Predictive Modelling for Cardiovascular Disease Identification and Early Disease Detection Using Gradient Boosting Machines (GBM) Model
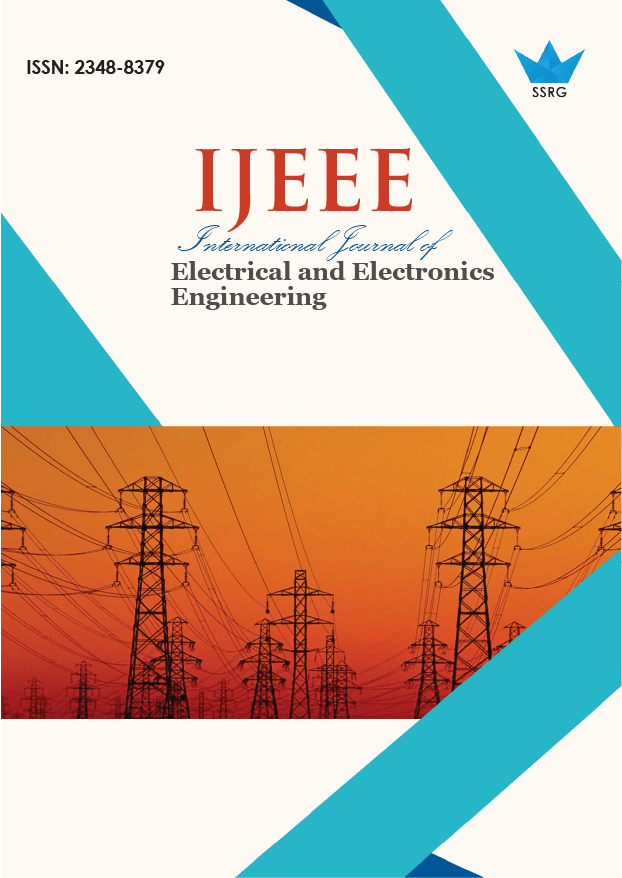
International Journal of Electrical and Electronics Engineering |
© 2025 by SSRG - IJEEE Journal |
Volume 12 Issue 3 |
Year of Publication : 2025 |
Authors : Anthani Kamala Priya, Bhavani Madireddy |
How to Cite?
Anthani Kamala Priya, Bhavani Madireddy, "Predictive Modelling for Cardiovascular Disease Identification and Early Disease Detection Using Gradient Boosting Machines (GBM) Model," SSRG International Journal of Electrical and Electronics Engineering, vol. 12, no. 3, pp. 1-12, 2025. Crossref, https://doi.org/10.14445/23488379/IJEEE-V12I3P101
Abstract:
CVD remains a global health concern. Early and accurate prediction is crucial for the prevention of treatments as well as better patient outcomes. In classification, Cardiovascular Disease (CVD) is identified using machine learning algorithms that analyze and predict if an individual will have CVD from a collection of medical data. The suggested process is comprised of several valuable steps. To ensure data completeness, imputation techniques are first used to fill in the missing values. However, numerical features are then scaled in order to improve model performance and convergence. Categorical variables are encoded to numerical representations to prevent some biases and preserve the informativeness of the variables. Finally, feature selection approaches are used to find the most instructive qualities of the models in order to improve their interpretability and efficiency. Machine learning is used to identify CVD. These algorithms were also proposed to use a dataset closer to real-time cases. The model is well-trained based on available historical data. To the model, it taught the patterns in the data. The metrics were then generated to find the model's proposed performance. The paper also proposes the preferred method of CVD prediction. The classification has always been done so that GBMs are the accurate method. Overall, the main intention is to develop a reliable model of accurate disease of the patients at risk. BM processes the categorical variables like smoking status and gender and numerical values such as age and blood pressure. The dataset considered is closer to the practical scenario for predicting CVD. The attribute's contribution when predicting the disease will be considered for each tree in the ensemble to try and learn more about the attributes with the strongest attributes in predicting the disease. The paper defines the prediction accuracy of CVD well. The real-time dataset is input to the model, and improved model accuracy is achieved by modifying the Gradient Boosting Machines GBMs. The proposed GBM model was evaluated in terms of performance, and it was found to outperform traditional classification models such as logistic regression by [percentage] in terms of predictiveness. Further validation of the model in predicting high-risk patients is achieved through sensitivity, specificity, and precision-recall curves. However, this technique could potentially reduce the burden of CVD by enabling healthcare practitioners to receive important insights that help reduce the risk of CVD and accurate risk assessment. Analysis of the primary variables driving the predictions adds insight into the clinical information beyond risk assessment that can be derived from the model. With this work, we contribute towards the effort of improving the management of cardiovascular health through artificial intelligence.
Keywords:
Early disease detection, Feature extraction, Machine Learning, Gradient Boosting Machines (GBM), Cardio Vascular Design (CVD).
