Real-Time Non-Intrusive Monitoring of Wear and Damage on Mining Truck Tires Using Digital Image Processing
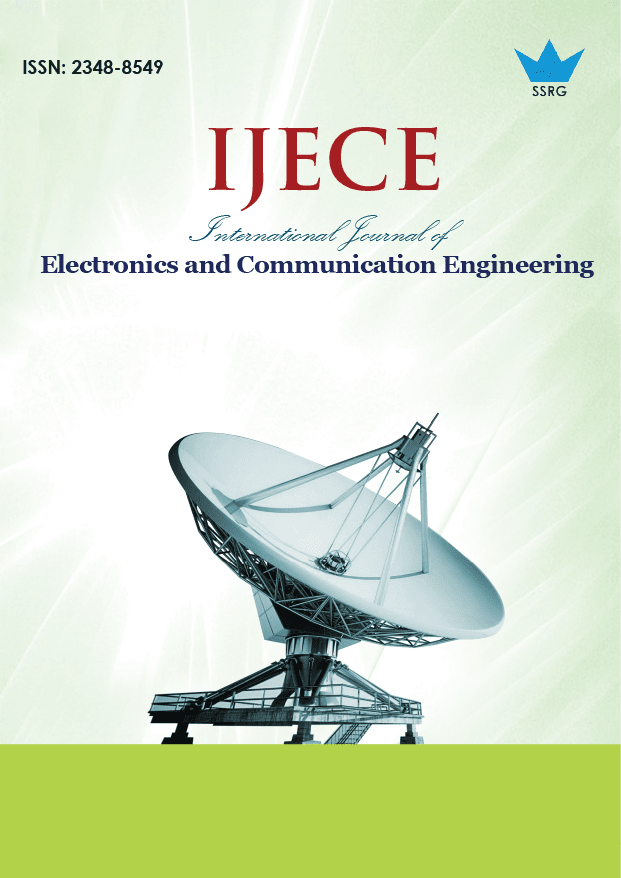
International Journal of Electronics and Communication Engineering |
© 2025 by SSRG - IJECE Journal |
Volume 12 Issue 3 |
Year of Publication : 2025 |
Authors : Gianfranco Jose Farfan Silva, Leonel Fred Caceres Solorzano, Jesus Talavera Suarez |
How to Cite?
Gianfranco Jose Farfan Silva, Leonel Fred Caceres Solorzano, Jesus Talavera Suarez, "Real-Time Non-Intrusive Monitoring of Wear and Damage on Mining Truck Tires Using Digital Image Processing," SSRG International Journal of Electronics and Communication Engineering, vol. 12, no. 3, pp. 134-142, 2025. Crossref, https://doi.org/10.14445/23488549/IJECE-V12I3P113
Abstract:
Truck tires, vulnerable to severe wear and possible damage in hostile settings, are a major component of mining operations' dependability and safety. This study describes a real-time, non-intrusive monitoring system that uses Digital Image Processing methods to identify tire wear and corrosion on mining trucks. While the mining truck is in motion, the system continuously captures video images of the surface via a camera located on the tire's fender. The processing method analyses these photos in real-time and categorises potential hazards such as jammed rocks, embedded nails or foreign wear. Because the system can quickly identify and report these problems, corrective action can be taken immediately, reducing the likelihood of tire failure and improving overall operational safety. The method ensures the longevity and performance of mining truck tires while reducing downtime and maintenance costs. It is also reasonably priced and scalable. Preliminary tests demonstrate the system's effectiveness in various mining situations, underscoring the potential for widespread use in the industry.
Keywords:
Tire wear and damage detection, Digital image processing, Non-intrusive monitoring, Mining truck maintenance.
References:
[1] R. Pascual et al., “Reducing Mining Footprint by Matching Haul Fleet Demand and Route-Oriented Tire Types,” Journal of Cleaner Production, vol. 227, pp. 645-651, 2019.
[CrossRef] [Google Scholar] [Publisher Link]
[2] Zahid Mahmood, “Digital Image Processing: Advanced Technologies and Applications,” Applied Sciences, vol. 14, no. 14, pp. 1-6, 2024.
[CrossRef] [Google Scholar] [Publisher Link]
[3] Aysegul Ucar, Mehmet Karakose, and Necim Kırımca, “Artificial Intelligence for Predictive Maintenance Applications: Key Components, Trustworthiness, and Future Trends,” Applied Sciences, vol. 14, no. 2, pp. 1-40, 2024.
[CrossRef] [Google Scholar] [Publisher Link]
[4] Izaz Raouf, Hyewon Lee, and Heung Soo Kim, “Mechanical Fault Detection Based on Machine Learning for Robotic RV Reducer Using Electrical Current Signature Analysis: A Data-Driven Approach,” Journal of Computational Design and Engineering, vol. 9, no. 2, pp. 417-433, 2022.
[CrossRef] [Google Scholar] [Publisher Link]
[5] Julio Alegre Luna et al., “Development of a Touchless Control System for a Clinical Robot with Multimodal User Interface,” International Journal of Advanced Computer Science and Applications, vol. 14, no. 9, pp. 1068-1075, 2023.
