Deep Learning-Based Techniques for Identification of Audio DeepFake with Open Issues: A Meta-Analysis
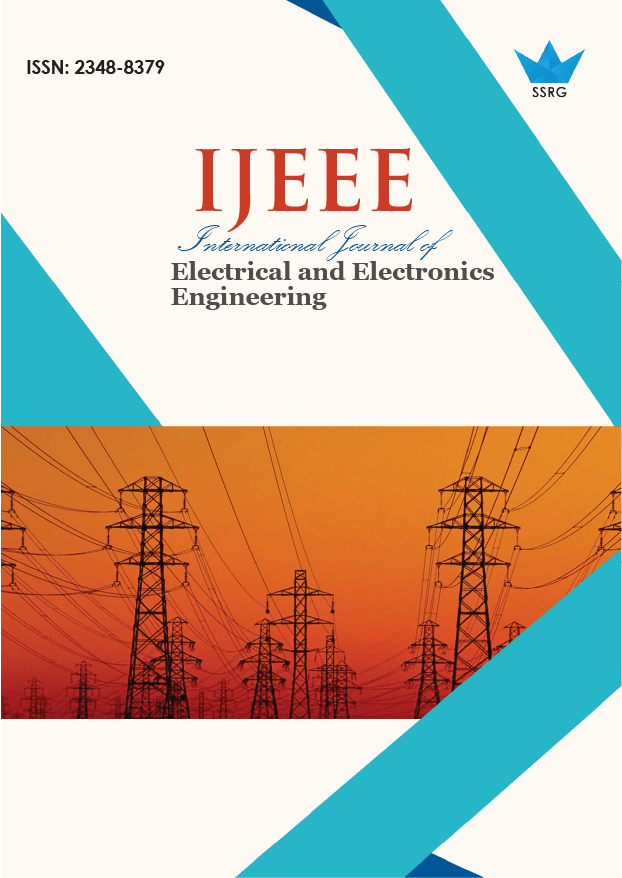
International Journal of Electrical and Electronics Engineering |
© 2025 by SSRG - IJEEE Journal |
Volume 12 Issue 3 |
Year of Publication : 2025 |
Authors : Krity Duhan, Abhishek Kajal |
How to Cite?
Krity Duhan, Abhishek Kajal, "Deep Learning-Based Techniques for Identification of Audio DeepFake with Open Issues: A Meta-Analysis," SSRG International Journal of Electrical and Electronics Engineering, vol. 12, no. 3, pp. 36-44, 2025. Crossref, https://doi.org/10.14445/23488379/IJEEE-V12I3P104
Abstract:
Amidst the ongoing advancements in artificial intelligence, a particularly fascinating and alarming progress is the rise of deepfake speech. The emergence of deepfake technology poses a substantial risk to national security, democratic systems, society as a whole and individual privacy. Consequently, it is imperative to create better methods for identifying and mitigating possible deepfake threats. Audio counterfeiting detection is a rapidly developing and important subject. A growing amount of literature is focused on studying deepfake detection computations, which have demonstrated successful results. However, it is important to note that the issue is still far from being completely settled. As synthetic voice generation technology improves, audio deepfake is growing as perhaps the most widespread form of deception. Therefore, the task of differentiating between counterfeit and authentic audio recordings is growing increasingly difficult. Hence, the significance of a system capable of promptly identifying genuine or deceptive audio cannot be exaggerated. In this paper, the evaluation of audio-based deepfake identification methods has been surveyed, and their comparative analysis is being done based on the dataset usage, metrics for evaluation like AUC, EER, a language considered for the dataset taken and the factors such as MFCC, and CQCC. Moreover, the open challenges and future research directions have been highlighted.
Keywords:
Audio, Deepfake, ASV spoof, GAN, Deep Learning, CNN, RNN, ResNet.
References:
[1] Lakshmanan Nataraj et al., “Detecting GAN Generated Fake Images Using Co-Occurrence Matrices,” arXiv Preprint, 2019.
[CrossRef] [Google Scholar] [Publisher Link]
[2] Sheng-Yu Wang et al., “CNN-Generated Images are Surprisingly Easy to Spot... for Now,” Proceedings of the IEEE/CVF Conference on Computer Vision and Pattern Recognition, Seattle, WA, USA, pp. 8695-8704, 2020.
