Using Extended Kalman Filter to Observe State Parameters of the Twin Rotor MIMO System in Order to Install Model Predictive Control Algorithm Based Phisical Model
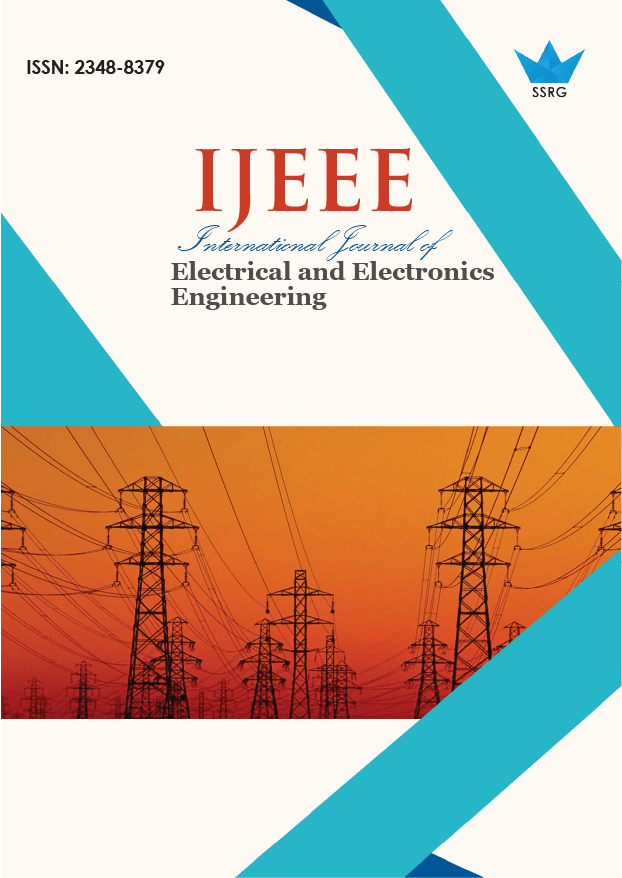
International Journal of Electrical and Electronics Engineering |
© 2017 by SSRG - IJEEE Journal |
Volume 4 Issue 12 |
Year of Publication : 2017 |
Authors : Huong T.M. Nguyen |
How to Cite?
Huong T.M. Nguyen, "Using Extended Kalman Filter to Observe State Parameters of the Twin Rotor MIMO System in Order to Install Model Predictive Control Algorithm Based Phisical Model," SSRG International Journal of Electrical and Electronics Engineering, vol. 4, no. 12, pp. 1-7, 2017. Crossref, https://doi.org/10.14445/23488379/IJEEE-V4I12P101
Abstract:
Most modern control methods including model predictive control (MPC) need to be measured or observed the state vectors of the object model. However, for real systems are often not fully measured state variables. Meanwhile, we must use the state observations to estimate the state vectors of the object model. One of the effective solutions to estimate the state of the system is to use Kalman filter for linear systems and extended Kalman filter (EKF) for nonlinear systems. This paper, we will mention the construction of extended Kalman filter to estimate the state parameters of the Twin rotor MIMO system (TRMS) and use this estimation to conduct tests on the real system so as to check the solution of the optimal problem by SQP algorithm mentioned in [4] and use variational method to solve the optimal problems, announced in [5] for the TRMS. Specifically the SQP algorithm and variational methods in the MPC given in [1], [2], [3].
Keywords:
Model predictive control, the Twin rotor MIMO system (TRMS), Kalman filter, state parameters, state observation.
References:
[1] Phuoc. Nguyen.D, (2016) “Optimization in control and Optimal control”. Publishing Technology, Ha Noi University of Sciences and Technology.
[2] Nocedal. J and Wright. S.J, (1996) Numerical Optimization. Springer - New York.
[3] Grüne. L and Pannek. J, (2010) “Nonlinear model predictive control,” Theory and Algorithms, Springer.
[4] Huong. Nguyen.T.M, Thai. Mai.T, Chinh. Nguyen. H, Dung. Tran.T and Lai. Lai.K, (09-2014), “Model Predictive Control for Twin Rotor MIMO system”, The University of Da Nang Journal of science and Technology, 12[85], pp. 39 - 42.
[5] Huong Nguyen T. M., Thai Mai T., Lai Lai K. (2015), “Model Predictive Control to get Desired Output with Infinite Predictive Horizon for Bilinear Continuous Systems”, International Journal of Mechanical Engineeringand Robotics Research, Vol. 4, No. 4, pp. 299 - 303.
[6] A. Rahideh, M.H. Shaheed. “Constrained output feedback model predictive control for nonlinear systems”, Control Engineering Practive 20, 2012, pp. 431-443.
[7] Huong. Nguyen.T.M, Thai. Mai.T, Chinh. Nguyen. H and Lai. Lai.K, “Researching effects of state parametters in Twin Rotor Mimo System (TRMS),” Journal of science and Technology of Thai Nguyen University, vol. 120, 2014, issue 06, pp. 87 – 92.
[8] A. Rahideh and M.H. Shaheed, “Mathematical dynamic modelling of a twin rotor multiple input–multiple output system,”, Proc. of the IMeche, Part I. Journal of Systems and Control Engineering 221, 2007, pp.89-101.