Modelling a Novel Random Trusted Multi-Aggregation Algorithm (RTMA2) for Enhances Data Aggregation
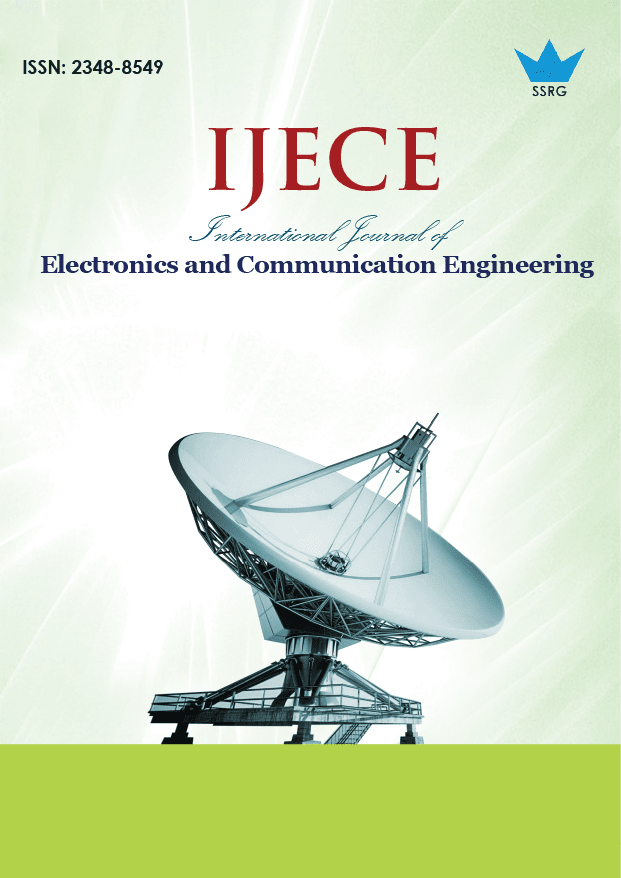
International Journal of Electronics and Communication Engineering |
© 2025 by SSRG - IJECE Journal |
Volume 12 Issue 3 |
Year of Publication : 2025 |
Authors : R. Nandha Kumar, P. Srimanchari |
How to Cite?
R. Nandha Kumar, P. Srimanchari, "Modelling a Novel Random Trusted Multi-Aggregation Algorithm (RTMA2) for Enhances Data Aggregation," SSRG International Journal of Electronics and Communication Engineering, vol. 12, no. 3, pp. 1-11, 2025. Crossref, https://doi.org/10.14445/23488549/IJECE-V12I3P101
Abstract:
It is essential to concentrate on the data aggregation process in order to improve the security of Wireless Sensor Networks (WSNs) for safe data transfer at both the Cluster Head (CH) and Base Station (BS). During data transmission, this technique combines data at each router. However, relying on energy-wasting nodes for this function can significantly shorten the network’s lifespan. Therefore, improving the data aggregation in WSNs is essential to increasing their energy efficiency. This refinement can be made at the CH and the BS using various mathematical techniques. Sensors are vulnerable to adversaries’ attacks when positioned over wide areas. These are the primary attackers who can introduce erroneous data into susceptible sensors during the data aggregation phase, resulting in inaccurate judgments at particular base stations. When it comes to such attacks, traditional methods of data averaging are unreliable. Observing how nodes behave during each data aggregation round is crucial to eliminate the inaccurate data introduced by these malicious parties precisely. This approach reduces the effect that inaccurate data has on the results. The Random Trusted Multi-Aggregation Algorithm (RTMA2) improves data aggregation by maximizing the energy efficiency of clustering by fusing a safe data aggregation technique with the capability to confirm data dependability. However, the entire data aggregation process is at risk since the aggregator has been compromised. Therefore, an aggregation protocol that maintains energy efficiency and security while also being resistant to compromised aggregators must be developed. In addition to reducing the dangers associated with compromised aggregators, The novel trust-based multi-aggregation protocol (RTMA2) shows how, in comparison to current methods, RTMA2 improves the energy efficiency, security, and adaptability of WSNs.
Keywords:
Sensor networks, Data aggregation, Trusted model, Random approach, Energy efficiency.
References:
[1] Raniyah Wazirali et al., “Secure Watermarking Schemes and Their Approaches in the IoT Technology: An Overview,” Electronics, vol. 10, no. 14, pp. 1-28, 2021.