[CrossRef] [Google Scholar] [Publisher Link]
[3] Chih-Chung Hsu, Chia-Yen Lee, and Yi-Xiu Zhuang, “Learning to Detect Fake Face Images in the Wild,” International Symposium on Computer, Consumer and Control, Taichung, Taiwan, pp. 388-391, 2018.
[CrossRef] [Google Scholar] [Publisher Link]
[4] Mehdi Mirza, and Simon Osindero, “Conditional Generative Adversarial Nets,” arXiv Preprint, 2014.
[CrossRef] [Google Scholar] [Publisher Link]
[5] Marwan Albahar, and Jameel Almalki, “Deepfakes: Threats and Countermeasures Systematic Review,” Journal of Theoretical and Applied Information Technology, vol. 97, no. 22, pp. 3242-3250, 2019.
[Google Scholar] [Publisher Link]
[6] Jonat John Mathew, “Towards the Development of a Real-Time Deepfake Audio Detection System in Communication Platforms,” arXiv Preprint, 2024.
[CrossRef] [Google Scholar] [Publisher Link]
[7] Staffy Kingra, Naveen Aggarwal, and Nirmal Kaur, “Emergence of Deepfakes and Video Tampering Detection Approaches: A Survey,” Multimedia Tools and Applications, vol. 82, no. 7, pp. 10165-10209, 2023.
[CrossRef] [Google Scholar] [Publisher Link]
[8] Yuxuan Wang et al., “Tacotron: Towards End-To-End Speech Synthesis,” arXiv Preprint, 2017.
[CrossRef] [Google Scholar] [Publisher Link]
[9] Sercan Arik et al., “Neural Voice Cloning with A Few Samples,” Advances in Neural Information Processing Systems, vol. 31, 2018.
[Google Scholar] [Publisher Link]
[10] Yipin Zhou, and Ser-Nam Lim, “Joint Audio-Visual Deepfake Detection,” Proceedings of the IEEE/CVF International Conference on Computer Vision, Montreal, QC, Canada, pp. 14800-14809, 2021.
[CrossRef] [Google Scholar] [Publisher Link]
[11] Abu Qais et al., “Deepfake Audio Detection with Neural Networks Using Audio Features,” International Conference on Intelligent Controller and Computing for Smart Power, Hyderabad, India, pp. 1-6, 2022.
[CrossRef] [Google Scholar] [Publisher Link]
[12] Andreas Rössler et al., “Faceforensics++: Learning to Detect Manipulated Facial Images,” Proceedings of the IEEE/CVF International Conference on Computer Vision, Seoul, South Korea, pp. 1-11, 2019.
[CrossRef] [Google Scholar] [Publisher Link]
[13] A. Saleema, and Sabu M. Thampi, Voice Biometrics: The Promising Future of Authentication in The Internet of Things, Handbook of Research on Cloud and Fog Computing Infrastructures for Data Science, pp. 360-389, 2018.
[CrossRef] [Google Scholar] [Publisher Link]
[14] Ali Javed et al., “Towards Protecting Cyber-Physical and IOT Systems from Single-and Multi-Order Voice Spoofing Attacks,” Applied Acoustics, vol. 183, 2021.
[CrossRef] [Google Scholar] [Publisher Link]
[15] Muteb Aljasem et al., “Secure Automatic Speaker Verification (SASV) System through sm-ALTP Features and Asymmetric Bagging,” IEEE Transactions on Information Forensics and Security, vol. 16, pp. 3524-3537, 2021.
[CrossRef] [Google Scholar] [Publisher Link]
[16] Mridul Sharma, and Mandeep Kaur, “A Review of Deepfake Technology: An Emerging AI Threat,” Soft Computing for Security Applications, Singapore, pp. 605-619, 2022.
[CrossRef] [Google Scholar] [Publisher Link]
[17] Yang Gao et al., “Generalized Spoofing Detection Inspired from Audio Generation Artifacts,” arXiv Preprint, 2021.
[CrossRef] [Google Scholar] [Publisher Link]
[18] Clara Borrelli et al., “Synthetic Speech Detection Through Short-Term and Long-Term Prediction Traces,” EURASIP Journal on Information Security, vol. 2021, no. 1, pp. 1-14, 2021.
