Emerging Trends in Lung Cancer Detection: Multiomics and Deep Learning Synergy
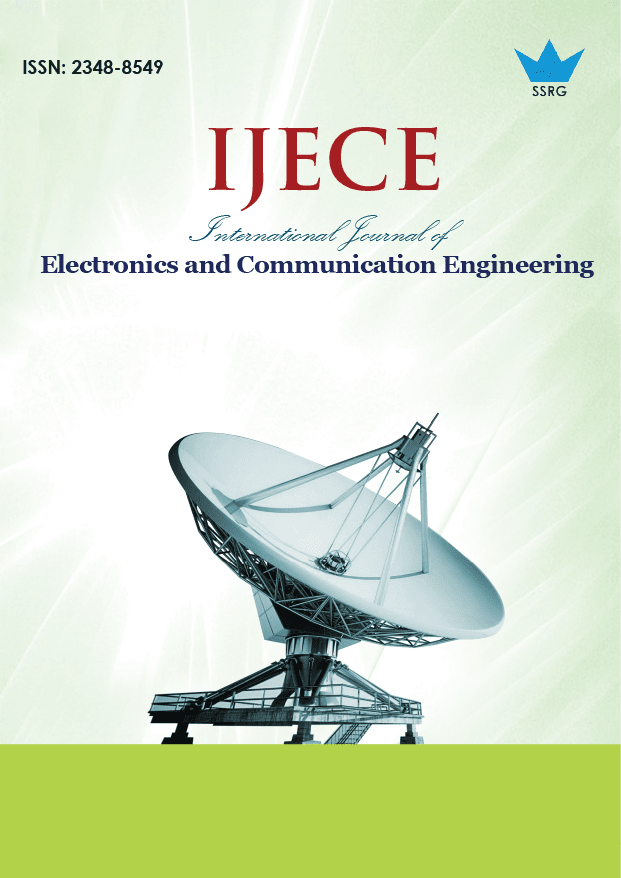
International Journal of Electronics and Communication Engineering |
© 2025 by SSRG - IJECE Journal |
Volume 12 Issue 3 |
Year of Publication : 2025 |
Authors : Nrupal Sankpal, Sandeep Musale, Supriya Mangale |
How to Cite?
Nrupal Sankpal, Sandeep Musale, Supriya Mangale, "Emerging Trends in Lung Cancer Detection: Multiomics and Deep Learning Synergy," SSRG International Journal of Electronics and Communication Engineering, vol. 12, no. 3, pp. 161-170, 2025. Crossref, https://doi.org/10.14445/23488549/IJECE-V12I3P116
Abstract:
Lung cancer remains the leading cause of cancer-related deaths worldwide, with Lung Adenocarcinoma (LUAD) accounting for a significant portion of non-small cell lung cancer cases. Early detection and accurate prognosis prediction are critical for improving treatment outcomes. Recent advancements in multi-omics data integration, including genomics, transcriptomics, and histopathology, have shown promise in enhancing the prediction accuracy for LUAD. Lung cancer detection benefits significantly from machine learning models trained on multi-omics datasets, including gene expression, methylation, and mutations. Techniques such as Random Forest, SVM, and GLM have been employed to achieve robust prediction outcomes. The contribution of features was further analyzed using SHAP values. In particular, models such as LungDWM, which uses Generative Adversarial Networks (GANs) and attention-based feature encoders, have shown superior performance in diagnosing LUAD subtypes and predicting patient outcomes. This review explores the application of multiomics approaches, such as identifying key prognostic genes and developing machine learning models, to improve survival prediction and cancer staging while highlighting the current state of research, challenges, and future directions in using multi-omics for lung cancer detection and prognosis.
Keywords:
Lung cancer, Machine Learning, Multiomics, Oncology.
References:
[1] Roman Jaksik et al., “Multiomics-Based Feature Extraction and Selection for the Prediction of Lung Cancer Survival,” International Journal of Molecular Sciences, vol. 25, no. 7, pp. 1-17, 2024.