References:
[1] Huazhong Yang et al., “Predicting Coronary Heart Disease Using an Improved Light GBM Model: Performance Analysis and Comparison,” IEEE Access, vol. 11, pp. 23366-23380, 2023.
[CrossRef] [Google Scholar] [Publisher Link]
[2] Gamal G.N. Geweid, and Mahmoud A. Abdallah, “A New Automatic Identification Method of Heart Failure Using Improved Support Vector Machine Based on Duality Optimization Technique,” IEEE Access, vol. 7, pp. 149595-149611, 2019.
[CrossRef] [Google Scholar] [Publisher Link]
[3] Hassan Nahas et al., “Artificial-Intelligence-Enhanced Ultrasound Flow Imaging at the Edge,” IEEE Micro, vol. 42, no. 6, pp. 96-106, 2022.
[CrossRef] [Google Scholar] [Publisher Link]
[4] Mohanad Alkhodari, Leontios J. Hadjileontiadis, and Ahsan H. Khandoker, “Identification of Congenital Valvular Murmurs in Young Patients Using Deep Learning-Based Attention Transformers and Phonocardiograms,” IEEE Journal of Biomedical and Health Informatics, vol. 28, no. 4, pp. 1803-1814, 2024.
[CrossRef] [Google Scholar] [Publisher Link]
[5] Kainat Zafar et al., “Deep Learning-Based Feature Engineering to Detect Anterior and Inferior Myocardial Infarction Using UWB Radar Data,” IEEE Access, vol. 11, pp. 97745-97757, 2023.
[CrossRef] [Google Scholar] [Publisher Link]
[6] Jingbo Wang, “OCT Image Recognition of Cardiovascular Vulnerable Plaque Based on CNN,” IEEE Access, vol. 8, pp. 140767-140776, 2020.
[CrossRef] [Google Scholar] [Publisher Link]
[7] Jing Zhang et al., “MLBF-Net: A Multi-Lead-Branch Fusion Network for Multi-Class Arrhythmia Classification Using 12-Lead ECG,” IEEE Journal of Translational Engineering in Health and Medicine, vol. 9, pp. 1-11, 2021.
[CrossRef] [Google Scholar] [Publisher Link]
[8] Børge Solli Andreassen et al., “Mitral Annulus Segmentation and Anatomical Orientation Detection in TEE Images Using Periodic 3D CNN,” IEEE Access, vol. 10, pp. 51472-51486, 2022.
[CrossRef] [Google Scholar] [Publisher Link]
[9] Müjde Akdeniz et al., “Deep Learning for Multi-Level Detection and Localization of Myocardial Scars Based on Regional Strain Validated on Virtual Patients,” IEEE Access, vol. 11, pp. 15788-15798, 2023.
[CrossRef] [Google Scholar] [Publisher Link]
[10] Fatemeh Taheri Dezaki et al., “Echo-SyncNet: Self-Supervised Cardiac View Synchronization in Echocardiography,” IEEE Transactions on Medical Imaging, vol. 40, no. 8, pp. 2092-2104, 2021.
[CrossRef] [Google Scholar] [Publisher Link]
[11] Jia Li et al., “Arrhythmia Classification Using Biased Dropout and Morphology-Rhythm Feature with Incremental Broad Learning,” IEEE Access, vol. 9, pp. 66132-66140, 2021.
[CrossRef] [Google Scholar] [Publisher Link]
[12] Jingshan Huang et al., “ECG Arrhythmia Classification Using STFT-Based Spectrogram and Convolutional Neural Network,” IEEE Access, vol. 7, pp. 92871-92880, 2019.
[CrossRef] [Google Scholar] [Publisher Link]
[13] Li Ping Shi et al., “Efficient Graphene Reconfigurable Reflectarray Antenna Electromagnetic Response Prediction Using Deep Learning,” IEEE Access, vol. 9, pp. 22671-22678, 2020.
[CrossRef] [Google Scholar] [Publisher Link]
[14] Ananth Bhoj, Ron Kinder, and Larry Gochberg, “Numerical Calculations on the Low-Pressure Behavior of a High-Density Plasma CVD Reactor,” IEEE 34th International Conference on Plasma Science, Albuquerque, NM, USA, pp. 568-568, 2007.
[CrossRef] [Google Scholar] [Publisher Link]
[15] Yun Kwan Kim et al., “Automatic Cardiac Arrhythmia Classification Using Residual Network Combined with Long Short-Term Memory,” IEEE Transactions on Instrumentation and Measurement, vol. 71, pp. 1-17, 2022.
[CrossRef] [Google Scholar] [Publisher Link]
[16] Liang-Hung Wang et al., “Three-Heartbeat Multilead ECG Recognition Method for Arrhythmia Classification,” IEEE Access, vol. 10, pp. 44046-44061, 2022.
