Development of an Improved Monte Carlo Simulation Algorithm for Reliability Assessment of Large Distribution Systems
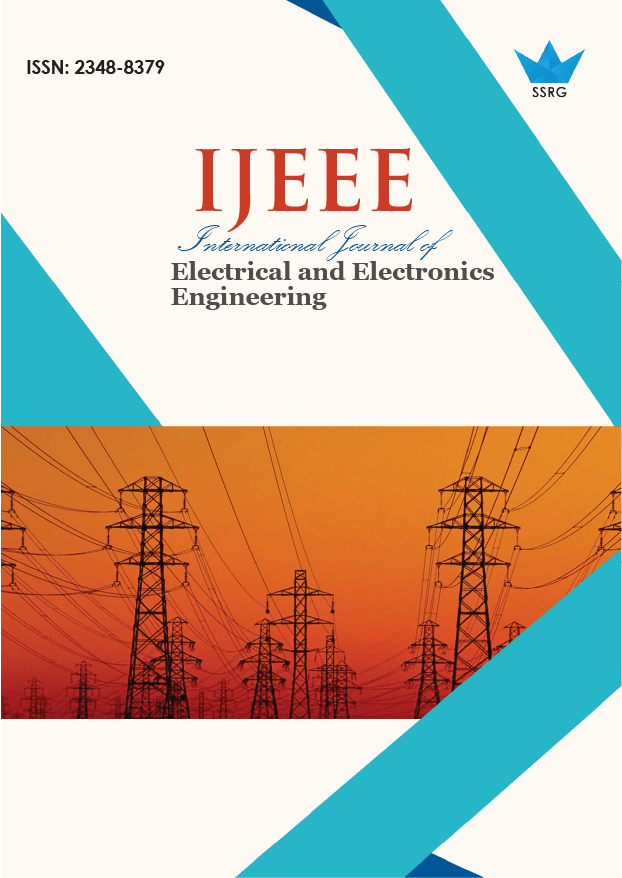
International Journal of Electrical and Electronics Engineering |
© 2020 by SSRG - IJEEE Journal |
Volume 7 Issue 5 |
Year of Publication : 2020 |
Authors : Ganiyu Adedayo Ajenikoko,Oluwadare Emmanuel Seluwa,Sarafadeen Adewale Jimoh, Moshood Kola Omotosho, Olubunmi Adewale Akinola, Ademola Abdulkareem,Sodiq Dayo Akinwumi, Olaide A, Osinubi |
How to Cite?
Ganiyu Adedayo Ajenikoko,Oluwadare Emmanuel Seluwa,Sarafadeen Adewale Jimoh, Moshood Kola Omotosho, Olubunmi Adewale Akinola, Ademola Abdulkareem,Sodiq Dayo Akinwumi, Olaide A, Osinubi, "Development of an Improved Monte Carlo Simulation Algorithm for Reliability Assessment of Large Distribution Systems," SSRG International Journal of Electrical and Electronics Engineering, vol. 7, no. 5, pp. 21-30, 2020. Crossref, https://doi.org/10.14445/23488379/IJEEE-V7I5P103
Abstract:
Reliability of power system refers to the ability of the system to supply electrical energy to its customers as at when due. Failure of power system to do this makes the system to be unreliable. The parametric indices employed in assessing the level of performance of the power system are termed “reliability indices”. Assessment of distribution system reliability can be done with two notable reliability indices- System Average Interruption Duration Index SAIDI and System Average Interruption Frequency Duration Index SAIFI. Previous studies have focused attentions on reliability assessment of large distribution systems using Monte-Carlo simulation method. This Method suffered some draw-backs such as computational efficiency and convergence problems. This research paper then developed an improved Monte-Carlo simulation algorithm for reliability assessment of large distribution systems. In the improved Monte Carlo Simulation algorithm, all the feeders were simulated separately for a period of 100 years. At the end of each iteration, the contribution of outages on various feeders was cumulated to compute the annual system indices and the confidence level of the output data was then computed to determine if the simulation had attained convergence at a specified precision level.
The time of failure and repair duration of all the segments of the feeders were generated and the faults were processed in a sequential order to evaluate the contributions of each outage to the annual system indices-SAIDI and SAIFI. The faults and repair times of the segments were saved after loading the next feeder once the time has reached the iteration period of 100years. Confidence test was performed on the annual system reliability indices-SAIDI and SAIFI after all the feeders have been simulated. Subsystems of different sizes but similar reliability characteristics i.e average SAIDI and SAIFI values were created from the 100 feeder system.
The results of the research paper showed that the computational times increased as the number of feeders increased, thus obeying a linear relationship because the state-duration employed in the algorithm generated a list of failure times for individual feeder segments so that the time of all outages in a given year were known. The same number of faults were generated for obtaining a specified accuracy level for systems of different sizes which indicated that the number of faults that must be simulated for a given accuracy level was independent of the system size.