[CrossRef] [Google Scholar] [Publisher Link]
[19] Massimiliano Todisco et al., “ASVspoof 2019: Future Horizons in Spoofed and Fake Audio Detection,” arXiv Preprint, 2019.
[CrossRef] [Google Scholar] [Publisher Link]
[20] Nishant Subramani, and Delip Rao, “Learning Efficient Representations for Fake Speech Detection,” Proceedings 34th AAAI Conference on Artificial Intelligence, vol. 34, no. 4, pp. 5859-5866, 2020.
[CrossRef] [Google Scholar] [Publisher Link]
[21] Dora M. Ballesteros et al., “Deep4SNet: Deep Learning for Fake Speech Classification,” Expert Systems with Applications, vol. 184, 2021.
[CrossRef] [Google Scholar] [Publisher Link]
[22] Emily R. Bartusiak, and Edward J. Delp, “Frequency Domain-Based Detection of Generated Audio,” arXiv Preprint, 2022.
[CrossRef] [Google Scholar] [Publisher Link]
[23] Mohammed Lataifeh et al., “Arabic Audio Clips: Identification and Discrimination of Authentic Cantillations from Imitations,” Neurocomputing, vol. 418, pp. 162-177, 2020.
[CrossRef] [Google Scholar] [Publisher Link]
[24] Mohammed Lataifeh, and Ashraf Elnagar, “Ar-DAD: Arabic Diversified Audio Dataset,” Data in Brief, vol. 33, 2020.
[CrossRef] [Google Scholar] [Publisher Link]
[25] Zhenchun Lei et al., “Siamese Convolutional Neural Network Using Gaussian Probability Feature for Spoofing Speech Detection,” Interspeech, pp. 1116-1120, 2020.
[CrossRef] [Google Scholar] [Publisher Link]
[26] Heinz Hofbauer, and Andreas Uhl, “Calculating A Boundary for The Significance from The Equal-Error Rate,” International Conference on Biometrics, Halmstad, Sweden, pp. 1-4, 2016.
[CrossRef] [Google Scholar] [Publisher Link]
[27] Steven Camacho, Dora Maria Ballesteros, and Diego Renza, “Fake Speech Recognition Using Deep Learning,” Applied Computer Sciences in Engineering: 8th Workshop on Engineering Applications, Medellín, Colombia, pp. 38-48, 2021.
[CrossRef] [Google Scholar] [Publisher Link]
[28] Ricardo Reimao, and Vassilios Tzerpos, “For: A Dataset for Synthetic Speech Detection,” International Conference on Speech Technology and Human-Computer Dialogue, Timisoara, Romania, pp. 1-10, 2019.
[CrossRef] [Google Scholar] [Publisher Link]
[29] Run Wang et al., “Deepsonar: Towards Effective and Robust Detection of AI-Synthesized Fake Voices,” Proceedings of the 28th ACM International Conference on Multimedia, Seattle WA, USA, pp. 1207-1216, 2020.
[CrossRef] [Google Scholar] [Publisher Link]
[30] R.L.M.A.P.C. Wijethunga et al., “Deepfake Audio Detection: A Deep Learning Based Solution for Group Conversations,” 2nd International Conference on Advancements in Computing, Malabe, Sri Lanka, vol. 1, pp. 192-197, 2020.
[CrossRef] [Google Scholar] [Publisher Link]
[31] Joao Monteiro, Jahangir Alam, and Tiago H. Falk, “Generalized End-To-End Detection of Spoofing Attacks to Automatic Speaker Recognizers,” Computer Speech & Language, vol. 63, 2020.
[CrossRef] [Google Scholar] [Publisher Link]
[32] Janavi Khochare et al., “A Deep Learning Framework for Audio Deepfake Detection,” Arabian Journal for Science and Engineering, vol. 47, no. 3, pp. 3447-3458, 2021.
[CrossRef] [Google Scholar] [Publisher Link]
[33] Hasam Khalid et al., “Evaluation of an Audio-Video Multimodal Deepfake Dataset Using Unimodal and Multimodal Detectors,” Proceedings of the 1st Workshop on Synthetic Multimedia-Audiovisual Deepfake Generation and Detection, Virtual Event China, pp. 7 15, 2021.
