Enhanced Breast Cancer Detection and Classification Using CNN-EfficientNet B3: A Deep Learning Approach with Segmentation and Feature Extraction from MIAS Dataset
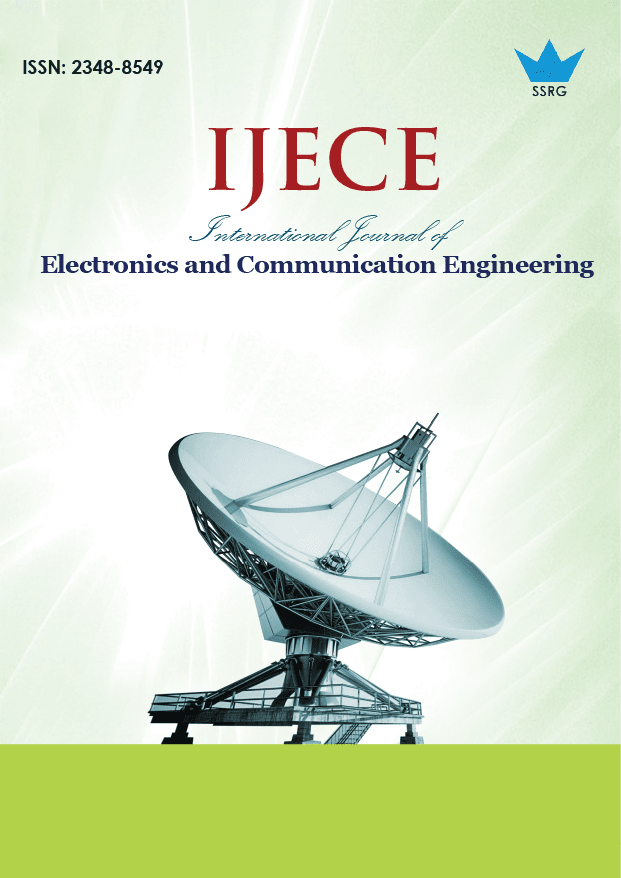
International Journal of Electronics and Communication Engineering |
© 2025 by SSRG - IJECE Journal |
Volume 12 Issue 1 |
Year of Publication : 2025 |
Authors : K. Punithavathi, G. Yamuna |
How to Cite?
K. Punithavathi, G. Yamuna, "Enhanced Breast Cancer Detection and Classification Using CNN-EfficientNet B3: A Deep Learning Approach with Segmentation and Feature Extraction from MIAS Dataset," SSRG International Journal of Electronics and Communication Engineering, vol. 12, no. 1, pp. 257-270, 2025. Crossref, https://doi.org/10.14445/23488549/IJECE-V12I1P120
Abstract:
Breast Cancer (BC), characterized by the uncontrolled proliferation of breast cells, remains the most prevalent and life-threatening cancer affecting women. Early detection significantly increases the chances of survival by enabling timely medical intervention. Numerous methods have been proposed for breast cancer detection; however, limitations in diagnostic accuracy and efficiency persist. To address these challenges, this study introduces a robust deep-learning framework leveraging fine-tuned EfficientNet-B3 for the detection and classification of breast tumors. The methodology employs segmentation techniques to accurately delineate the affected breast tumor regions, reducing training complexity while enhancing classification precision. The model was trained and evaluated using the Mammographic Image Analysis Society (MIAS) dataset, incorporating critical preprocessing steps to optimize image quality and feature extraction. Fine-tuning of EfficientNet-B3 was carried out to adapt the pre-trained network to mammogram-specific features, with hyperparameters optimized for this domain. Performance was assessed using three primary evaluation metrics: accuracy, specificity, and sensitivity. Experimental results demonstrate that the proposed CNN-EfficientNet-B3 framework achieves superior performance compared to conventional approaches, with specificity, accuracy, and sensitivity rates of 97.12%, 96.50%, and 96.43%, respectively. These findings highlight the potential of the proposed method to significantly enhance breast cancer detection and classification, paving the way for more effective clinical applications.
Keywords:
Convolutional Neural Networks, Deep Learning, Breast Cancer, EfficientNet, Mammogram.
