SEOA DRN: Social Exponential Optimization Algorithm Based Deep Residual Network for Visual Speech Recognition
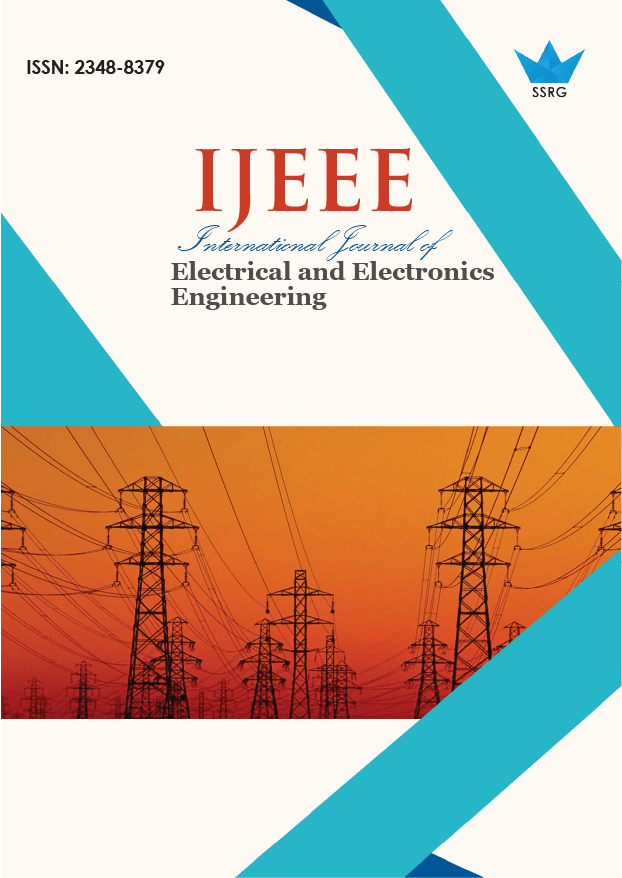
International Journal of Electrical and Electronics Engineering |
© 2023 by SSRG - IJEEE Journal |
Volume 10 Issue 1 |
Year of Publication : 2023 |
Authors : G. N. Srikanth, M. K. Venkatesha |
How to Cite?
G. N. Srikanth, M. K. Venkatesha, "SEOA DRN: Social Exponential Optimization Algorithm Based Deep Residual Network for Visual Speech Recognition," SSRG International Journal of Electrical and Electronics Engineering, vol. 10, no. 1, pp. 90-105, 2023. Crossref, https://doi.org/10.14445/23488379/IJEEE-V10I1P109
Abstract:
The recognition of visual speech is considered an emerging solution for feasible recognition. However, the choice of imperative features is a challenging task for acquiring elevated performance. A deep model is devised for lip reading-based visual speech recognition. The CFPNet-M is being employed for extracting the regions of lips. The Lipreading technique helps to provide a silent interface and enhances speech recognition in noisy platforms, as the optical signal is not impacted via noise. The features, like Convolutional Neural Network (CNN) features, Gabor features, width, area, mass, location, orientation, Local Gabor Ternary Pattern (LGTP), statistical features, along with the voice features and spectral features, are considered. With the aid of a deep residual network (DRN), speech recognition is carried out effectively, wherein weight update of DRN is performed using Social Exponential Optimization Algorithm (SEOA). The resultant output of SEOA-based DRN is considered for visual speech recognition. The experimentation of the proposed method is done using certain measures by illustrating the efficiency of each technique. The proposed SEOA-DRN offered high efficiency with elevated accuracy of 88.4%, sensitivity of 90.6% and specificity of 90.6%.
Keywords:
Visual speech recognition, Deep Residual Network, Voice signals, Video frames, Lip reading
References:
[1] Xuejie Zhang et al., “Visual Speech Recognition with Lightweight Psychologically Motivated Gabor Features,” Entropy, vol. 22, no. 12, p. 1367, 2020. Crossref, https://doi.org/10.3390/e22121367
[2] Stavros Petridis et al., “End-to-End Visual Speech Recognition for Small-Scale Datasets,” Pattern Recognition Letters, vol. 131, pp. 421-427, 2020. Crossref, https://doi.org/10.1016/j.patrec.2020.01.022
[3] H Liu, Z Chen, and B Yang, “Lip Graph Assisted Audio-Visual Speech Recognition Using Bidirectional Synchronous Fusion,” Proceedings of INTERSPEECH, pp. 3520-3524, 2020. Crossref, https://doi.org/10.21437/Interspeech.2020-3146
[4] Pingchuan Ma, Stavros Petridis, and Maja Pantic, “End-To-End Audio-Visual Speech Recognition with Conformers,” Proceedings of ICASSP 2021-2021 IEEE International Conference on Acoustics, Speech and Signal Processing, pp. 7613-7617, 2021. Crossref, https://doi.org/10.1109/ICASSP39728.2021.9414567
[5] Nilay Shrivastava et al., “MobiVSR: Efficient and Light-Weight Neural Network for Visual Speech Recognition on Mobile Devices,” Proceedings of INTERSPEECH, pp. 2753-2757, 2019. Crossref, https://doi.org/10.21437/Interspeech.2019-3273
[6] Abitha A, and Lincy K, "A Faster RCNN Based Image Text Detection and Text to Speech Conversion," SSRG International Journal of Electronics and Communication Engineering, vol. 5, no. 5, pp. 11-14, 2018. Crossref, https://doi.org/10.14445/23488549/IJECE-V5I5P103
[7] T. Ozcan, and A. Basturk, “Lip Reading using Convolutional Neural Networks with and without Pre-Trained Models,” Balkan Journal of Electrical and Computer Engineering, vol. 7, no. 2, pp. 195-201, 2019. Crossref, https://doi.org/10.17694/bajece.479891
[8] Yuanyao Lu, and Jie Yan, “Automatic Lip Reading Using Convolution Neural Network and Bidirectional Long Short-Term Memory,” International Journal of Pattern Recognition and Artificial Intelligence, vol. 34, no. 101, p. 2054003, 2020. Crossref, https://doi.org/10.1142/S0218001420540038
