Block-Based Fractional Wavelet Filter for Compression of Hyperspectral Images over Wireless Multimedia Sensor Network Platforms
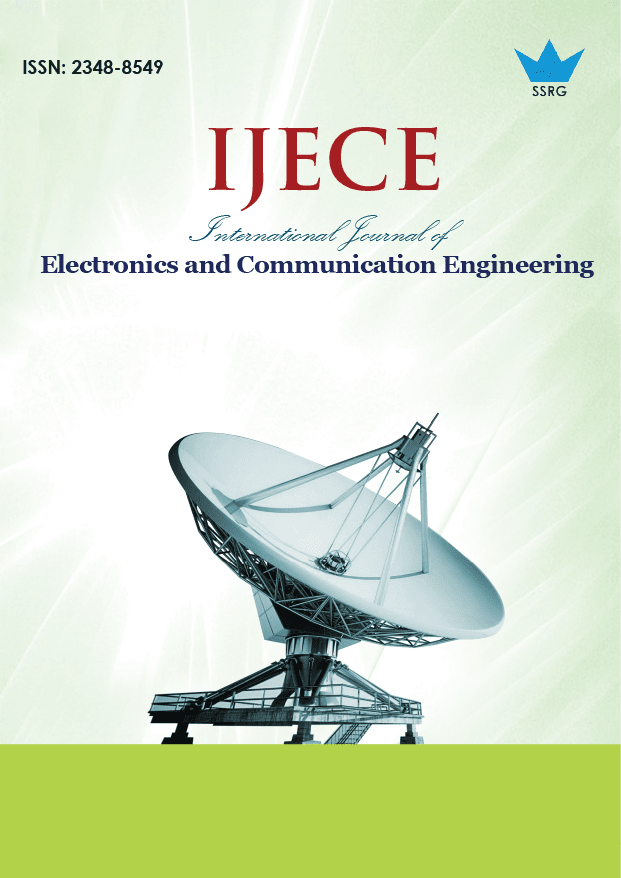
International Journal of Electronics and Communication Engineering |
© 2025 by SSRG - IJECE Journal |
Volume 12 Issue 3 |
Year of Publication : 2025 |
Authors : Rajesh, Shrish Bajpai, Naimur Rahman Kidwai |
How to Cite?
Rajesh, Shrish Bajpai, Naimur Rahman Kidwai, "Block-Based Fractional Wavelet Filter for Compression of Hyperspectral Images over Wireless Multimedia Sensor Network Platforms," SSRG International Journal of Electronics and Communication Engineering, vol. 12, no. 3, pp. 21-42, 2025. Crossref, https://doi.org/10.14445/23488549/IJECE-V12I3P103
Abstract:
With the property of excellent energy compaction and the ability to analyse hyperspectral images in the space-frequency domain, the 3D Dyadic Wavelet Transform is broadly used for the compression of hyperspectral images. However, calculating the transform coefficient through the conventional wavelet transform is memory intensive, which makes the compression algorithm unsuitable for the resource constraint hyperspectral image sensors. In the present study, the transform coefficients are calculated through block-based fractional wavelet transform frame by frame of the hyperspectral image to reduce the transform memory. The transform coefficient is coded with the zero memory set partitioned embedded block. We evaluated the proposed compression algorithm through MATLAB simulations with six popular hyperspectral images. From the result, it has been observed that demand for transform memory and transform complexity is reduced significantly while the requirement of the coding memory is zero and coding complexity is at par with other listless compression algorithms. Thus, the proposed compression algorithm is an optimum choice for the resource constraint hyperspectral image sensors.
Keywords:
Hyperspectral Image Compression, Low Memory Architecture, Lossy Compression, Hyperspectral Image Coding, Wavelet Transform, Low Complexity.
References:
[1] B. Krishna Mohan, and Alok Porwal, “Hyperspectral Image Processing and Analysis,” Current Science, vol. 108, no. 5, pp. 833-841, 2015.
[Google Scholar] [Publisher Link]
[2] D. Chutia et al., “Hyperspectral Remote Sensing Classifications: A Perspective Survey,” Transactions in GIS, vol. 20, no. 4, pp. 463-490, 2016.
