Amudhan, Gopalkrishna Gururaj, and Parthasarathy Satishchandra, “Epilepsy in India, I: Epidemiology in Public Health,” Annals of Indian Academy of Neurology, vol. 18, no. 3, pp. 263–277, 2015.
[CrossRef] [Google Scholar] [Publisher Link]
[13] Anouk Van de Vel et al., “Non-EEG Seizure Detection Systems and Potential SUDEP Prevention: State of the Art,” Seizure, vol. 22, no. 5, pp. 345–355, 2013.
[CrossRef] [Google Scholar] [Publisher Link]
[14] Sriram Ramgopal et al., “Seizure Detection, Seizure Prediction, and Closed Loop Warning Systems in Epilepsy,” Epilepsy and Behavior, vol. 37, pp. 291-307, 2014.
[CrossRef] [Google Scholar] [Publisher Link]
[15] Sigge N. Larsen et al., “Detection of Tonic Epileptic Seizures Based on Surface Electromyography,” 2014 36th Annual International Conference of the IEEE Engineering in Medicine and Biology Society, Chicago, IL, USA, pp. 942-945, 2014.
[CrossRef] [Google Scholar] [Publisher Link]
[16] Tamara M. Nijsen et al., “Automated Detection of Tonic Seizures using 3-D Accelerometry,” 4th Europe an Conference of the International Federation for Medical and Biological Engineering, Antwerp, Belgium, pp. 188-191, 2009.
[CrossRef] [Google Scholar] [Publisher Link]
[17] Diana Cogan et al., “Multi-bio Signal Analysis for Epileptic Seizure Monitoring,” International Journal of Neural Systems, vol. 27, no.1, 2017.
[CrossRef] [Google Scholar] [Publisher Link]
[18] L.D. Blumhardt, P.E.M. Smith, and Lynne Owen, “Electrocardiographic Accompaniments of Temporal Lobe Epileptic Seizures,” Lancet Journal, vol. 327, no. 8489, pp. 1051-1056, 1986.
[CrossRef] [Google Scholar] [Publisher Link]
[19] Jesper Jeppesen et al., “Seizure Detection based on Heart Rate Variability using a Wearable Electrocardiography Device,” Epilepsia, vol. 60, no. 10, pp. 2105-2113, 2019.
[CrossRef] [Google Scholar] [Publisher Link]
[20] P.E.M. Smith et al., “Profiles of Instant Heart Rate during Partial Seizures,” Electroencephalography and Clinical Neurophysiology, vol. 72, no. 3, pp. 207-217, 1989.
[CrossRef] [Google Scholar] [Publisher Link]
[21] Athi Ponnusamy, Jefferson L.B. Marques, and Markus Reuber, “Comparison of Heart Rate Variability Parameters during Complex Partial Seizures and Psychogenic Nonepileptic Seizures,” Epilepsia, vol. 53, no. 8, pp. 1314-1321, 2012.
[CrossRef] [Google Scholar] [Publisher Link]
[22] Yuedong Song, “A Review of Developments of EEG-Based Automatic Medical Support Systems for Epilepsy Diagnosis and Seizure Detection,” Journal of Biomedical Science and Engineering, vol. 4, pp. 788-796, 2011.
[Google Scholar] [Publisher Link]
[23] Alexandros T. Tzallas et al., “Automated Epileptic Seizure Detection Methods: A Review Study,” Epilepsy-Histological, Electroencephalographic and Psychological Aspects, pp. 75-98, 2012.
[CrossRef] [Google Scholar] [Publisher Link]
[24] Ramendra Nath Bairagi, “Epileptic Seizure Identification in EEG Signals using DWT, ANN and Sequential Window Algorithm,” Soft Computing Letters, vol. 3, pp. 1-10, 2021.
[CrossRef] [Google Scholar] [Publisher Link]
[25] WorldPress Without Limits. [Online]. Available: https://wordpress.com/
[26] P. Kidmose, D. Looney, and D.P. Mandic, “Auditory Evoked Responses from Ear-EEG Recordings,” 2012 Annual International Conference of the IEEE Engineering in Medicine and Biology Society, San Diego, CA, USA, 2012.
[CrossRef] [Google Scholar] [Publisher Link]
[27] Kaare B. Mikkelsen et al., “EEG Recorded from the Ear: Characterizing the Ear-EEG Method,” Neuroscience, vol. 9, pp. 1-8, 2015.
[CrossRef] [Google Scholar] [Publisher Link]
[28] Evy Cleeren et al., “Comparison between Scalp EEG and Behind-the-Ear EEG for Development of a Wearable Seizure Detection System for Patients with Focal Epilepsy,” Sensors, vol. 18, no. 1, 2018.
[CrossRef] [Google Scholar] [Publisher Link]
[29] S.S. Papacostas, “Sudden Unexpected Death in Epilepsy: Experience from a Tertiary Epilepsy Centre in Cyprus with Review of the Literature,” Hippokratia, vol. 19, no. 4, pp. 338-343, 2015.
