Bayesian Reinforcement Learning for Beam Forming in Millimetre-Wave Networks for Multi-Target Detection in 5G Radio Resource Allocation
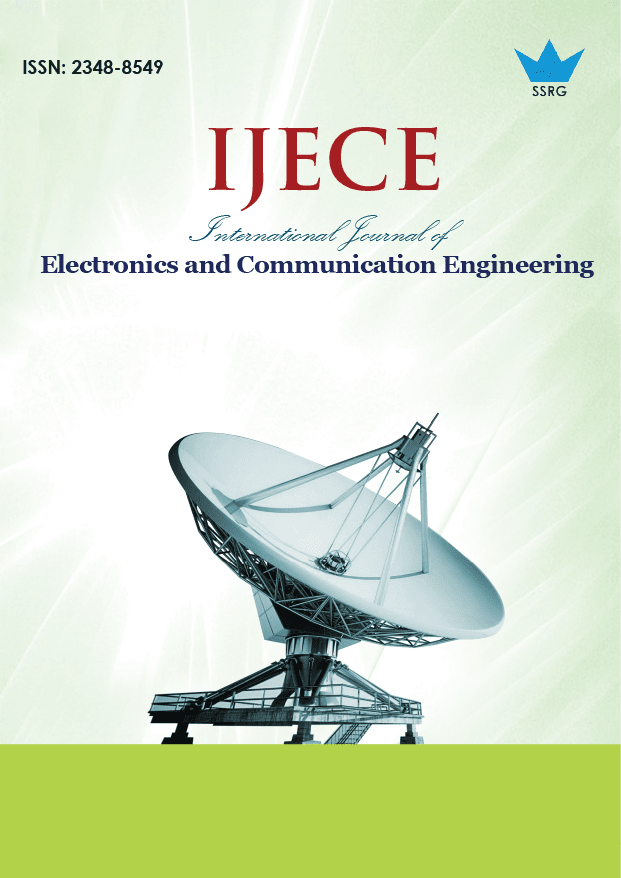
International Journal of Electronics and Communication Engineering |
© 2025 by SSRG - IJECE Journal |
Volume 12 Issue 1 |
Year of Publication : 2025 |
Authors : Phaneendra Jonnabhatla, T. Vimala |
How to Cite?
Phaneendra Jonnabhatla, T. Vimala, "Bayesian Reinforcement Learning for Beam Forming in Millimetre-Wave Networks for Multi-Target Detection in 5G Radio Resource Allocation," SSRG International Journal of Electronics and Communication Engineering, vol. 12, no. 1, pp. 174-192, 2025. Crossref, https://doi.org/10.14445/23488549/IJECE-V12I1P114
Abstract:
Millimeter wave (mmWave) beam forming has long been explored as a key enabler of Fifth-Generation (5G) technologies. Wireless network providers face numerous challenges due to the increasing density of wireless systems, including, but not limited to, the high costs associated with fiber construction and site acquisition. To address these challenges and take advantage of the maturity of mmWave communication for wireless backhaul links, Integrated Access and Backhaul (IAB) systems have been developed for 5G networks. In the context of 5G radio distribution, Bayesian Reinforcement Learning (BRL) introduces a novel approach to beamforming in mmWave systems, optimizing multi-target identification. Optimal beamforming is crucial for maximizing network capacity and ensuring effective utilization of the radio spectrum, as mmWave frequencies offer significant capacity and high-resolution capabilities. The proposed BRL framework improves beamforming strategies by probabilistically adapting to changing network conditions and user requirements through Bayesian estimation, thereby enhancing the accuracy of multi-target identification. The system dynamically adjusts beam patterns and resource allocation in real time based on immediate feedback, optimizing both signal quality and resource utilization. Experimental results demonstrate that BRL outperforms existing methods in resource utilization and target identification reliability, thereby increasing the capacity of mmWave systems in the 5G era. Extensive simulations and numerical results show that BRL-mmWave significantly improves efficiency and accelerates training compared to previous approaches.
Keywords:
Bayesian reinforcement learning, Beamforming, Millimeter-wave networks, Multi-target detection, 5G radio resource allocation, Adaptive beamforming, Network optimization, Resource utilization.