[CrossRef] [Google Scholar] [Publisher Link]
[3] Chaitanya B. Pande, and Kanak N. Moharir, Application of Hyperspectral Remote Sensing Role in Precision Farming and Sustainable Agriculture under Climate Change: A Review, Climate Change Impacts on Natural Resources, Ecosystems and Agricultural Systems, Springer, Cham, pp. 503-520, 2023.
[CrossRef] [Google Scholar] [Publisher Link]
[4] Jean-Baptiste Courbot et al., “Pairwise Markov Fields for Segmentation in Astronomical Hyperspectral Images,” Signal Processing, vol. 163, pp. 41-48, 2019.
[CrossRef] [Google Scholar] [Publisher Link]
[5] Yihui Zhou et al., “Machine Learning Empowered Coherent Raman Imaging and Analysis for Biomedical Applications,” Communications Engineering, vol. 4, pp. 1-14, 2025.
[CrossRef] [Google Scholar] [Publisher Link]
[6] Jessica Roberts et al., “A Short Update on the Advantages, Applications and Limitations of Hyperspectral and Chemical Imaging in Food Authentication,” Applied Sciences, vol. 8, no. 4, pp. 1-7, 2018.
[CrossRef] [Google Scholar] [Publisher Link]
[7] Dominik Thomas, and Max Gündel, “Hyperspectral Imaging Systems for Corrosion Detection from Remotely Operated Vehicles,” CE/Papers, vol. 6, no. 5, pp. 934-938, 2023.
[CrossRef] [Google Scholar] [Publisher Link]
[8] Lingxi Liu et al., “Neural Networks for Hyperspectral Imaging of Historical Paintings: A Practical Review,” Sensors, vol. 23, no. 5, pp. 1-25, 2023.
[CrossRef] [Google Scholar] [Publisher Link]
[9] Ndubisi A. Aviara et al., “Potential Application of Hyperspectral Imaging in Food Grain Quality Inspection, Evaluation and Control during Bulk Storage,” Journal of Agriculture and Food Research, vol. 8, pp. 1-10, 2022.
[CrossRef] [Google Scholar] [Publisher Link]
[10] Kristiane de Cássia Mariotti et al., “Hyperspectral Imaging in Forensic Science: An Overview of Major Application Areas,” Science & Justice, vol. 63, no. 3, pp. 387-395, 2023.
[CrossRef] [Google Scholar] [Publisher Link]
[11] K. Karthik et al., “Study of Land Cover Classification from Hyperspectral Images Using Deep Learning Algorithm,” Computer Networks and Inventive Communication Technologies: Proceedings of Fifth ICCNCT 2022, pp. 721-737, 2023.
[CrossRef] [Google Scholar] [Publisher Link]
[12] Baowei Fei, “Hyperspectral Imaging In Medical Applications,” Data Handling in Science and Technology, vol. 32, pp. 523-565, 2019.
[CrossRef] [Google Scholar] [Publisher Link]
[13] Michal Shimoni, Rob Haelterman, and Christiaan Perneel, “Hypersectral Imaging for Military and Security Applications: Combining Myriad Processing and Sensing Techniques,” IEEE Geoscience and Remote Sensing Magazine, vol. 7, no. 2, pp. 101-117, 2019.
[CrossRef] [Google Scholar] [Publisher Link]
[14] Laureen Coic et al., “Essential Spectra to Improve Vibrational Imaging of Pharmaceutical Samples,” Microchemical Journal, vol. 209, pp. 1-9, 2025.
[CrossRef] [Google Scholar] [Publisher Link]
[15] Alankar Kotwal et al., “Hyperspectral Imaging in Neurosurgery: A Review of Systems, Computational Methods, and Clinical Applications,” Journal of Biomedical Optics, vol. 30, no. 2, pp. 1-54, 2025.
[CrossRef] [Google Scholar] [Publisher Link]
[16] Sneha, and Ajay Kaul, “Hyperspectral Imaging and Target Detection Algorithms: A Review,” Multimedia Tools and Applications, vol. 81, pp. 44141-44206, 2022.
