NACDN: A Novel Approach for Noise Intensity Estimation and Adaptive Denoising in Medical Images
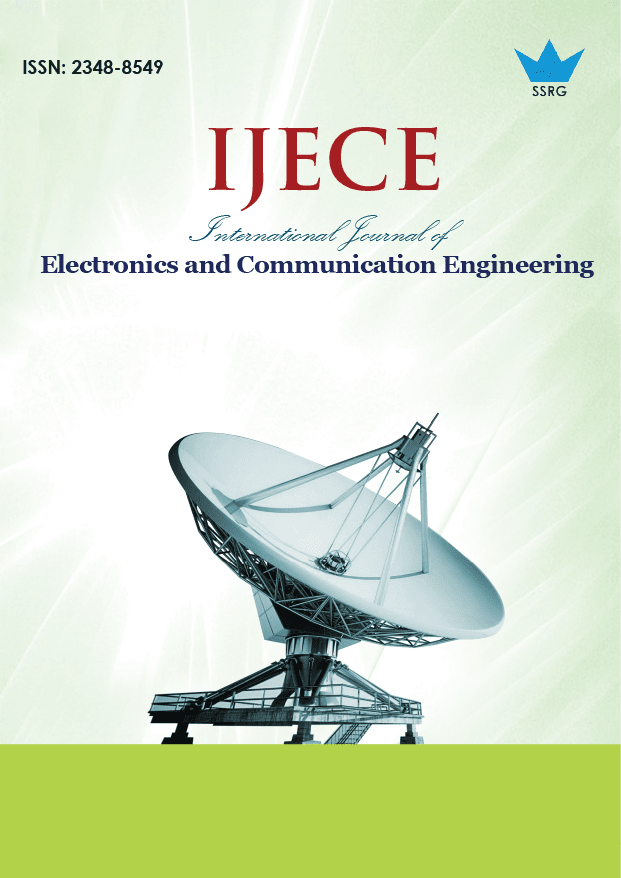
International Journal of Electronics and Communication Engineering |
© 2025 by SSRG - IJECE Journal |
Volume 12 Issue 3 |
Year of Publication : 2025 |
Authors : Maddimsetty Surya Prakash, Tamilselvan Sadasivam |
How to Cite?
Maddimsetty Surya Prakash, Tamilselvan Sadasivam, "NACDN: A Novel Approach for Noise Intensity Estimation and Adaptive Denoising in Medical Images," SSRG International Journal of Electronics and Communication Engineering, vol. 12, no. 3, pp. 190-201, 2025. Crossref, https://doi.org/10.14445/23488549/IJECE-V12I3P119
Abstract:
Image denoising is a crucial task in digital image processing, aiming to remove unwanted noise while preserving important medical image details. The Noise-Adaptive Convolutional Denoising Network (NACDN) presents an innovative methodology for addressing the challenge of medical image noise. In this work, we propose a novel deep learning framework designed to estimate the intensity or level of noise present in medical images while facilitating adaptive and precise denoising strategies tailored to the specific characteristics of each image. NACDN comprises three main modules: a Noise Estimation Module, a Noise Classification Module, and an Adaptive Denoising Module. Leveraging convolutional neural networks, NACDN accurately estimates the noise intensity in medical images and categorizes noise types, enabling targeted denoising approaches. The Adaptive Denoising Module applies denoising algorithms specific to the estimated noise characteristics, ensuring optimal noise reduction while preserving image details. Through extensive experiments and evaluations, NACDN demonstrates superior performance in enhancing image quality by effectively reducing noise artifacts. This research introduces a significant advancement in image processing, offering a robust solution for noise estimation and adaptive denoising in diverse imaging applications.
Keywords:
Image denoising, Noise estimation, Adaptive denoising, Convolutional Neural Networks, Deep Learning.
References:
[1] Ravi Ranjan Kumar, and Rahul Priyadarshi, “Denoising and Segmentation in Medical Image Analysis: A Comprehensive Review on Machine Learning and Deep Learning Approaches,” Multimedia Tools and Applications, 2024.
[CrossRef] [Google Scholar] [Publisher Link]
[2] Rajesh Patil, and Surendra Bhosale, “Medical Image Denoising Techniques: A Review,” International Journal on Engineering, Science and Technology, vol. 4, no. 1, pp. 21-33, 2022.
[CrossRef] [Google Scholar] [Publisher Link]
[3] Mufeng Geng et al., “Content-Noise Complementary Learning for Medical Image Denoising,” IEEE Transactions on Medical Imaging, vol. 41, no. 2, pp. 407-419, 2022.
[CrossRef] [Google Scholar] [Publisher Link]
[4] Guohui Li, Wenjia Bu, and Hong Yang, “Research on Noise Reduction Method for Ship Radiate Noise Based on Secondary Decomposition,” Ocean Engineering, vol. 268, 2023.
[CrossRef] [Google Scholar] [Publisher Link]
[5] A. Velayudham, K. Madhan Kumar, and Krishna Priya M.S., “Enhancing Clinical Diagnostics: Novel Denoising Methodology for Brain MRI with Adaptive Masking and Modified Non-local Block,” Medical & Biological Engineering & Computing, vol. 62, pp. 3043-3056, 2024.
[CrossRef] [Google Scholar] [Publisher Link]
[6] Xiaoning Liu et al., “NTIRE 2024 Challenge on Low Light Image Enhancement: Methods and Results,” Conference on Computer Vision and Pattern Recognition Workshops, Seattle, WA, USA, pp. 6571-6594, 2024.