[CrossRef] [Google Scholar] [Publisher Link]
[6] Francesca Guerriero, Luigi Di Puglia Pugliese, and Nathalie Mitton, “Efficient Wireless Sensor Deployment at Minimum Cost,” Proceedings 18th International Conference on Ad-Hoc, Mobile, and Wireless Networks, Luxembourg, pp. 575-587, 2019.
[CrossRef] [Google Scholar] [Publisher Link]
[7] Ilie Gligorea et al., “Adaptive Learning Using Artificial Intelligence in e-Learning: A Literature Review,” Education Sciences, vol. 13, no. 12, pp. 1-27, 2023.
[CrossRef] [Google Scholar] [Publisher Link]
[8] Yazhong Si, Fan Yang, and Zhao Liu, “Sand Dust Image Visibility Enhancement Algorithm via Fusion Strategy,” Scientific Reports, vol. 12, pp. 1-13, 2022.
[CrossRef] [Google Scholar] [Publisher Link]
[9] Muhammad Shafiq, and Zhaoquan Gu, “Deep Residual Learning for Image Recognition: A Survey,” Applied Sciences, vol. 12, no. 18, pp. 1-43, 2022.
[CrossRef] [Google Scholar] [Publisher Link]
[10] Vinod Vasan et al., “Tire Condition Monitoring Using Transfer Learning-Based Deep Neural Network Approach,” Sensors, vol. 23, no. 4, pp. 1-18, 2023.
[CrossRef] [Google Scholar] [Publisher Link]
[11] C.V. Prasshanth, and V. Sugumaran, “Tire Wear Monitoring Using Feature Fusion and Cat Boost Classifier,” Artificial Intelligence Review, vol. 57, pp. 1-28, 2024.
[CrossRef] [Google Scholar] [Publisher Link]
[12] Shaofeng Wang, Jiangjiang Yin, and Zilong Zhou, “A Comprehensive Evaluation Method for Dust Pollution: Digital Image Processing and Deep Learning Approach,” Journal of Hazardous Materials, vol. 475, 2024.
[CrossRef] [Google Scholar] [Publisher Link]
[13] Kaiqi Huang et al., “A Real-Time Object Detecting and Tracking System for Outdoor Night Surveillance,” Pattern Recognition, vol. 41, no. 1, pp. 432-444, 2008.
[CrossRef] [Google Scholar] [Publisher Link]
[14] Kaiming He et al., “Deep Residual Learning for Image Recognition,” IEEE Conference on Computer Vision and Pattern Recognition, Las Vegas, pp. 770-778, 2016.
[CrossRef] [Google Scholar] [Publisher Link]
[15] Przemysław Dabek et al., “Measurement of Idlers Rotation Speed in Belt Conveyors Based on Image Data Analysis for Diagnostic Purposes,” Measurement, vol. 202, pp. 1-15, 2022.
[CrossRef] [Google Scholar] [Publisher Link]
[16] Daniel Becker et al., “Cost-Effective Camera based Ground Truth for Indoor Localization,” IEEE International Conference on Advanced Intelligent Mechatronics, Busan, Korea (South), pp. 885-890, 2015.
[CrossRef] [Google Scholar] [Publisher Link]
[17] Andreas Theissler et al., “Predictive Maintenance Enabled by Machine Learning: Use Cases and Challenges in the Automotive Industry,” Reliability Engineering & System Safety, vol. 215, pp. 1-21, 2021.
[CrossRef] [Google Scholar] [Publisher Link]
[18] Shih-Lin Lin et al., “Research on Tire Crack Detection Using Image Deep Learning Method,” Scientific Reports, vol. 13, pp. 1-17, 2023.
[CrossRef] [Google Scholar] [Publisher Link]
[19] Zhihe Wu, Zhi Jin, and Xiying Li “Two-Stage Progressive Learning for Vehicle Re-Identification in Variable Illumination Conditions,” Electronics, vol. 12, no. 24, pp. 1-17, 2023.
[CrossRef] [Google Scholar] [Publisher Link]
[20] A.A. Kassim, M.A. Mannan, and Zhu Mian, “Texture Analysis Methods for Tool Condition Monitoring,” Image and Vision Computing, vol. 25, no. 7, pp. 1080-1090, 2007.
[CrossRef] [Google Scholar] [Publisher Link]
[21] Hoanh Nguyen, Tuan Anh Nguyen, and Nguyen Duc Toan, “Optimizing Feature Extraction and Fusion for High-Resolution Defect Detection in Solar Cells,” Intelligent Systems with Applications, vol. 24, pp. 1-12, 2024.
[CrossRef] [Google Scholar] [Publisher Link]
[22] Zhenyue Wang et al., “Foreign-Object Detection in High-Voltage Transmission Line Based on Improved YOLOv8m,” Applied Sciences, vol. 13, no. 23, pp. 1-22, 2023.
[CrossRef] [Google Scholar] [Publisher Link]