[CrossRef] [Google Scholar] [Publisher Link]
[17] Kohzo Homma et al., “Water Pollution Monitoring Using a Hyperspectral Imaging Spectropolarimeter,” Multispectral and Hyperspectral Remote Sensing Instruments and Applications II, vol. 5655, pp. 419-426, 2005.
[CrossRef] [Google Scholar] [Publisher Link]
[18] Mihaela Antonina Calin, Adrian Cantemir Calin, and Doina Nicoleta Nicolae, “Application of Airborne and Spaceborne Hyperspectral Imaging Techniques for Atmospheric Research: Past, Present, and Future,” Applied Spectroscopy Reviews, vol. 56, no. 4, pp. 289-323, 2021.
[CrossRef] [Google Scholar] [Publisher Link]
[19] V.R.S. Mani, “Comparison of Three Un-Mixing Based Hyperspectral Image Fusion Techniques,” Journal of the Geological Society of India, vol. 91, pp. 541-546, 2018.
[CrossRef] [Google Scholar] [Publisher Link]
[20] Yaman Dua et al., “Convolution Neural Network Based Lossy Compression of Hyperspectral Images,” Signal Processing: Image Communication, vol. 95, 2021.
[CrossRef] [Google Scholar] [Publisher Link]
[21] Yaman Dua, Ravi Shankar Singh, and Vinod Kumar, “Compression of Multi-Temporal Hyperspectral Images Based on RLS Filter,” The Visual Computer, vol. 38, pp. 65-75, 2022.
[CrossRef] [Google Scholar] [Publisher Link]
[22] Brajesh Kumar et al., “Feature Extraction for Hyperspectral Image Classification: A Review,” International Journal of Remote Sensing, vol. 41, no. 16, pp. 6248-6287, 2020.
[CrossRef] [Google Scholar] [Publisher Link]
[23] Gulsen Taskin, E. Fatih Yetkin, and Gustau Camps-Valls, “A Scalable Unsupervised Feature Selection with Orthogonal Graph Representation for Hyperspectral Images,” IEEE Transactions on Geoscience and Remote Sensing, vol. 61, pp. 1-13, 2023.
[CrossRef] [Google Scholar] [Publisher Link]
[24] Mohammad Esmaeili et al., “Hyperspectral Image Band Selection Based on CNN Embedded GA (CNNeGA),” IEEE Journal of Selected Topics in Applied Earth Observations and Remote Sensing, vol. 16, pp. 1927-1950, 2023.
[CrossRef] [Google Scholar] [Publisher Link]
[25] Chiman Kwan, “Methods and Challenges Using Multispectral and Hyperspectral Images for Practical Change Detection Applications,” Information, vol. 10, no. 11, pp. 1-29, 2019.
[CrossRef] [Google Scholar] [Publisher Link]
[26] Aloke Datta, Susmita Ghosh, and Ashish Ghosh, “Supervised Feature Extraction of Hyperspectral Images Using Partitioned Maximum Margin Criterion,” IEEE Geoscience and Remote Sensing Letters, vol. 14, no. 1, pp. 82-86, 2017.
[CrossRef] [Google Scholar] [Publisher Link]
[27] Xing Wei, Jiahua Xiao, and Yihong Gong, “Blind Hyperspectral Image Denoising with Degradation Information Learning,” Remote Sensing, vol. 15, no. 2, pp. 1-23, 2023.
[CrossRef] [Google Scholar] [Publisher Link]
[28] Mohammed Abdulmajeed Moharram, and Divya Meena Sundaram, “Dimensionality Reduction Strategies for Land Use Land Cover Classification Based on Airborne Hyperspectral Imagery: A Survey,” Environmental Science and Pollution Research, vol. 30, no. 3, pp. 5580-5602, 2023.
[CrossRef] [Google Scholar] [Publisher Link]
[29] Reaya Grewal, Singara Singh Kasana, and Geeta Kasana, “Hyperspectral Image Segmentation: A Comprehensive Survey,” Multimedia Tools and Applications, vol. 82, pp. 20819-20872, 2023.