[Google Scholar] [Publisher Link]
[30] Iosif Mporas et al., “Seizure Detection using EEG and ECG Signals for Computer-based Monitoring, Analysis and Management of Epileptic Patients,” Expert Systems with Applications, vol. 42, no. 6, pp. 3227-3233, 2015.
[CrossRef] [Google Scholar] [Publisher Link]
[31] J. Gotman, “Automatic Recognition of Epileptic Seizures in the EEG,” Electroencephalography and Clinical Neurophysiology, vol. 54, no. 5, pp. 530-540, 1982.
[CrossRef] [Google Scholar] [Publisher Link]
[32] S. Nasehi, and H. Pourghassem, “Seizure Detection Algorithms Based on Analysis of EEG and ECG Signals: A Survey,” Neurophysiology, vol. 44, pp. 174-186, 2012.
[CrossRef] [Google Scholar] [Publisher Link]
[33] Md. Nazmus Sahadat, S. Consul-Pacareu, and Bashir I. Morshed, “Wireless Ambulatory ECG Signal Capture for HRV and Cognitive Load Study using the Neuro Monitor Platform,” 2013 6th Annual International IEEE EMBS Conference on Neural Engineering (NER), San Diego, CA, USA, pp. 497-500, 2013.
[CrossRef] [Google Scholar] [Publisher Link]
[34] E. Tessy, P.P. Muhammed Shanir, and Shaleena Manafuddin, “Time Domain Analysis of Epileptic EEG for Seizure Detection,” 2016 International Conference on Next Generation Intelligent Systems (ICNGIS), Kottayam, India, pp. 1-4, 2016. [CrossRef] [Google Scholar] [Publisher Link]
[35] Thomas De Cooman et al., “Online Detection of Tonic-Clonic Seizures in Pediatric Patients using ECG and Low-Complexity Incremental Novelty Detection,” 2015 37th Annual International Conference of the IEEE Engineering in Medicine and Biology Society (EMBC), Milan, Italy, pp. 5597-5600, 2015.
[CrossRef] [Google Scholar] [Publisher Link]
[36] A. Asuvaran, and G. Elatharasan, “Analysis of ECG and EEG Signals to Detect Epileptic Seizures,” International Journal of Engineering Research & Technology (IJERT), vol. 4, no. 24, pp. 1-4, 2018.
[CrossRef] [Publisher Link]
[37] Md Mursalina et al., “Automated Epileptic Seizure Detection using Improved Correlation-based Feature Selection with Random Forest Classifier,” Neurocomputing, vol. 241, pp. 204-214, 2017.
[CrossRef] [Google Scholar] [Publisher Link]
[38] Ashwini D. Bhople, and P.A. Tijare, “Fast Fourier Transform based Classification of Epileptic Seizure using Artificial Neural Network,” International Journal of Advanced Research Computer Science Software Engineering, vol. 2, no. 4, 2012.
[Google Scholar]
[39] Narendra Kumar Ambulkar, and S.N. Sharma, “Detection of Epileptic Seizure in EEG Signals using Window Width Optimized S-Transform and Artificial Neural Networks,” 2015 IEEE Bombay Section Symposium (IBSS), Mumbai, India, 2015.
[CrossRef] [Google Scholar] [Publisher Link]
[40] Maeike Zijlmans, Danny Flanagan, and Jean Gotman, “Heart Rate Changes and ECG Abnormalities during Epileptic Seizures: Prevalence and Definition of an Objective Clinical Sign,” Epilepsia, vol. 43, no. 8, pp. 847-854, 2002.
[CrossRef] [Google Scholar] [Publisher Link]
[41] Jing Li, “Digital Signal Characterization for Seizure Detection using Frequency Domain Analysis,” Student thesis, School of Electrical Engineering and Computer Science, pp. 1-63, 2021.
[Google Scholar] [Publisher Link]
[42] Nastaran Darjani, and Hesam Omranpour, “Phase Space Elliptic Density Feature for Epileptic EEG Signals Classification using Metaheuristic Optimization Method,” Knowledge-Based Systems, vol. 205, 2020.
[CrossRef] [Google Scholar] [Publisher Link]
[43] Yang Li et al., “Epileptic Seizure Detection in EEG Signals using Sparse Multiscale Radial Basis Function Networks and the Fisher Vector Approach,” Knowledge-Based Systems, vol. 164, pp. 96-106, 2019.
[CrossRef] [Google Scholar] [Publisher Link]
[44] Sourabh Banik et al., “Assessment of EEG-PPG Cross Frequency Coherence under Evoked Emotional Arousal,” Current Directions in Biomedical Engineering, vol. 10, no. 4, pp. 49-52, 2024.
[CrossRef] [Google Scholar] [Publisher Link]
[45] Hasan Polat, and Mehmet Sirac Ozerdem, “Epileptic Seizure Detection from EEG Signals by Using Wavelet and Hilbert Transform,” 2016 XII International Conference on Perspective Technologies and Methods in MEMS Design (MEMSTECH), Lviv, Ukraine, 2016.
