Fault Prediction of Induction Motor using Machine Learning Algorithm
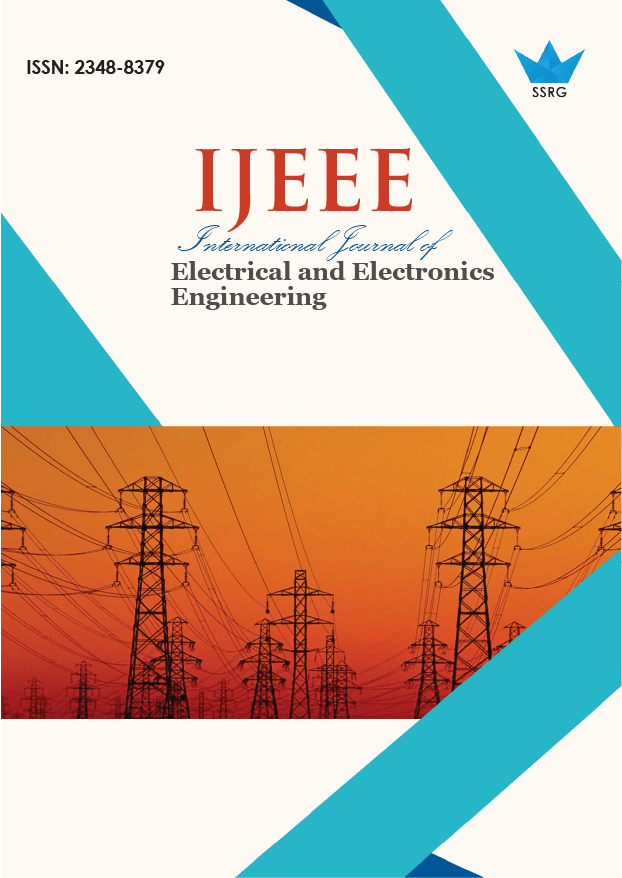
International Journal of Electrical and Electronics Engineering |
© 2021 by SSRG - IJEEE Journal |
Volume 8 Issue 11 |
Year of Publication : 2021 |
Authors : B. Balaji, Kanagaraj. U, Mahendran. R, Rethinasiranjeevi. R |
How to Cite?
B. Balaji, Kanagaraj. U, Mahendran. R, Rethinasiranjeevi. R, "Fault Prediction of Induction Motor using Machine Learning Algorithm," SSRG International Journal of Electrical and Electronics Engineering, vol. 8, no. 11, pp. 1-6, 2021. Crossref, https://doi.org/10.14445/23488379/IJEEE-V8I11P101
Abstract:
Induction motor fault identification prior to the occurrence of total shut-down is critical for industries. The identification of faults based on condition monitoring techniques and the use of machine learning has enormous potential. Machine learning's power may be harnessed and properly applied for motor defect detection. To avoid losses, the issue, particularly in induction motors, must be repaired at the appropriate time. Machine learning algorithm applications in the sphere of defect detection give a dependable and effective preventative maintenance solution. In this paper, an algorithm-based machine learning approach is developed to learn features from the frequency distribution of vibration signals with the goal of characterizing the working status of induction motors such as current, voltage, and temperature, and it is also updated in the IoT-based application. It combines feature extraction and classification tasks to enable automated and intelligent problem diagnosis.
Keywords:
Induction Motor, Machine, Fault Prediction
References:
[1] H Gao, L Liang, X Chen, et al. Feature extraction and recognition for rolling element bearing fault utilizing short-time Fourier transform and non-negative matrix factorization. Chinese Journal of Mechanical Engineering, 28(1) (2015) 96–105.
[2] G Chen, L Qie, A Zhang, et al. Improved CICA algorithm used for single-channel compound fault diagnosis of rolling bearings. Chinese Journal of Mechanical Engineering, 29(1) (2016) 204–211.
[3] M Riera-Guasp, J A Antonino-Daviu, G A Capolino. Advances in the electrical machine, power electronic, and drive condition monitoring and fault detection: state of the art. IEEE Transactions on Industrial Electronics., 62(3) (2015) 1746–1759.
[4] M H Drif, A J Cardoso. Stator fault diagnostics in squirrel cage three-phase induction motor drives using the instantaneous active and reactive power signature analyses. IEEE Transactions on Industrial Informatics, 10(2) (2014) 1348–1360.
[5] Y Wang, F Zhang, T Cui, et al. Fault diagnosis for manifold absolute pressure sensor (MAP) of diesel engine based on Elman neural network observer. Chinese Journal of Mechanical Engineering, 29(2) (2016) 386–395.
[6] J Antonino-Daviu, S Aviyente, E G Strangas, et al. Scale-invariant feature extraction algorithm for the automatic diagnosis of rotor asymmetries in induction motors. IEEE Transactions on Industrial Informatics, 9(1) (2013) 100–108.
[7] J Faiz, V Ghorbanian, BM Ebrahimi. EMD-based analysis of industrial induction motors with broken rotor bars for identification of operating points at different supply modes. IEEE Transactions on Industrial Informatics, 10(2) (2014) 957–966.
[8] P Karvelis, G Georgoulas, I P Tsoumas, et al. A symbolic representation approach for the diagnosis of broken rotor bars in induction motors. IEEE Transactions on Industrial Informatics, 11(5) (2015) 1028–1037.
[9] M Zhang, J Tang, X Zhang, et al. Intelligent diagnosis of the short hydraulic signal based on improved EEMD and SVM with few low-dimensional training samples. Chinese Journal of Mechanical Engineering, 29(2) (2016) 396–405.
[10] D Matic´, F Kulic´, M Pineda-sa´nchez, et al. Support vector machine classifier for diagnosis in electrical machines: Application to the broken bar. Expert Systems with Applications, 39(10) (2012) 8681–8689.
[11] Y Lei, F Jia, J Lin, et al. An intelligent fault diagnosis method using unsupervised feature learning towards big mechanical data. IEEE Transactions on Industrial Electronics, 63(5) (2016) 3137–3147.
[12] T Boukra, A Lebaroud, G Clerc. Statistical and neural-network approaches for the classification of induction machine faults using the ambiguity plane representation. IEEE Transactions on Industrial Electronics, 60(9) (2013) 4034–4042.
[13] H Keskes, A Braham. Recursive undecimated wavelet packet transform and DAG SVM for induction motor diagnosis. IEEE Transactions on Industrial Informatics, 11(5) (2015) 1059–1066.
[14] C Chen, B Zhang, G Vachtsevanos. Prediction of machine health condition using neuro-fuzzy and Bayesian algorithms. IEEE Transactions on Instrumentation and Measurement, 61(2) (2012) 297–306.
[15] Y L Murphey, M A Masru, Z Chen, et al. Model-based fault diagnosis in electric drives using machine learning. IEEE/ASME Transactions on Mechatronics, 11(3) (2006) 290–303.
[16] J Wang, R X Gao, R Yan. Multi-scale enveloping order spectrogram for rotating machine health diagnosis. Mechanical Systems and Signal Processing, 46(1) (2014) 28–44.