[CrossRef] [Google Scholar] [Publisher Link]
[30] Silvia Valero, Philippe Salembier, and Jocelyn Chanussot, “Object Recognition in Hyperspectral Images Using Binary Partition Tree Representation,” Pattern Recognition Letters, vol. 56, pp. 45-51, 2015.
[CrossRef] [Google Scholar] [Publisher Link]
[31] Yaman Dua, Vinod Kumar, and Ravi Shankar Singh, “Comprehensive Review of Hyperspectral Image Compression Algorithms,” Optical Engineering, vol. 59, no. 9, pp. 1-39, 2020.
[CrossRef] [Google Scholar] [Publisher Link]
[32] Nor Rizuan Mat Noor, and Tanya Vladimirova, “Investigation Into Lossless Hyperspectral Image Compression for Satellite Remote Sensing,” International Journal of Remote Sensing, vol. 34, no. 14, pp. 5072-5104, 2013.
[CrossRef] [Google Scholar] [Publisher Link]
[33] Jose Melián et al., “A Novel Data Reutilization Strategy for Real-Time Hyperspectral Image Compression,” IEEE Geoscience and Remote Sensing Letters, vol. 19, pp. 1-5, 2022.
[CrossRef] [Google Scholar] [Publisher Link]
[34] Antonis Tsigkanos et al., “High-Performance COTS FPGA SoC for Parallel Hyperspectral Image Compression with CCSDS-123.0-B-1,” IEEE Transactions on Very Large Scale Integration (VLSI) Systems, vol. 28, no. 11, pp. 2397-2409, 2020.
[CrossRef] [Google Scholar] [Publisher Link]
[35] Shrish Bajpai, and Naimur Rahman Kidwai, “Fractional Wavelet Filter Based Low Memory Coding for Hyperspectral Image Sensors,” Multimedia Tools and Applications, vol. 83, pp. 26281-26306, 2024.
[CrossRef] [Google Scholar] [Publisher Link]
[36] Rui Dusselaar, and Manoranjan Paul, “Hyperspectral Image Compression Approaches: Opportunities, Challenges, and Future Directions: Discussion,” Journal of the Optical Society of America A, vol. 34, no. 12, pp. 2170-2180, 2017.
[CrossRef] [Google Scholar] [Publisher Link]
[37] Dristi Datta et al., “Comparative Analysis of Machine and Deep Learning Models for Soil Properties Prediction from Hyperspectral Visual Band,” Environments, vol. 10, no. 5, pp. 1-18, 2023.
[CrossRef] [Google Scholar] [Publisher Link]
[38] Dristi Datta et al., “Soil Moisture, Organic Carbon, and Nitrogen Content Prediction with Hyperspectral Data Using Regression Models,” Sensors, vol. 22, no. 20, pp. 1-20, 2022.
[CrossRef] [Google Scholar] [Publisher Link]
[39] Nisha Rani, Venkata Ravibabu Mandla, and Tejpal Singh, “Performance of Image Classification on Hyperspectral Imagery for Lithological Mapping,” Journal of the Geological Society of India, vol. 88, pp. 440-448, 2016.
[CrossRef] [Google Scholar] [Publisher Link]
[40] Zhuang Zhao et al., “Redundant Compressed Single-Pixel Hyperspectral Imaging System,” Optics Communications, vol. 546, 2023.
[CrossRef] [Google Scholar] [Publisher Link]
[41] Mohd Tausif, Ekram Khan, and Antonio Pinheiro, “Computationally Efficient Wavelet-Based Low Memory Image Coder for WMSNs/IoT,” Multidimensional Systems and Signal Processing, vol. 34, pp. 657-680, 2023.
[CrossRef] [Google Scholar] [Publisher Link]
[42] Diego Valsesia, and Enrico Magli, “A Novel Rate Control Algorithm for Onboard Predictive Coding of Multispectral and Hyperspectral Images,” IEEE Transactions on Geoscience and Remote Sensing, vol. 52, no. 10, pp. 6341-6355, 2014.