References:
[1] Wang Yi, Wei Zhiqing, and Feng Zhiyong, “Beam Training and Tracking in mmWave Communication: A Survey,” China Communications, vol. 21, no. 6, pp. 1-22, 2024.
[CrossRef] [Google Scholar] [Publisher Link]
[2] Jaspreet Kaur, “Exploiting the Location Information for Adaptive Beamforming in Transport Systems,” Ph.D. Thesis, University of Glasgow, pp. 1-150, 2024.
[Google Scholar] [Publisher Link]
[3] Karamot Kehinde Biliaminu et al., “Beam Prediction for mmWave V2I Communication Using ML-Based Multiclass Classification Algorithms,” Electronics, vol. 13, no. 13, pp. 1-21, 2024.
[CrossRef] [Google Scholar] [Publisher Link]
[4] Shailender, Shelej Khera, and Sajjan Singh, SBLKF- Based Estimation of Time- Selective (Fast-Fading) Channel for Hybrid mmWave Massive MIMO System, 1st ed., Intelligent Circuits and Systems for SDG 3 - Good Health and Well-Being, CRC Press, pp. 1-9, 2024.
[Google Scholar] [Publisher Link]
[5] Roscia J.S. Jayakumar, and Allwyn Clarence Asis Arul, “A Survey on Beamspace Millimeter-Wave Massive Mimo Systems: An Overview of Open Issues, Challenges, and Future Research Trends,” International Journal of Sensors Wireless Communications and Control, vol. 14, no. 1, pp. 1-20, 2024.
[CrossRef] [Google Scholar] [Publisher Link]
[6] K. Shoukath Ali et al., The Performance Analysis on Channel Estimation in Millimeter-Wave Communication and Their Challenges, Next Generation Wireless Communication, Springer, Cham, pp. 35-53, 2024.
[CrossRef] [Google Scholar] [Publisher Link]
[7] Dhiraj Shrivastava, Akash Gupta, and Bharat Verma, Applications of Reinforcement Learning in the Physical Layer of Wireless Communication, 1st ed., Applications of Computational Intelligence Techniques in Communications, CRC Press, pp. 53-65, 2024.
[Google Scholar] [Publisher Link]
[8] Kunlun Li et al., “Localization in Reconfigurable Intelligent Surface Aided mmWave Systems: A Multiple Measurement Vector Based Channel Estimation Method,” IEEE Transactions on Vehicular Technology, vol. 73, no. 9, pp. 13326-13339, 2024.
[CrossRef] [Google Scholar] [Publisher Link]
[9] Bushra Sabir et al., “Systematic Literature Review of AI-Enabled Spectrum Management in 6G and Future Networks,” arXiv, pp. 1-35, 2024.
[CrossRef] [Google Scholar] [Publisher Link]
[10] Ranjitham Govindasamy et al., “Deep Optimized Hybrid Beamforming Intelligent Reflecting Surface Assisted UM-MIMO THz Communication for 6G Broad Band Connectivity,” Telecommunication Systems, vol. 86, pp. 645-660, 2024.
[CrossRef] [Google Scholar] [Publisher Link]
[11] Lopamundra Hota, Biraja Prasad Nayak, and Arun Kumar, Algorithms for Optimization and in Intelligence Wireless Networks, 5G and Beyond Wireless Communications: Fundamentals, Applications, and Challenges, pp. 306-332, 2024.
[Google Scholar] [Publisher Link]
[12] Vijit Sai Tagirisa, “Develop a Predictive Model & Analyze the Impact on Common Memory Usage in a 5G Radio Unit,” Blekinge Institute of Technology, pp. 1-39, 2024.
[Google Scholar] [Publisher Link]
[13] Nurul Absar Siddiky, Muhammad Enayetur Rahman, and Shahriar Uzzal, “Beyond 5G: A Comprehensive Exploration of 6G Wireless Communication Technologies,” Preprints, pp. 1-49, 2024.
[CrossRef] [Google Scholar] [Publisher Link]
[14] Kennedy Chinedu Okafor et al., “Agile Gravitational Search Algorithm for Cyber-Physical Path-Loss Modelling in 5G Connected Autonomous Vehicular Network,” Vehicular Communications, vol. 45, pp. 1-18, 2024.