References:
[1] Ihsan Jasim Hussein et al., “Fully-Automatic Identification of Gynaecological Abnormality Using a New Adaptive Frequency Filter and Histogram of Oriented Gradients (HOG),” Expert Systems, vol. 33, no. 3, pp. 1-47, 2021.
[CrossRef] [Google Scholar] [Publisher Link]
[2] Seifedine Kadry et al., “Extraction of Tumour in Breast MRI using Joint Thresholding and Segmentation-A Study, 2021 Seventh International Conference on Bio Signals, Images, and Instrumentation (ICBSII), Chennai, India, pp. 1-5, 2021.
[CrossRef] [Google Scholar] [Publisher Link]
[3] Rizwana Irfan et al., “Dilated Semantic Segmentation for Breast Ultrasonic Lesion Detection Using Parallel Feature Fusion,” Diagnostics, vol. 11, no. 7, pp. 1-20, 2021.
[CrossRef] [Google Scholar] [Publisher Link]
[4] Venkatesan Rajinikanth et al., “Breast Cancer Detection Using Thermal Images with Marine-Predators-Algorithm Selected Features,” 2021 Seventh International Conference on Bio Signals, Images, and Instrumentation (ICBSII), Chennai, India, pp. 1-6, 2021.
[CrossRef] [Google Scholar] [Publisher Link]
[5] Ihsan Jasim Hussein et al., “Fully Automatic Segmentation of Gynaecological Abnormality Using a New Viola-Jones Model,” Computers, Materials & Continua, vol. 66, no. 3, pp. 3161-318, 2021.
[CrossRef] [Google Scholar] [Publisher Link]
[6] Katharina Holland et al., “Quantification of Masking Risk in Screening Mammography with Volumetric Breast Density Maps,” Breast Cancer Research and Treatment, vol. 162, pp. 541-548, 2017.
[CrossRef] [Google Scholar] [Publisher Link]
[7] Adrian P. Brady, “Error and Discrepancy in Radiology: Inevitable or Avoidable?,” Insights into Imaging, vol. 8, pp. 171-182, 2017.
[CrossRef] [Google Scholar] [Publisher Link]
[8] Piero Olliaro, and Els Torreele, “Managing the Risks of Making the Wrong Diagnosis: First, do No Harm,” International Journal of Infectious Diseases, vol. 106, pp. 382-385, 2021.
[CrossRef] [Google Scholar] [Publisher Link]
[9] Saleem Z. Ramadan, “Methods Used in Computer-Aided Diagnosis for Breast Cancer Detection Using Mammograms: A Review,” Journal of Healthcare Engineering, vol. 2020, no. 1, pp. 1-21, 2020.
[CrossRef] [Google Scholar] [Publisher Link]
[10] Erich Sorantin, “The Augmented Radiologist: Artificial Intelligence in the Practice of Radiology,” Pediatric Radiology, vol. 52, pp. 2074-2086, 2022.
[CrossRef] [Google Scholar] [Publisher Link]
[11] Gelan Ayana, Kokeb Dese, and Se-woon Choe, “Transfer Learning in Breast Cancer Diagnoses via Ultrasound Imaging,” Cancers, vol. 13, no. 4, pp. 1-15, 2021.
[CrossRef] [Google Scholar] [Publisher Link]
[12] Hanan Aljuaid et al., “Computer-Aided Diagnosis for Breast Cancer Classification Using Deep Neural Networks and Transfer Learning,” Computer Methods and Programs in Biomedicine, vol. 223, pp. 1-10, 2022.
[CrossRef] [Google Scholar] [Publisher Link]
[13] Y. Wang et al., “Improved Breast Cancer Histological Grading Using Deep Learning,” Annals of Oncology, vol. 33, no. 1, pp. 89-98, 2022.
[CrossRef] [Google Scholar] [Publisher Link]
[14] Farjana Parvin, and Md. Al Mehedi Hasan, “A Comparative Study of Different Types of Convolutional Neural Networks for Breast Cancer Histopathological Image Classification,” 2020 IEEE Region 10 Symposium (TENSYMP), Dhaka, Bangladesh, pp. 945-948, 2020.