[CrossRef] [Google Scholar] [Publisher Link]
[43] Shen-en Qian et al., “Fast Three-Dimensional Data Compression of Hyperspectral Imagery Using Vector Quantization with Spectral-Feature-Based Binary Coding,” Optical Engineering, vol. 35, no. 11, pp. 3242-3249, 1996.
[CrossRef] [Google Scholar] [Publisher Link]
[44] R. Nagendran, and A. Vasuki, “Hyperspectral Image Compression Using Hybrid Transform with Different Wavelet-Based Transform Coding,” International Journal of Wavelets, Multiresolution and Information Processing, vol. 18, no. 1, 2020.
[CrossRef] [Google Scholar] [Publisher Link]
[45] K.S. Gunasheela, and H.S. Prasantha, “Compressive Sensing Approach to Satellite Hyperspectral Image Compression,” Information and Communication Technology for Intelligent Systems, pp. 495-503, 2018.
[CrossRef] [Google Scholar] [Publisher Link]
[46] Samiran Das, “Hyperspectral Image, Video Compression Using Sparse Tucker Tensor Decomposition,” IET Image Processing, vol. 15, no. 4, pp. 964-973, 2021.
[CrossRef] [Google Scholar] [Publisher Link]
[47] Chubo Deng, Yi Cen, and Lifu Zhang, “Learning-Based Hyperspectral Imagery Compression through Generative Neural Networks,” Remote Sensing, vol. 12, no. 21, pp. 1-19, 2020.
[CrossRef] [Google Scholar] [Publisher Link]
[48] Yuanyuan Guo et al., “Edge-Guided Hyperspectral Image Compression with Interactive Dual Attention,” IEEE Transactions on Geoscience and Remote Sensing, vol. 61, pp. 1-17, 2023.
[CrossRef] [Google Scholar] [Publisher Link]
[49] Agnieszka C. Miguel et al., Predictive Coding of Hyperspectral Images, Hyperspectral Data Compression, Springer, Boston, MA, pp. 197-231, 2006.
[CrossRef] [Google Scholar] [Publisher Link]
[50] Daniel Báscones, Carlos González, and Daniel Mozos, “Hyperspectral Image Compression Using Vector Quantization, PCA and JPEG2000,” Remote Sensing, vol. 10, no. 6, pp. 1-13, 2018.
[CrossRef] [Google Scholar] [Publisher Link]
[51] Rui Li, Zhibin Pan, and Yang Wang, “The Linear Prediction Vector Quantization for Hyperspectral Image Compression,” Multimedia Tools and Applications, vol. 78, pp. 11701-11718, 2019.
[CrossRef] [Google Scholar] [Publisher Link]
[52] Irem Ulku, and Behçet Uğur Töreyin, “Sparse Representations for Online-Learning-Based Hyperspectral Image Compression,” Applied optics, vol. 54, no. 29, pp. 8625-8631, 2015.
[CrossRef] [Google Scholar] [Publisher Link]
[53] Azam Karami, Soosan Beheshti, and Mehran Yazdi, “Hyperspectral Image Compression Using 3D Discrete Cosine Transform and Support Vector Machine Learning,” 11th International Conference on Information Science, Signal Processing and their Applications, Montreal, QC, Canada, pp. 809-812, 2012.
[CrossRef] [Google Scholar] [Publisher Link]
[54] Francesco Rizzo, Giovanni Motta, and James A. Storer, Hyperspectral Data Compression, Springer US, pp. 1-417, 2006.
[Google Scholar] [Publisher Link]
[55] Amal Altamimi, and Belgacem Ben Youssef, “A Systematic Review of Hardware-Accelerated Compression of Remotely Sensed Hyperspectral Images,” Sensors, vol. 22, no. 1, pp. 1-53, 2021.
[CrossRef] [Google Scholar] [Publisher Link]
[56] Basant K. Mohanty, and Pramod K. Meher, “Memory-Efficient Architecture for 3-D DWT Using Overlapped Grouping of Frames,” IEEE Transactions on Signal Processing, vol. 59, no. 11, pp. 5605-5616, 2011.