[CrossRef] [Google Scholar] [Publisher Link]
[2] Xingze Wang et al., “Lung Cancer Subtype Diagnosis Using Weakly-Paired Multi-omics Data,” Bioinformatics, vol. 38, no. 22, pp. 5092-5099, 2022.
[CrossRef] [Google Scholar] [Publisher Link]
[3] Eskezeia Yihunie Dessie, Jan-Gowth Chang, and Ya-Sian Chang, “A Nine-Gene Signature Identification and Prognostic Risk Prediction for Patients with Lung Adenocarcinoma using Novel Machine Learning Approach,” Computers in Biology and Medicine vol. 145, 2022.
[CrossRef] [Google Scholar] [Publisher Link]
[4] Surong Zhang et al., “Identification of Seven-Gene Marker to Predict the Survival of Patients with Lung Adenocarcinoma using Integrated Multi-Omics Data Analysis,” Journal of Clinical Laboratory Analysis, vol. 36, no. 2, pp. 1-14, 2022.
[CrossRef] [Google Scholar] [Publisher Link]
[5] C. Anil Kumar et al., “[Retracted] Lung Cancer Prediction from Text Datasets Using Machine Learning,” BioMed Research International, vol. 2022, pp. 1-10, 2022.
[CrossRef] [Google Scholar] [Publisher Link]
[6] Wei Li et al., “[Retracted] Lung Cancer Stage Prediction Using Multi-Omics Data,” Computational and Mathematical Methods in Medicine, vol. 2022, pp. 1-10, 2022.
[CrossRef] [Google Scholar] [Publisher Link]
[7] Suli Liu, and Wu Yao, “Prediction of Lung Cancer using Gene Expression and Deep Learning with KL Divergence Gene Selection,” BMC Bioinformatics, vol. 23, pp. 1-11, 2022.
[CrossRef] [Google Scholar] [Publisher Link]
[8] Douglas Hanahan, “Hallmarks of Cancer: New Dimensions,” Cancer Discovery, vol. 12, no. 1, pp. 31-46, 2022.
[CrossRef] [Google Scholar] [Publisher Link]
[9] ZHU Rong et al., “Diagnostic Classification of Lung Cancer Using Deep Transfer Learning Technology and Multi-Omics Data,” Chinese Journal of Electronics, vol. 30, no. 5, pp. 843-852, 2022.
[CrossRef] [Google Scholar] [Publisher Link]
[10] Ying Xie et al., “Early Lung Cancer Diagnostic Biomarker Discovery by Machine Learning Methods,” Translational Oncology, vol. 14, no. 1, pp. 1-10, 2021.
[CrossRef] [Google Scholar] [Publisher Link]
[11] Linyan Chen et al., “Histopathological Images and Multi-omics Integration Predict Molecular Characteristics and Survival in Lung Adenocarcinoma,” Frontiers in Cell and Developmental Biology, vol. 9, pp. 1-13, 2021.
[CrossRef] [Google Scholar] [Publisher Link]
[12] Aditya Dubey, and Akhtar Rasool, “Efficient Technique of Microarray Missing Data Imputation using Clustering and Weighted Nearest Neighbour,” Scientific Reports, vol. 11, pp. 1-12, 2021.
[CrossRef] [Google Scholar] [Publisher Link]
[13] Xinshan Zhu et al., “An Efficient Ensemble Method for Missing Value Imputation in Microarray Gene Expression Data,” BMC Bioinformatics, vol. 22, pp. 1-25, 2021.
[CrossRef] [Google Scholar] [Publisher Link]
[14] Mingyuan Luan et al., “Multi-omics Integrative Analysis and Survival Risk Model Construction of Non-Small Cell Lung Cancer Based on the Cancer Genome Atlas Datasets,” Oncology Letters, vol. 20, no. 4, pp. 1-13, 2020.
[CrossRef] [Google Scholar] [Publisher Link]
[15] Baoshan Ma et al., “Identification of a Sixteen-gene Prognostic Biomarker for Lung Adenocarcinoma using a Machine Learning Method,” Journal of Cancer, vol. 11, no. 5, pp. 1288-1298, 2020.
