Intrusion Detection in Wireless Ad Hoc Networks Using Advanced Graph Theory Models
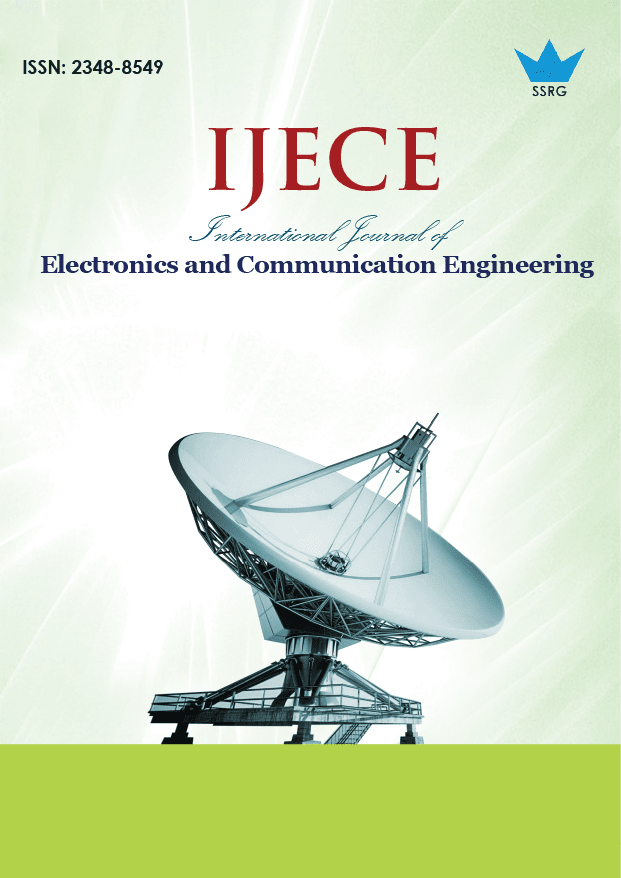
International Journal of Electronics and Communication Engineering |
© 2025 by SSRG - IJECE Journal |
Volume 12 Issue 3 |
Year of Publication : 2025 |
Authors : K. Sudharson, Rajesh Kambattan Kovarasan, N. Sathish Kumar, S. Rajalakshmi |
How to Cite?
K. Sudharson, Rajesh Kambattan Kovarasan, N. Sathish Kumar, S. Rajalakshmi, "Intrusion Detection in Wireless Ad Hoc Networks Using Advanced Graph Theory Models," SSRG International Journal of Electronics and Communication Engineering, vol. 12, no. 3, pp. 202-209, 2025. Crossref, https://doi.org/10.14445/23488549/IJECE-V12I3P120
Abstract:
Our proposed system, described in this paper, focuses on intrusion detection in WANETs, where there are emerging complexities in the structure of the graph models and the number of attributes of a node from which information must be extracted or aggregated. Attacks on WANETs are mostly abrupt, and that calls for systems that can capture fluctuations in topology. Real-time identification of such changes is achieved through our dynamic graph transformations using variational inequalities as the modeling vehicle. The novel model under consideration is Matrix Completion-GCN with nonlinear activation functions and Variational Autoencoders (VAEs) to improve the efficiency of graph representation learning. Moreover, Spectral Signal Clustering (SSC) is used in the process as a prescreening form to filter raw data and prettify the graph signal. The results evaluated on several real-world WANET datasets show that the proposed model has much higher performance compared to the traditional models like SVM and RF, giving 95.5% precision, 97.2 % recall, 98.1% detection rate and 1.2% false positive. This research provides high accuracy for real-time intrusion detection, and future research can be done to optimize computational cost and broaden its scope for IoT networks.
Keywords:
Wireless Ad Hoc Networks, Intrusion detection, Graph-based classification, Spectral graph preprocessing, Nonlinear variational inequalities.
References:
[1] Shiwen He et al., “An Overview on the Application of Graph Neural Networks in Wireless Networks,” IEEE Open Journal of the Communications Society, vol. 2, pp. 2547-2565, 2021.
[CrossRef] [Google Scholar] [Publisher Link]
[2] Aabid A. Mir et al., “Variational Graph Convolutional Networks for Dynamic Graph Representation Learning,” IEEE Access, vol. 12, pp. 161697-161717, 2024.