[CrossRef] [Google Scholar] [Publisher Link]
[57] Jiaji Wu, Zhensen Wu, and Chengke Wu, “Lossy to Lossless Compressions of Hyperspectral Images Using Three-Dimensional Set Partitioning Algorithm,” Optical Engineering, vol. 45, no. 2, 2006.
[CrossRef] [Google Scholar] [Publisher Link]
[58] Emmanuel Christophe, Corinne Mailhes, and Pierre Duhamel, “Hyperspectral Image Compression: Adapting SPIHT and EZW to Anisotropic 3-D Wavelet Coding,” IEEE Transactions on Image processing, vol. 17, no. 12, pp. 2334-2346, 2008.
[CrossRef] [Google Scholar] [Publisher Link]
[59] Xiaoli Tang, and W.A. Pearlman, “Lossy-to-Lossless Block-Based Compression of Hyperspectral Volumetric Data,” International Conference on Image Processing, Singapore, vol. 5, pp. 3283-3286, 2004.
[CrossRef] [Google Scholar] [Publisher Link]
[60] Xiaoli Tang, and William A. Pearlman, Three-Dimensional Wavelet-based Compression of Hyperspectral Images, Hyperspectral Data Compression, Springer, Boston, MA, pp. 273-308, 2006.
[CrossRef] [Google Scholar] [Publisher Link]
[61] Shrish Bajpai, Naimur Rahman Kidwai, and Harsh Vikram Singh, “3D Wavelet Block Tree Coding for Hyperspectral Images,” International Journal of Innovative Technology and Exploring Engineering, vol. 8, no. 6C, pp. 64-68, 2019.
[Google Scholar] [Publisher Link]
[62] Ruzelita Ngadiran et al., “Efficient Implementation of 3D Listless Speck,” International Conference on Computer and Communication Engineering, Kuala Lumpur, Malaysia, pp. 1-4, 2010.
[CrossRef] [Google Scholar] [Publisher Link]
[63] V.K. Sudha, and R. Sudhakar, “3D Listless Embedded Block Coding Algorithm for Compression of Volumetric Medical Images,” Journal of Scientific and industrial Research, vol. 72, pp. 735-748, 2013.
[Google Scholar] [Publisher Link]
[64] Shrish Bajpai et al., “Low Memory Block Tree Coding for Hyperspectral Images,” Multimedia Tools and Applications, vol. 78, no. 19, pp. 27193-27209, 2019.
[CrossRef] [Google Scholar] [Publisher Link]
[65] Shrish Bajpai, “Low Complexity Block Tree Coding for Hyperspectral Image Sensors,” Multimedia Tools and Applications, vol. 81, no. 23, pp. 33205-33323, 2022.
[CrossRef] [Google Scholar] [Publisher Link]
[66] Shrish Bajpai et al., “A Low Complexity Hyperspectral Image Compression through 3D Set Partitioned Embedded Zero Block Coding,” Multimedia Tools and Applications, vol. 81, no. 1, pp. 841-872, 2022.
[CrossRef] [Google Scholar] [Publisher Link]
[67] Shrish Bajpai et al., “Curvelet Transform Based Compression Algorithm for Low Resource Hyperspectral Image Sensors,” Journal of Electrical and Computer Engineering, vol. 2023, pp. 1-18, 2023.
[CrossRef] [Google Scholar] [Publisher Link]
[68] Mohd Tausif et al., “Memory-Efficient Architecture for FrWF-Based DWT of High-Resolution Images for IoMT Applications,” Multimedia Tools and Applications, vol. 80, pp. 11177-11199, 2021.
[CrossRef] [Google Scholar] [Publisher Link]
[69] Mohd Tausif et al., “SFrWF: Segmented Fractional Wavelet Filter Based DWT for Low Memory Image Coders,” 4th IEEE Uttar Pradesh Section International Conference on Electrical, Computer and Electronics, Mathura, India, pp. 593-597, 2017.