[CrossRef] [Google Scholar] [Publisher Link]
[17] Runnan He et al., “Automatic Cardiac Arrhythmia Classification Using Combination of Deep Residual Network and Bidirectional LSTM,” IEEE Access, vol. 7, pp. 102119-102135, 2019.
[CrossRef] [Google Scholar] [Publisher Link]
[18] Hui Yang, and Zhiqiang Wei, “Arrhythmia Recognition and Classification Using Combined Parametric and Visual Pattern Features of ECG Morphology,” IEEE Access, vol. 8, pp. 47103-47117, 2020.
[CrossRef] [Google Scholar] [Publisher Link]
[19] G.S. Nijaguna et al., “Feature Selection Using Selective Opposition Based Artificial Rabbits Optimization for Arrhythmia Classification on Internet of Medical Things Environment,” IEEE Access, vol. 11, pp. 100052-100069, 2023.
[CrossRef] [Google Scholar] [Publisher Link]
[20] Classification,” IEEE Transactions on Consumer Electronics, vol. 69, no. 3, pp. 250-260, 2023.
[CrossRef] [Google Scholar] [Publisher Link]
[21] Maytham N. Meqdad, Fardin Abdali-Mohammadi, and Seifedine Kadry, “Meta Structural Learning Algorithm with Interpretable Convolutional Neural Networks for Arrhythmia Detection of Multisession ECG,” IEEE Access, vol. 10, pp. 61410-61425, 2022.
[CrossRef] [Google Scholar] [Publisher Link]
[22] Tanvir Mahmud, Shaikh Anowarul Fattah, and Mohammad Saquib, “DeepArrNet: An Efficient Deep CNN Architecture for Automatic Arrhythmia Detection and Classification from Denoised ECG Beats,” IEEE Access, vol. 8, pp. 104788-104800, 2020.
[CrossRef] [Google Scholar] [Publisher Link]
[23] Mariya R. Kiladze et al., “Multimodal Neural Network for Recognition of Cardiac Arrhythmias Based on 12-Load Electrocardiogram Signals,” IEEE Access, vol. 11, pp. 133744-133754, 2023.
[CrossRef] [Google Scholar] [Publisher Link]
[24] Ashley Spann et al., “Applying Machine Learning in Liver Disease and Transplantation: A Comprehensive Review,” Hepatology, vol. 71, no. 3, pp. 1093-1105, 2020.
[CrossRef] [Google Scholar] [Publisher Link]
[25] Yihao Liu, and Minghua Wu, “Deep Learning in Precision Medicine and Focus on Glioma,” BioEngineering and Translational Medicine, vol. 8, no. 5, pp. 1-21, 2023.
[CrossRef] [Google Scholar] [Publisher Link]
[26] Md Maruf Hossain et al., “Cardiovascular Disease Identification Using a Hybrid CNN-LSTM Model with Explainable AI,” Informatics in Medicine Unlocked, vol. 42, 2023.
[CrossRef] [Google Scholar] [Publisher Link]
[27] Agustin Martin-Morales et al., “Predicting Cardiovascular Disease Mortality: Leveraging Machine Learning for Comprehensive Assessment of Health and Nutrition Variables,” Nutrients, vol. 15, no. 18, pp. 1-13, 2023.
[CrossRef] [Google Scholar] [Publisher Link]
[28] Patricia Rufes et al., “Heart Disease Prediction Using Machine Learning,” International Research Journal on Advanced Engineering Hub, vol. 2 no. 3, pp. 485-490, 2024.
[CrossRef] [Publisher Link]
[29] Najmu Nissa, Sanjay Jamwal, and Mehdi Neshat, “A Technical Comparative Heart Disease Prediction Framework Using Boosting Ensemble Techniques,” Computation, vol. 12, no. 15, pp. 1-22, 2024.
[CrossRef] [Google Scholar] [Publisher Link]
[30] Yahia Baashar et al., “Effectiveness of Artificial Intelligence Models for Cardiovascular Disease Prediction: Network Meta-Analysis,” Computational Intelligence and Neuroscience, vol. 2022, no. 1, pp. 1-12, 2022.
[CrossRef] [Google Scholar] [Publisher Link]
[31] Adedayo Ogunpola et al., “Machine Learning-Based Predictive Models for Detection of Cardiovascular Diseases,” Diagnostics, vol. 14, no. 2. pp. 1-19, 2024.
[CrossRef] [Google Scholar] [Publisher Link]
[32] Jesse Gabriel, “A Machine Learning-Based Web Application for Heart Disease Prediction,” Intelligent Control and Automation, vol. 15, no. 1, pp. 9-27, 2024.
[CrossRef] [Google Scholar] [Publisher Link]
[33] K. Karthick et al., “[Retracted] Implementation of a Heart Disease Risk Prediction Model Using Machine Learning,” Computational and Mathematical Methods in Medicine, vol. 2023, no. 1, pp. 1-14, 2023.
[CrossRef] [Google Scholar] [Publisher Link]