[CrossRef] [Google Scholar] [Publisher Link]
[34] Hasam Khalid et al., “FakeAVCeleb: A Novel Audio-Video Multimodal Deepfake Dataset,” arXiv Preprint, 2021.
[CrossRef] [Google Scholar] [Publisher Link]
[35] Moustafa Alzantot, Ziqi Wang, and Mani B. Srivastava “Deep Residual Neural Networks for Audio Spoofing Detection,” arXiv Preprint, 2019.
[CrossRef] [Google Scholar] [Publisher Link]
[36] Cheng-I Lai et al., “ASSERT: Anti-Spoofing with Squeeze-Excitation and Residual Networks,” arXiv Preprint, 2019.
[CrossRef] [Google Scholar] [Publisher Link]
[37] Ziyue Jiang et al., “Self-Supervised Spoofing Audio Detection Scheme,” Interspeech, pp. 4223-4227, 2020.
[CrossRef] [Google Scholar] [Publisher Link]
[38] Kai-Da Xu et al., “60-GHz Third-Order On-Chip Bandpass Filter Using GaAs pHEMT Technology,” Semiconductor Science and Technology, vol. 37, no. 5, 2022.
[CrossRef] [Google Scholar] [Publisher Link]
[39] In-Jae Yu et al., “Manipulation Classification for JPEG Images Using Multi-Domain Features,” IEEE Access, vol. 8, pp. 210837-210854, 2020.
[CrossRef] [Google Scholar] [Publisher Link]
[40] Trisha Mittal, “Emotions Don't Lie: An Audio-Visual Deepfake Detection Method Using Affective Cues,” Proceedings of the 28th ACM International Conference on Multimedia, Seattle WA, USA, pp. 2823-2832, 2020.
[CrossRef] [Google Scholar] [Publisher Link]
[41] Xu Li et al., “Replay and Synthetic Speech Detection with Res2net Architecture,” IEEE International Conference on Acoustics, Speech and Signal Processing, Toronto, ON, Canada, pp. 6354-6358, 2021.
[CrossRef] [Google Scholar] [Publisher Link]
[42] Jiangyan Yi et al., “Half-Truth: A Partially Fake Audio Detection Dataset,” arXiv Preprint, 2021.
[CrossRef] [Google Scholar] [Publisher Link]
[43] Rohan Kumar Das, Jichen Yang, and Haizhou Li, “Data Augmentation with Signal Companding for Detection of Logical Access Attacks,” IEEE International Conference on Acoustics, Speech and Signal Processing, Toronto, ON, Canada, pp. 6349-6353, 2021.
[CrossRef] [Google Scholar] [Publisher Link]
[44] Haoxin Ma et al., “Continual Learning for Fake Audio Detection,” arXiv Preprint, 2021.
[CrossRef] [Google Scholar] [Publisher Link]
[45] Ehab A. AlBadawy, Siwei Lyu, and Hany Farid, “Detecting AI-Synthesized Speech Using Bispectral Analysis,” CVPR Workshops, pp. 104-109, 2019.
[Google Scholar] [Publisher Link]
[46] Yang Gao et al., “Generalized Spoofing Detection Inspired from Audio Generation Artifacts,” arXiv Preprint, 2021.
[CrossRef] [Google Scholar] [Publisher Link]
[47] Joao Monteiro, Jahangir Alam, and Tiago H. Falk, “Generalized End-To-End Detection of Spoofing Attacks to Automatic Speaker Recognizers,” Computer Speech & Language, vol. 63, 2020.
[CrossRef] [Google Scholar] [Publisher Link]
[48] Tianxiang Chen et al., “Generalization of Audio Deepfake Detection,” Odyssey, The Speaker and Language Recognition Workshop, pp. 132-137, 2020.
[Google Scholar] [Publisher Link]
[49] Lian Huang, and Chi-Man Pun, “Audio Replay Spoof Attack Detection by Joint Segment-Based Linear Filter Bank Feature Extraction and Attention-Enhanced DenseNet-BiLSTM Network,” IEEE/ACM Transactions on Audio, Speech, and Language Processing, vol. 28, pp. 1813-1825, 2020.
[CrossRef] [Google Scholar] [Publisher Link]