[CrossRef] [Google Scholar] [Publisher Link]
[3] Xiaofeng Zhao et al., “A Review of Hyperspectral Image Classification Based on Graph Neural Networks,” Artificial Intelligence Review, vol. 58, no. 6, 2025.
[CrossRef] [Google Scholar] [Publisher Link]
[4] Sandeep Singh Sengar et al., “Generative Artificial Intelligence: A Systematic Review and Applications,” Multimedia Tools and Applications, 2024.
[CrossRef] [Google Scholar] [Publisher Link]
[5] Patrick L. Combettes, and Zev C. Woodstock, “A Variational Inequality Model for the Construction of Signals from Inconsistent Nonlinear Equations,” SIAM Journal on Imaging Sciences, vol. 15, no. 1, pp. 84-109, 2022.
[CrossRef] [Google Scholar] [Publisher Link]
[6] V. Senthil Murugan, and Bhuvan Unhelkar, “Optimizing Mobile Ad Hoc Network Cluster Based Routing: Energy Prediction Via Improved Deep Learning Technique,” International Journal of Communication Systems, vol. 37, no. 10, 2024.
[CrossRef] [Google Scholar] [Publisher Link]
[7] Giuseppe Granato et al., “Graph-Based Multi-Label Classification for WiFi Network Traffic Analysis,” Applied Sciences, vol. 12, no. 21, pp. 1-22, 2022.
[CrossRef] [Google Scholar] [Publisher Link]
[8] Aristidis G. Vrahatis, Konstantinos Lazaros, and Sotiris Kotsiantis, “Graph Attention Networks: A Comprehensive Review of Methods and Applications,” Future Internet, vol. 16, no. 1, pp. 1-34, 2024.
[CrossRef] [Google Scholar] [Publisher Link]
[9] Usama Ahmed et al., “Signature-Based Intrusion Detection Using Machine Learning and Deep Learning Approaches Empowered with Fuzzy Clustering,” Scientific Reports, vol. 15, no. 1, pp. 1-33, 2025.
[CrossRef] [Google Scholar] [Publisher Link]
[10] H.S. Manjula et al., “Intrusion Detection Model for IoT Networks Using Graph Convolution Networks (GCN),” Smart Innovation, Systems and Technologies, ICT for Intelligent Systems, Singapore, vol. 361, pp. 1-12, 2023.
[CrossRef] [Google Scholar] [Publisher Link]
[11] Zhongnan Qu et al., “DRESS: Dynamic Real-Time Sparse Subnets,” arXiv Preprints, 2022.
[CrossRef] [Google Scholar] [Publisher Link]
[12] Hamza Kheddar et al., “Reinforcement-Learning-Based Intrusion Detection in Communication Networks: A Review,” IEEE Communications Surveys & Tutorials, 2024.
[CrossRef] [Google Scholar] [Publisher Link]
[13] Marco Procaccini, Amin Sahebi, and Roberto Giorgi, “A Survey of Graph Convolutional Networks (GCNs) in FPGA-Based Accelerators,” Journal of Big Data, vol. 11, no. 1, 2024.
[CrossRef] [Google Scholar] [Publisher Link]
[14] Haochen Xu et al., “A Novel Approach for Detecting Malicious Hosts Based on RE-GCN in Intranet,” Cybersecurity, vol. 7, no. 1, 2024.
[CrossRef] [Google Scholar] [Publisher Link]
[15] Rongjun Chen et al., “An Optimized Lightweight Real-Time Detection Network Model for IoT Embedded Devices,” Scientific Reports, vol. 15, no. 1, 2025.
[CrossRef] [Google Scholar] [Publisher Link]
[16] Jizhao Liu, and Minghao Guo, “DIGNN-A: Real-Time Network Intrusion Detection with Integrated Neural Networks Based on Dynamic Graph,” Computers, Materials & Continua, vol. 82, no. 1, pp. 817-842, 2025.
[CrossRef] [Google Scholar] [Publisher Link]
[17] S. Saravanan, “Deep Learning Models for Intrusion Detection Systems in MANETs: A Comparative Analysis,” Decision Making Advances, vol. 3, no. 1, pp. 96-110, 2025.
[CrossRef] [Google Scholar] [Publisher Link]