[CrossRef] [Google Scholar] [Publisher Link]
[70] Mohd Tausif, Ekram Khan, and Mohd Hasan, “BFrWF: Block-Based FrWF for Coding of High-Resolution Images with Memory-Complexity Constrained-Devices,” 5th IEEE Uttar Pradesh Section International Conference on Electrical, Electronics and Computer Engineering, Gorakhpur, India, pp. 1-5, 2018.
[CrossRef] [Google Scholar] [Publisher Link]
[71] Mohd Tausif et al., “SMFrWF: Segmented Modified Fractional Wavelet Filter: Fast Low-Memory Discrete Wavelet Transform (DWT),” IEEE Access, vol. 7, pp. 84448-84467, 2019.
[CrossRef] [Google Scholar] [Publisher Link]
[72] Stephan Rein, and Martin Reisslein, “Performance Evaluation of the Fractional Wavelet Filter: A Low-Memory Image Wavelet Transform for Multimedia Sensor Networks,” Ad Hoc Networks, vol. 9, no. 4, pp. 482-496, 2011.
[CrossRef] [Google Scholar] [Publisher Link]
[73] Mohd Tausif et al., “Lifting-Based Fractional Wavelet Filter: Energy-Efficient DWT Architecture for Low-Cost Wearable Sensors,” Advances in Multimedia, vol. 2020, no. 1, pp. 1-13, 2020.
[CrossRef] [Google Scholar] [Publisher Link]
[74] Divya Sharma, Y.K. Prajapati, and R. Tripathi, “Success Journey of Coherent PM-QPSK Technique with its Variants: A Survey,” IETE Technical Review, vol. 37, no. 1, pp. 36-55, 2020.
[CrossRef] [Google Scholar] [Publisher Link]
[75] W.A. Pearlman et al., “Efficient, Low-Complexity Image Coding with a Set-Partitioning Embedded Block Coder,” IEEE Transactions on Circuits and Systems for Video Technology, vol. 14, no. 11, pp. 1219-1235, 2004.
[CrossRef] [Google Scholar] [Publisher Link]
[76] Mrityunjaya V. Latte, Narasimha H. Ayachit, and D.K. Deshpande, “Reduced Memory Listless Speck Image Compression,” Digital Signal Processing, vol. 16, no. 6, pp. 817-824, 2006.
[CrossRef] [Google Scholar] [Publisher Link]
[77] Divya Sharma, “Image Quality Assessment Metrics for Hyperspectral Image Compression Algorithms,” Second International Conference Computational and Characterization Techniques in Engineering & Sciences, Lucknow, India, pp. 1-5, 2024.
[CrossRef] [Google Scholar] [Publisher Link]
[78] Nadia Zikiou, Mourad Lahdir, and David Helbert, “Support Vector Regression-Based 3D-Wavelet Texture Learning for Hyperspectral Image Compression,” The Visual Computer, vol. 36, no. 7, pp. 1473-1490, 2020.
[CrossRef] [Google Scholar] [Publisher Link]
[79] Divya Sharma, Y.K. Prajapati, and Rajeev Tripathi, “0.55 Tb/s Heterogeneous Nyquist-WDM Superchannel Using Different Polarization Multiplexed Subcarriers,” Photonic Network Communications, vol. 39, pp. 120-128, 2020.
[CrossRef] [Google Scholar] [Publisher Link]
[80] Samiran Das, and Sandip Ghosal, “Unmixing Aware Compression of Hyperspectral Image by Rank Aware Orthogonal Parallel Factorization Decomposition,” Journal of Applied Remote Sensing, vol. 17, no. 4, 2023.
[CrossRef] [Google Scholar] [Publisher Link]
[81] Pallavi Ranjan et al., “Revolutionizing Hyperspectral Image Classification for Limited Labeled Data: Unifying Autoencoder-Enhanced GANs with Convolutional Neural Networks and Zero-Shot Learning,” Earth Science Informatics, vol. 18, no. 2, pp. 1-26, 2025.
[CrossRef] [Google Scholar] [Publisher Link]