The mean SAIDI for 100, 90, 80, 70, 60 and 50 feeders were 3.39, 3.36, 3.34, 347, 3.53 and 3.55 respectively while feeders of system sizes 40, 30, 20 and 10 gave mean SAID of 3.68, 3.92, 3.26 and 3.77 respectively because the concept of relative standard deviation can be used to compare the deviation of the reliability indices of different systems.. There was an inverse relationship between the system sizes and the standard deviation. Thus, for system sizes 100, 90, 80, 70, 60, 50, 40, 30, 20 and 10, the standard deviations were 1.287, 1.312, 1.426, 1.510, 1.687, 1.818, 2.113, 2.415, 2.437 and 3.553 respectively. The mean SAIFI did not obey any definite pattern as they fluctuated as the system sizes changed. Thus, for system sizes 100, 80, 60, 40 and 20, the mean SAIFI were recorded as 0.947, 0.912, 0.902, 0.894 and 0.921 respectively due to the configuration of the system feeders. As the system sizes decreased, the SAIFI standard deviation increased proportionately due to the number of customers experiencing prolonged interruptions during the time. The spread of the annual system indices depends on the size of the system, thus the smaller the system size, the greater is the deviation in annual reliability indices. In this case, smaller systems had greater variations in the annual indices as compared to larger systems, even though, the average reliability level was the same in both cases.If the same reliability standard was used for different systems, smaller systems were likely to experience more interruptions more frequently as compared to larger ones. The reliability standards must ensure that smaller systems were not affected inadvertently.
The results of the research paper indicated that the performance improvement of the improved Monte Carlo simulations was due to the state-duration method used in the algorithm which generated a list of failure times for individual feeder segment. In addition, as the system size increased, the number of years for which the simulation must be performed to obtain the indices with 85% confidence level decreased and the number of faults analyzed for attainment of convergence which must be simulated for a given accuracy level was independent of the system size
Keywords:
Improved Monte Carlo Simulation, Algorithm, Reliability Indices, SAIDI, SAIFI, Confidence test, Feeders.
References:
[1] R. Billinton, and T. Jonnavithula, “Optimal switching Device placement in radial distribution systems”, IEEE Transactions on power systems, vol. 11(3), pp 1646-1651, 2016.
[2] E. Brown, S. Gupta, R. D. Christie, S. S. Venkara, and R. Fletcher, “Distribution system reliability assessment: momentary interruptions and storms”, IEEE Transactions on power Delivery, vol. 12(4), pp. 1569-1575, 2017.
[3] L. Cummins, E. P. Krider, and M. D. Malone, “The U.S national lightning detection network and applications of cloud- to-ground lightning data by electric power utilities”, IEEE Transactions on Electromagnetic Compatibility, vol.40(4), pp. 465-480, 2018.
[4] A. Huda, and R. Zivanovic, “Accelerated distribution systems reliability evaluation by Multilevel Monte Carlo Simulation: Implementation of Two Discretisation Schemes”, LET Generation , Transmission and Distribution, 11(13), pp.3397-3405, 2017.
[5] L. Goel, and R. Billinton, “Monte- carlo simulation applied to distribution feeder reliability evaluation”, Electric Power System Research, vol. 29(3), pp. 193-202, 2014.
[6] P. Wang, R. Billinton, and L. Goel, “Unreliability cost assessment of an electric power system using reliability network equivalent approach”, IEEE Power Engineering Review, vol. 22(7), pp. 57-58, 2007.
[7] K. Xie, J. Zhou, and R. Billinton, “Reliability evaluation algorithm for complex medium voltage electrical distribution networks based on the shortest path”, IEEE Proceedings Generation, Transmission and Distribution, vol. 150(6), pp. 686-690, 2004.
[8] S. Asgarpoor, and M. J. Mathine, “Reliability evaluation of distribution systems with non-exponential down times”, IEEE Transactions on power systems, vol.12(2), pp 579-584, 2017.
[9] R. Billinton, and J. E. Billinton, “Distribution system reliability indices”, IEEE Transactions on power Delivery, vol. 4(1) pp. 561-568, 2009.
[10] R. Billinton, and R. Goel, “An analytical approach to evaluate probability distributions associated with the reliability indices of electrical distribution systems”, IEEE Trans., vol. 104(11), pp 245-251, 2016.
[11] L. Goel, “Monte carlo simulation-based reliability studies of a distribution test system”, Electric Power Systems Research, vol. 54(1), pp. 55-65, 2000.
[12] W. Li, Z. Li, and Y. Liu, “Reliability assessment of complex radial distribution system”, Proceedings of the CSEE, vol. 23(3), pp. 69-73, 2003.
[13] A. Ozdemir, R. Caglar, and F. Mekie, “A new active failure simulation approach for distribution system reliability assessment”, International Conference on Power System Technology, Proceedings, Powercon. IEEE, vol. 1, pp. 237-240, 1998.
[14] G. Wan, Z. Ren, and R. Wu, (2004):” Application of hybrid method in reliability assessment of complex distribution network”, Proceedings of the CSEE, vol. 24(9), pp. 92-98, 2004.
[15] Z. Wei, F. Zhou, and C. Xiao, “Reliability evaluation algorithm for complex medium voltage distribution network analysis based on simplified network model”, Power system Technology, vol. 30(15)., pp. 72-75, 2006.
[16] Z. Xu, and J. Zhou, (2005):” Improved fault traversal method for reliability assessment of complex distribution networks”, Power System Technology, vol. 299(14), pp. 64-67, 2005.
[17] Z. Bie, and X. Wang, “Reliability analysis of power distribution system “, Electric power , pp. 10-13, 1997.
[18] R. Billinton, and P. Wang, "Teaching distribution system reliability evaluation using monte carlo simulation”, IEEE Transactions on power systems, vol. 14(2), pp. 397-403, 2009.
[19] R. Billinton, and T. Wojczynski, “Distributional variation of distribution system reliability indices”, IEEE Transactions on power Apparatus and Systems, vol. 104(11), pp. 3152-3160, 2015.
[20] Z. Li, and W. Li, “Fault transversal algorithm for system reliability assessment based on radial distribution”, Journal of Automation of Electric power systems, 26(2):53-56, 2002.