[CrossRef] [Google Scholar] [Publisher Link]
[2] Khalid Haseeb et al., “RTS: A Robust and Trusted Scheme for IoT-Based Mobile Wireless Mesh Networks,” IEEE Access, vol. 8, pp. 68379-68390, 2020.
[CrossRef] [Google Scholar] [Publisher Link]
[3] Khattak Hasan Ali et al., “Cross-Layer Design and Optimization Techniques in Wireless Multimedia Sensor Networks for Smart Cities,” Computer Science and Information Systems, vol. 16, no. 1, pp. 1-17, 2019.
[CrossRef] [Google Scholar] [Publisher Link]
[4] Avishek Banerjee et al., “Design of Energy Efficient WSN Using a Noble SMOWA Algorithm,” Computers, Materials & Continua, vol. 72, no. 2, pp. 3585-3600, 2022.
[CrossRef] [Google Scholar] [Publisher Link]
[5] Zeeshan Ahmad et al., “Anomaly Detection Using Deep Neural Network for IoT Architecture,” Applied Sciences, vol. 11, no. 15, pp. 1-19, 2021.
[CrossRef] [Google Scholar] [Publisher Link]
[6] Laxminarayan Sahoo et al., “Optimization of Data Distributed Network System under Uncertainty,” Discrete Dynamics in Nature and Society, vol. 2022, no. 1, pp. 1-12, 2022.
[CrossRef] [Google Scholar] [Publisher Link]
[7] Chirihane Gherbi, Zibouda Aliouat, and Mohamed Benmohammed, “An Adaptive Clustering Approach to Dynamic Load Balancing and Energy Efficiency in Wireless Sensor Networks,” Energy, vol. 114, pp. 647-662, 2016.
[CrossRef] [Google Scholar] [Publisher Link]
[8] Palash Dutta, “Multi-Criteria Decision Making under Uncertainty via the Operations of Generalized Intuitionistic Fuzzy Numbers,” Granular Computing, vol. 6, pp. 321-337, 2021.
[CrossRef] [Google Scholar] [Publisher Link]
[9] A. Cagri Tolga, I. Burak Parlak, and Oscar Castillo, “Finite-Interval-Valued Type-2 Gaussian Fuzzy Numbers Applied to Fuzzy TODIM in a Healthcare Problem,” Engineering Applications of Artificial Intelligence, vol. 87, 2020.
[CrossRef] [Google Scholar] [Publisher Link]
[10] Elaheh Moharamkhani et al., “Multiobjective Fuzzy Knowledge-Based Bacterial Foraging Optimization for Congestion Control in Clustered Wireless Sensor Networks,” International Journal of Communication Systems, vol. 34, no. 16, 2021.
[CrossRef] [Google Scholar] [Publisher Link]
[11] Sonam Lata et al., “Fuzzy Clustering Algorithm for Enhancing Reliability and Network Lifetime of Wireless Sensor Networks,” IEEE Access, vol. 8, pp. 66013-66024, 2020.
[CrossRef] [Google Scholar] [Publisher Link]
[12] Damien Wohwe Sambo et al., “Optimized Clustering Algorithms for Large Wireless Sensor Networks: A Review,” Sensors, vol. 19, no. 2, pp. 1-27, 2019.
[CrossRef] [Google Scholar] [Publisher Link]
[13] Y.U. Xiu-wu et al., “A Clustering Routing Algorithm Based on Wolf Pack Algorithm for Heterogeneous Wireless Sensor Networks,” Computer Networks, vol. 167, 2020.
[CrossRef] [Google Scholar] [Publisher Link]
[14] S.R. Deepa, and D. Rekha, “Cluster Optimization in Wireless Sensor Networks Using Particle Swarm Optimization,” Social Transformation – Digital Way: 52nd Annual Convention of the Computer Society of India, Kolkata, India, pp. 240-253, 2018.
[CrossRef] [Google Scholar] [Publisher Link]
[15] Chun-Ho Chen, “A Novel Multi-Criteria Decision-Making Model for Building Material Supplier Selection Based on Entropy-AHP Weighted TOPSIS,” Entropy, vol. 22, no. 2, pp. 1-23, 2020.
[CrossRef] [Google Scholar] [Publisher Link]
[16] Laxminarayan Sahoo, “Some Score Functions on Fermatean Fuzzy Sets and its Application to Bride Selection Based on TOPSIS Method,” International Journal of Fuzzy System Applications, vol. 10, no. 3, pp. 18-29, 2021.
[CrossRef] [Google Scholar] [Publisher Link]
[17] Dinesh Kumar Kotary, and Satyasai Jagannath Nanda, “Distributed Robust Data Clustering in Wireless Sensor Networks Using Diffusion Moth Flame Optimization,” Engineering Applications of Artificial Intelligence, vol. 87, 2020.