[CrossRef] [Google Scholar] [Publisher Link]
[7] Furkan Kirik et al., “Quantitative Analysis of Deep Learning-Based Denoising Model Efficacy on Optical Coherence Tomography Images with Different Noise Levels,” Photodiagnosis and Photodynamic Therapy, vol. 45, pp. 1-6, 2024.
[CrossRef] [Google Scholar] [Publisher Link]
[8] Prabhishek Singh et al., “A Method Noise-Based Convolutional Neural Network Technique for CT Image Denoising,” Electronics, vol. 11, no. 21, pp. 1-19, 2022.
[CrossRef] [Google Scholar] [Publisher Link]
[9] Veeraraghava Raju Hasti, and Dongyun Shin, “Denoising and Fuel Spray Droplet Detection from Light-scattered Images Using Deep Learning,” Energy and AI, vol. 7, pp. 1-10, 2022.
[CrossRef] [Google Scholar] [Publisher Link]
[10] Rini Smita Thakur, Ram Narayan Yadav, and Lalita Gupta, “State‐of‐art Analysis of Image Denoising Methods Using Convolutional Neural Networks,” IET Image Processing, vol. 13, no. 13, pp. 2367-2380, 2019.
[CrossRef] [Google Scholar] [Publisher Link]
[11] Poonam Pawar et al., “Deep Learning Approach for the Detection of Noise Type in Ancient Images,” Sustainability, vol. 14, no. 18, pp. 1-19, 2022.
[CrossRef] [Google Scholar] [Publisher Link]
[12] Zhuxiang Shen, Wei Li, and Hui Han, “Deep Learning‐Based Wavelet Threshold Function Optimization on Noise Reduction in Ultrasound Images,” Scientific Programming, vol. 2021, no. 1, pp. 1-8, 2021.
[CrossRef] [Google Scholar] [Publisher Link]
[13] Asavaron Limsuebchuea, Rakkrit Duangsoithong, and Pornchai Phukpattaranont, “Self-Augmented Noisy Image for Noise2Noise Image Denoising,” IEEE Access, vol. 12, pp. 71076-71087, 2024.
[CrossRef] [Google Scholar] [Publisher Link]
[14] Yang Shao, Toshie Yaguchi, and Toshiaki Tanigaki, “Denoising Low-Dose Images Using Deep Learning of Time Series Images,” Arxiv, pp. 1-16, 2024.
[CrossRef] [Google Scholar] [Publisher Link]
[15] Walid El-Shafai et al., “Traditional and Deep-Learning-Based Denoising Methods for Medical Images,” Multimedia Tools and Applications, vol. 83, pp. 52061-52088, 2024.
[CrossRef] [Google Scholar] [Publisher Link]
[16] Bin Qiu et al., “Comparative Study of Deep Neural Networks with Unsupervised Noise2Noise Strategy for Noise Reduction of Optical Coherence Tomography Images,” Journal of Biophotonics, vol. 14, no. 11, 2021.
[CrossRef] [Google Scholar] [Publisher Link]
[17] Alexander Krull et al., “Image Denoising and The Generative Accumulation of Photons,” Proceedings of the IEEE/CVF Winter Conference on Applications of Computer Vision, pp. 1528-1537, 2024.
[Google Scholar] [Publisher Link]
[18] Inpyo Hong, Youngbae Hwang, and Daeyoung Kim, “Efficient Deep Learning of Image Denoising Using Patch Complexity Local Divide and Deep Conquer,” Pattern Recognition, vol. 96, 2019.
[CrossRef] [Google Scholar] [Publisher Link]
[19] Junshen Xu, and Elfar Adalsteinsson, “Deformed2self: Self-supervised Denoising for Dynamic Medical Imaging,” Medical Image Computing and Computer Assisted Intervention, pp. 25-35, 2021.
[CrossRef] [Google Scholar] [Publisher Link]
[20] Miral S. Tawfik et al., “Comparative Study of Traditional and Deep-learning Denoising Approaches for Image-based Petrophysical Characterization of Porous Media,” Frontiers in Water, vol. 3, pp. 1-23, 2021.
[CrossRef] [Google Scholar] [Publisher Link]
[21] Saikiran Pendem et al., “Influence of Deep Learning Image Reconstruction Algorithm for Reducing Radiation Dose and Image Noise Compared to Iterative Reconstruction and Filtered Back Projection for Head and Chest Computed Tomography Examinations: A Systematic Review,” F1000Research, pp. 1-21, 2024.
[CrossRef] [Google Scholar] [Publisher Link]
[22] Muhammad Zubair et al., “Enabling Predication of the Deep Learning Algorithms for Low-Dose CT Scan Image Denoising Models: A Systematic Literature Review,” IEEE Access, vol. 12, pp. 79025-79050, 2024.
[CrossRef] [Google Scholar] [Publisher Link]
[23] Kai Wu et al., “Blind Deep-Learning Based Preprocessing Method for Fourier Ptychographic Microscopy,” Optics & Laser Technology, vol. 169, 2024.
[CrossRef] [Google Scholar] [Publisher Link]
[24] Zhongxing Zhou et al., “Image Quality Evaluation in Deep‐Learning‐Based CT Noise Reduction Using Virtual Imaging Trial Methods: Contrast‐Dependent Spatial Resolution,” Medical Physics, vol. 51, no. 8, pp. 5399-5413, 2024.
[CrossRef] [Google Scholar] [Publisher Link]
[25] Florence M. Muller et al., “Image Denoising of Low-Dose PET Mouse Scans with Deep Learning: Validation Study for Preclinical Imaging Applicability,” Molecular Imaging and Biology, vol. 26, pp. 101-113, 2024.
[CrossRef] [Google Scholar] [Publisher Link]