[CrossRef] [Google Scholar] [Publisher Link]
[46] Manish Sharma, Ankit A. Bhurane, and U. Rajendra Acharya, “MMSFL-OWFA: A Novel Class of Orthogonal Wavelet Filters for Epileptic Seizure Detection,” Knowledge-Based Systems, vol. 160, pp. 265-277, 2018.
[CrossRef] [Google Scholar] [Publisher Link]
[47] Anubha Gupta, Pushpendra Singh, and Mandar Karlekar, “A Novel Signal Modeling Approach for Classification of Seizure and Seizure-free EEG Signals,” IEEE Transactions on Neural Systems and Rehabilitation Engineering, vol. 26, no. 5, pp. 925-935, 2018.
[CrossRef] [Google Scholar] [Publisher Link]
[48] S. Hadiyoso, I.D. Irawati, and A. Rizal, “Epileptic Electroencephalogram Classification using Relative Wavelet Sub-band Energy and Wavelet Entropy,” International Journal of Engineering, vol. 34, no. 1, pp. 75-81, 2021.
[CrossRef] [Google Scholar] [Publisher Link]
[49] R.G. Andrzejak, The Bonn EEG Time Series Download Page. [Online]. Available: https://www.upf.edu/web/ntsa/downloads/-/asset_publisher/xvT6E4pczrBw/content/2001-indications-of-nonlinear-deterministic-and-finite-dimensional-structures-in-time-series-of-brain-electrical-activity-dependence-on-recording-regi?inheritRedirect=false&redir
[50] Zarita Zainuddin, Lai Kee Huong, and Ong Pauline, “On the use of Wavelet Neural Networks in the Task of Epileptic Seizure Detection from Electroencephalography Signals,” Procedia Computer Science, vol. 11, pp. 149-159, 2012.
[CrossRef] [Google Scholar] [Publisher Link]
[51] Rezvan Abbasi, and Mansour Esmaeilpour, “Selecting Statistical Characteristics of Brain Signals to Detect Epileptic Seizures using Discrete Wavelet Transform and Perceptron Neural Network,” International Journal of Interactive Multimedia Artificial Intellegence, vol. 4, no. 5, pp. 33-38, 2017.
[CrossRef] [Google Scholar] [Publisher Link]
[52] R. Panda et al., “Classification of EEG signal using Wavelet Transform and Support Vector Machine for Epileptic Seizure Diction,” International Conference on Systems in Medicine and Biology, Kharagpur, India, pp. 405-408, 2010.
[CrossRef] [Google Scholar] [Publisher Link]
[53] G.R. Kiranmayi, and V. Udayashankara, “Detection of Epilepsy using Discrete Cosine Harmonic Wavelet Transform-based Features and Neural Network Classifier,” International Journal of Biomedical Engineering and Technology, vol. 32, no. 2, pp. 109-122, 2020.
[CrossRef] [Google Scholar] [Publisher Link]
[54] Muhammad Bilal Qureshi et al., “Machine Learning-based EEG Signals Classification Model for Epileptic Seizure Detection,” Multimedia Tools and Applications, vol. 80, pp. 17849-17877, 2021.
[CrossRef] [Google Scholar] [Publisher Link]
[55] Hong Peng et al., “Automatic Epileptic Seizure Detection Via Stein Kernel-based Sparse Representation,” Computers in Biology and Medicine, vol. 132, 2021.
[CrossRef] [Google Scholar] [Publisher Link]
[56] Mrutyunjaya Sahani, Susanta Kumar Rout, and Pradipta Kishor Dash, “Epileptic Seizure Recognition using Reduced Deep Convolutional Stack Autoencoder and Improved Kernel RVFLN from EEG Signals,” IEEE Transactions on Biomedical Circuits and Systems, vol. 15, no. 3, pp. 595-605, 2021.
[CrossRef] [Google Scholar] [Publisher Link]
[57] Jiuwen Cao et al., “Epileptic Classification with Deep-transfer-learning-based Feature Fusion Algorithm,” IEEE Transactions on Cognitive and Developmental Systems, vol. 14, no. 2, pp. 684-695, 2022.
[CrossRef] [Google Scholar] [Publisher Link]
[58] Andreas Miltiadous et al., “Machine Learning Algorithms for Epilepsy Detection based on Published EEG Databases: A Systematic Review,” IEEE Access, vol. 11, pp. 564-594, 2022.
[CrossRef] [Google Scholar] [Publisher Link]
[59] Ary L. Goldberger et al., “Physiobank, Physiotoolkit, and Physionet Components of a New Research Resource for Complex Physiologic Signals,” Circulation, vol. 101, no. 23, pp. 1-6, 2000.
[CrossRef] [Google Scholar] [Publisher Link]
[60] Magdalena Damian Chavez et al., “N&C-TEC: ECG and EEG Files,” Mendeley Data, 2020.
[CrossRef] [Publisher Link]
[61] R2024b Release Highlights – MATLAB and Simulink. [Online]. Available: https://in.mathworks.com/products/new_products/latest_features.html
[62] Rahul Kher, “Signal Processing Techniques for Removing Noise from ECG Signals,” Journal of Biomedical Engineering and Research, vol. 3, 2019.
[Google Scholar]