[Google Scholar] [Publisher Link]
[16] Tzong-Yi Lee et al., “Incorporating Deep Learning and Multi-omics Autoencoding for Analysis of Lung Adenocarcinoma Prognostication,” Computational Biology and Chemistry, vol. 87, 2020.
[CrossRef] [Google Scholar] [Publisher Link]
[17] Halil Ibrahim Kuru, Oznur Tastan, and A. Ercument Cicek, “MatchMaker: A Deep Learning Framework for Drug Synergy Prediction,” IEEE/ACM Transactions on Computational Biology and Bioinformatics, vol. 19, no. 4, pp. 2334-2344, 2022.
[CrossRef] [Google Scholar] [Publisher Link]
[18] Tongxin Wang et al., “MOGONET Integrates Multi-Omics Data using Graph Convolutional Networks Allowing Patient Classification and Biomarker Identification,” Nature Communications, vol. 12, pp. 1-13, 2021.
[CrossRef] [Google Scholar] [Publisher Link]
[19] Xiaohan Xing et al., “An Interpretable Multi-Level Enhanced Graph Attention Network for Disease Diagnosis with Gene Expression Data,” IEEE International Conference on Bioinformatics and Biomedicine (BIBM), Houston, TX, USA, 556-561, 2021.
[CrossRef] [Google Scholar] [Publisher Link]
[20] Muta Tah Hira et al., “Integrated Multi-omics Analysis of Ovarian Cancer using Variational Autoencoders,” Scientific Reports, vol. 11, pp. 1-16, 2021.
[CrossRef] [Google Scholar] [Publisher Link]
[21] Md. Mohaiminul Islam et al., “An Integrative Deep Learning Framework for Classifying Molecular Subtypes of Breast Cancer,” Computational and Structural Biotechnology Journal, vol. 18, pp. 2185-2199, 2020.
[CrossRef] [Google Scholar] [Publisher Link]
[22] Yuhua Fu et al., “A Gene Prioritization Method Based on a Swine Multi-Omics Knowledgebase and a Deep Learning Model. Communications Biology, vol. 3, no. 1, pp. 1-11, 2020.
[CrossRef] [Google Scholar] [Publisher Link]
[23] Long-Yi Guo et al., “Deep Learning-Based Ovarian Cancer Subtypes Identification using Multi-Omics Data,” BioData Mining, vol. 13, pp. 1-12, 2020.
[CrossRef] [Google Scholar] [Publisher Link]
[24] Tianle Ma, and Aidong Zhang, “Integrate Multi-omics Data with Biological Interaction Networks using Multi-View Factorization Autoencoder (MAE),” BMC Genomics, vol. 20, pp. 1-11, 2019.
[CrossRef] [Google Scholar] [Publisher Link]
[25] Xiaoyu Zhang et al., “Integrated Multiomics Analysis using Variational Autoencoders: Application to Pan-Cancer Classification,” IEEE International Conference on Bioinformatics and Biomedicine (BIBM), San Diego, CA, USA, pp. 765-769, 2019.
[CrossRef] [Google Scholar] [Publisher Link]
[26] Jonathan Ronen, Sikander Hayat, and Altuna Akalin, “Evaluation of Colorectal Cancer Subtypes and Cell Lines using Deep Learning,” Life Science Alliance, vol. 2, no. 6, pp. 1-16, 2019.
[CrossRef] [Google Scholar] [Publisher Link]
[27] Kristina Preuer et al., “DeepSynergy: Predicting Anti-Cancer Drug Synergy with Deep Learning,” Bioinformatics, vol. 34, no. 9, 1538-1546, 2018.
[CrossRef] [Google Scholar] [Publisher Link]
[28] Olivier B. Poirion, Kumardeep Chaudhary, and Lana X. Garmire, “Deep Learning Data Integration for Better Risk Stratification Models of Bladder Cancer,” AMIA Joint Summits on Translational Science Proceedings, pp. 197-206, 2018.
[CrossRef] [Google Scholar] [Publisher Link]