[CrossRef] [Google Scholar] [Publisher Link]
[15] Pradheep T.B. Rajan, and N. Muthukumaran, “Optimized CNN and Adaptive RBFNN for Channel Estimation and Hybrid Precoding Approaches for Multi User Millimeter Wave Massive MIMO,” International Journal of Electronics, pp. 1-24, 2024.
[CrossRef] [Google Scholar] [Publisher Link]
[16] Thulitha Senevirathna et al., “A Survey on XAI for 5G and Beyond Security: Technical Aspects, Challenges and Research Directions,” IEEE Communications Surveys & Tutorials, 2024.
[CrossRef] [Google Scholar] [Publisher Link]
[17] Khandoker Hoque et al., “Technological Trends in 5G Networks for IoT-Enabled Smart Healthcare: A Review,” International Journal of Science and Research Archive, vol. 12, no. 2, pp. 1399-1410, 2024.
[CrossRef] [Google Scholar] [Publisher Link]
[18] Balajee Sharma, and Akant Kumar Raghuwanshi, “Advancements in AI-Driven Techniques for MIMO-OFDM Systems: A Comparative Analysis,” International Journal of Innovative Research in Technology and Science, vol. 12, no. 2, pp. 427-433, 2024.
[Google Scholar] [Publisher Link]
[19] R. Ramalakshmi, and S. Tamil Selvi, “Spectral Efficiency Analysis on Massive MIMO Filter Bank,” International Journal of Electronics, vol. 111, no. 10, pp. 1631-1652, 2024.
[CrossRef] [Google Scholar] [Publisher Link]
[20] Degefe S. Hisabo, Thomas O. Olwal, and Murad R. Hassen, “Channel Modeling Techniques for Multiband Vehicle to Everything Communications: Challenges and Opportunities,” International Journal of Computing and Digital Systems, vol. 15, no. 1, pp. 29-50, 2024.
[Google Scholar]
[21] Peng Chen et al., “Beam Coverage Model of Ultra-Massive MIMO Communication Systems for Intelligent Transportation,” 2024 18th European Conference on Antennas and Propagation (EuCAP), Glasgow, United Kingdom, pp. 1-4, 2024.
[CrossRef] [Google Scholar] [Publisher Link]
[22] Peizheng Li et al., “BmmW: A DNN-Based Joint BLE and mmWave Radar System for Accurate 3D Localization with Goal-Oriented Communication,” Pervasive and Mobile Computing, vol. 102, 2024.
[CrossRef] [Google Scholar] [Publisher Link]
[23] Syed Adnan Yusuf, Arshad Khan, and Riad Souissi, “Vehicle-to-Everything (V2X) in the Autonomous Vehicles Domain - A Technical Review of Communication, Sensor, and AI Technologies for Road User Safety,” Transportation Research Interdisciplinary Perspectives, vol. 23, pp. 1-23, 2024.
[CrossRef] [Google Scholar] [Publisher Link]
[24] P. Prakash, G. Manoj, and J. Samson Immanuel, “A Comprehensive Review: Advances in MIMO Antenna for IOT Application,” Wireless Personal Communications, vol. 136, pp. 793-826, 2024.
[CrossRef] [Google Scholar] [Publisher Link]
[25] Xiaojie Wang et al., “Integration of Sensing, Communication, and Computing for Metaverse: A Survey,” ACM Computing Surveys, vol. 56, no. 10, pp. 1-38, 2024.
[CrossRef] [Google Scholar] [Publisher Link]
[26] Kai Zheng et al., “Enhancing mmWave Radar Sensing Using a Phased-MIMO Architecture,” Proceedings of the 22nd Annual International Conference on Mobile Systems, Applications and Services, Minato-ku, Tokyo Japan, pp. 56-69, 2024.
[CrossRef] [Google Scholar] [Publisher Link]
[27] Hang Thanh Bui et al., “Agriculture 4.0 and Beyond: Evaluating Cyber Threat Intelligence Sources and Techniques in Smart Farming Ecosystems,” Computers & Security, vol. 140, pp. 1-32, 2024.
[CrossRef] [Google Scholar] [Publisher Link]