[CrossRef] [Google Scholar] [Publisher Link]
[18] Syed Fawad Hussain, and Shahid Iqbal, “CCGA: Co-Similarity Based Co-Clustering Using Genetic Algorithm,” Applied Soft Computing, vol. 72, pp. 30-42, 2018.
[CrossRef] [Google Scholar] [Publisher Link]
[19] K.B. Gurumoorthy et al., “A Novel Clustering Method for Fault Recovery and Routing in Mobile Ad-Hoc Networks,” International Journal of Communication Systems, vol. 34, no. 15, 2021.
[CrossRef] [Google Scholar] [Publisher Link]
[20] Shunchao Zhang, “Riemannian Distance-Based Fast K-Medoids Clustering Algorithm for Cooperative Spectrum Sensing,” IEEE Systems Journal, vol. 16, no. 1, pp. 880-890, 2022.
[CrossRef] [Google Scholar] [Publisher Link]
[21] Saima Zafar, A. Bashir, and S.A. Chaudhry, “Mobility-Aware Hierarchical Clustering in Mobile Wireless Sensor Networks,” IEEE Access, vol. 7, pp. 20394-20403, 2019.
[CrossRef] [Google Scholar] [Publisher Link]
[22] Supriyan Sen et al., “Wireless Sensor Network Lifetime Extension via K-Medoids and MCDM Techniques in Uncertain Environment,” Applied Sciences, vol. 13, no. 5, pp. 1-20, 2023.
[CrossRef] [Google Scholar] [Publisher Link]
[23] Proshikshya Mukherjee et al., “Recommended System for Cluster Head Selection in a Remote Sensor Cloud Environment Using the Fuzzy-Based Multi-Criteria Decision-Making Technique,” Sustainability, vol. 13, no. 19, pp. 1-16, 2021.
[CrossRef] [Google Scholar] [Publisher Link]
[24] Bryan Raj et al., “A Survey on Cluster Head Selection and Cluster Formation Methods in Wireless Sensor Networks,” Wireless Communications and Mobile Computing, vol. 2022, pp. 1-53, 2022.
[CrossRef] [Google Scholar] [Publisher Link]
[25] Rakesh Kumar Yadav, and R.P. Mahapatra, “Energy Aware Optimized Clustering for Hierarchical Routing in Wireless Sensor Network,” Computer Science Review, vol. 41, 2021.
[CrossRef] [Google Scholar] [Publisher Link]
[26] Pramod Singh Rathore et al., “Energy-Efficient Cluster Head Selection through Relay Approach for WSN,” The Journal of Supercomputing, vol. 77, pp. 7649-7675, 2021.
[CrossRef] [Google Scholar] [Publisher Link]
[27] Amir Abbas Baradaran, and Keivan Navi, “HQCA-WSN: High-Quality Clustering Algorithm and Optimal Cluster Head Selection Using Fuzzy Logic in Wireless Sensor Networks,” Fuzzy Sets and Systems, vol. 389, pp. 114-144, 2020.
[CrossRef] [Google Scholar] [Publisher Link]
[28] Abel García-Nájera, Saúl Zapotecas-Martínez, and Karen Miranda, “Analysis of the Multi-Objective Cluster Head Selection Problem in WSNs,” Applied Soft Computing, vol. 112, 2021.
[CrossRef] [Google Scholar] [Publisher Link]
[29] Mohammad Al-Quraan et al., “Edge-Native Intelligence for 6G Communications Driven by Federated Learning: A Survey of Trends and Challenges,” IEEE Transactions on Emerging Topics in Computational Intelligence, vol. 7, no. 3, pp. 957-979, 2021.
[CrossRef] [Google Scholar] [Publisher Link]
[30] Ihsan Ullah, Hee Yong Youn, and Youn-Hee Han, “An Efficient Data Aggregation and Outlier Detection Scheme Based on Radial Basis Function Neural Network for WSN,” Journal of Ambient Intelligence and Humanized Computing, pp. 1-17, 2021.
[CrossRef] [Google Scholar] [Publisher Link]
[31] G. Saranraj, K. Selvamani, and P. Malathi, “A Novel Data Aggregation Using Multi Objective Based Male Lion Optimization Algorithm (DA-MOMLOA) in Wireless Sensor Network,” Journal of Ambient Intelligence and Humanized Computing, vol. 13, pp. 5645-5653, 2022.
[CrossRef] [Google Scholar] [Publisher Link]