[CrossRef] [Google Scholar] [Publisher Link]
[15] Zabit Hameed et al., “Breast Cancer Histopathology Image Classification Using an Ensemble of Deep Learning Models,” Sensors, vol. 20, no. 16, 2020.
[CrossRef] [Google Scholar] [Publisher Link]
[16] Hanan A. Hosni Mahmoud, Amal H. Alharbi, and Doaa S. Khafga, “Breast Cancer Classification Using Deep Convolution Neural Network with Transfer Learning,” Intelligent Automation & Soft Computing, vol. 29, no. 3, pp. 803-814, 2021.
[CrossRef] [Google Scholar] [Publisher Link]
[17] Said Boumaraf et al., “A New Transfer Learning Based Approach to Magnification Dependent and Independent Classification of Breast Cancer in Histopathological Images,” Biomedical Signal Processing and Control, vol. 63, 2021.
[CrossRef] [Google Scholar] [Publisher Link]
[18] V.K. Reshma et al., “Detection of Breast Cancer Using Histopathological Image Classification Dataset with Deep Learning Techniques,” BioMed Research International, vol. 2022, no. 1, 2022.
[CrossRef] [Google Scholar] [Publisher Link]
[19] Madallah Alruwaili, and Walaa Gouda, “Automated Breast Cancer Detection Models Based on Transfer Learning,” Sensors, vol. 22, no. 3, 2022.
[CrossRef] [Google Scholar] [Publisher Link]
[20] Isha Gupta, Sheifali Gupta, and Swati Singh “Architectures Based on Deep Learning for the Detection of Invasive Ductal Carcinoma,” ECS Transactions, vol. 107, no. 1, 2022.
[CrossRef] [Google Scholar] [Publisher Link]
[21] Nusrat Ameen Barsha, Aimon Rahman, and M.R.C. Mahdy, “Automated Detection and Grading of Invasive Ductal Carcinoma Breast Cancer Using Ensemble of Deep Learning Models,” Computers in Biology and Medicine, vol. 139, 2021.
[CrossRef] [Google Scholar] [Publisher Link]
[22] Mamoona Humayun et al., “Framework for Detecting Breast Cancer Risk Presence Using Deep Learning,” Electronics, Vol. 12, no. 2, pp. 1-16, 2023.
[CrossRef] [Google Scholar] [Publisher Link]
[23] Mohammad Abdolah et al., “Artificial Intelligence in Automatic Classification of Invasive Ductal Carcinoma Breast Cancer in Digital Pathology Images,” Medical Journal of the Islamic Republic of Iran, vol. 34, no. 140, pp. 1-9, 2020.
[CrossRef] [Google Scholar] [Publisher Link]
[24] Jianfei Zhang et al., “Automatic Detection of Invasive Ductal Carcinoma Based on the Fusion of Multi-Scale Residual Convolutional Neural Network and SVM,” IEEE Access. vol. 9, pp. 40308-40317, 2021.
[CrossRef] [Google Scholar] [Publisher Link]
[25] Xiaomei Wang et al., “Intelligent Hybrid Deep Learning Model for Breast Cancer Detection,” Electronics, vol. 11, no. 17, pp. 1-18, 2022.
[CrossRef] [Google Scholar] [Publisher Link]
[26] Yusuf Celik et al., “Automated Invasive Ductal Carcinoma Detection Based Using Deep Transfer Learning with Whole-Slide Images,” Pattern Recognition Letters, vol. 133, pp. 232-239, 2020.
[CrossRef] [Google Scholar] [Publisher Link]
[27] Sukhendra Singh et al., “Hybrid Models for Breast Cancer Detection via Transfer Learning Technique,” Tech Science Press, vol. 74, no. 2, pp. 3063-3083, 2023.
[CrossRef] [Google Scholar] [Publisher Link]
[28] Jyoti Kundale, and Sudhir Dhage, “Classification of Breast Cancer Using Histology Images: Handcrafted and Pre-Trained Features-Based Approach,” IOP Conference Series: Materials Science and Engineering, vol. 1074, pp. 1-18, 2021.[CrossRef] [Google Scholar] [Publisher Link]