[9] Michael S. Saccucci, Raid W. Amin, and James M. Lucas, “Exponentially Weighted Moving Average Control Schemes with Variable Sampling Intervals,” Communications in Statistics-Simulation and Computation, vol. 21, no.3, pp. 627-657, 1992. Crossref, https://doi.org/10.1080/03610919208813040
[10] Ayush Jain et al., "Detection of Sarcasm through Tone Analysis on video and Audio files: A Comparative Study on AI Models Performance," SSRG International Journal of Computer Science and Engineering, vol. 8, no. 12, pp. 1-5, 2021. Crossref, https://doi.org/10.14445/23488387/IJCSE-V8I12P101
[11] Adriana Fernandez-Lopez, and Federico M.Sukno, “Survey on Automatic Lip-Reading in the Era of Deep Learning,” Image and Vision Computing, vol. 78, pp. 53-72, 2018. Crossref, https://doi.org/10.1016/j.imavis.2018.07.002
[12] George Sterpu, and Naomi Harte, “Towards Lipreading Sentences with Active Appearance Models,” 2018.
[13] Nancy Tye-Murray et al., “Lipreading in School-Age Children: The Roles of Age, Hearing Status, and Cognitive Ability,” Journal of Speech, Language, and Hearing Research, vol. 57, no. 2, pp. 556-565, 2014. Crossref, https://doi.org/10.1044/2013_JSLHR-H-12-0273
[14] C. Senthil Kumar, and Y. Raj Kumar, "Bus Embarking System for Visual Impaired People using Radio-Frequency Identification," SSRG International Journal of Electronics and Communication Engineering, vol. 4, no. 4, pp. 10-15, 2017. Crossref, https://doi.org/10.14445/23488549/IJECE-V4I4P103
[15] Yiting Li et al., “Lip Reading Using a Dynamic Feature of Lip Images and Convolutional Neural Networks,” Proceedings of 2016 IEEE/ACIS 15th International Conference on Computer and Information Science (ICIS), pp. 1-6, 2016. Crossref, https://doi.org/10.1109/ICIS.2016.7550888
[16] Timothy Israel Santos, and Andrew Abel, “Using Feature Visualisation for Explaining Deep Learning Models in Visual Speech,” Proceedings of 2019 IEEE 4th International Conference on Big Data Analytics, pp. 231-235, 2019. Crossref, https://doi.org/10.1109/ICBDA.2019.8713256
[17] Andrew Abel et al., “A Data Driven Approach to Audiovisual Speech Mapping,” Proceedings of International Conference on Brain Inspired Cognitive Systems, pp. 331-342, 2016. Crossref, https://doi.org/10.1007/978-3-319-49685-6_30
[18] The Oxford-BBC Lip Reading in the Wild (LRW). [Online]. Available: https://www.robots.ox.ac.uk/~vgg/data/lip_reading/lrw1.html
[19] Ravi Kumar Saini, and Mrs. Mamta Yadav, "Image & Video Quality Assessment and Human Visual Perception," International Journal of Computer & organization Trends, vol. 6, no. 3, pp. 1-4, 2016. Crossref, https://doi.org/10.14445/22492593/IJCOT-V34P301
[20] Ange Lou, Shuyue Guan, and Murray Loew, “CFPNet-M: A Light-Weight Encoder-Decoder Based Network for Multimodal Biomedical Image Real-Time Segmentation,” 2021.
[21] Wai Chee Yau, Hans Weghorn, and Dinesh Kant Kumar, “Visual Speech Recognition and Utterance Segmentation Based on Mouth Movement,” Proceedings of 9th Biennial Conference of the Australian Pattern Recognition Society on Digital Image Computing Techniques and Applications, pp. 7-14, 2007. Crossref, https://doi.org/10.1109/DICTA.2007.4426769
[22] Chandar Kumar et al., “Analysis of MFCC and BFCC in a Speaker Identification System,” Proceedings 2018 International Conference on Computing, Mathematics and Engineering Technologies, pp. 1-5, 2018. Crossref, https://doi.org/10.1109/ICOMET.2018.8346330
[23] Osama S. Faragallah, “Robust Noise MKMFCC–SVM Automatic Speaker Identification,” International Journal of Speech Technology, vol. 21, no. 2, pp. 185-192, 2018. Crossref, https://doi.org/10.1007/s10772-018-9494-9
[24] Kasiprasad Mannepalli, Panyam Narahari Sastry, and Maloji Suman, "A Novel Adaptive Fractional Deep Belief Networks for Speaker Emotion Recognition," Alexandria Engineering Journal, vol. 56, no. 4, pp. 485-497, 2017. Crossref, https://doi.org/10.1016/j.aej.2016.09.002
[25] Ahnaf Rashik Hassan, and Mohammad Aynal Haque, "Computer-Aided Sleep Apnea Diagnosis from Single-Lead Electrocardiogram Using Dual Tree Complex Wavelet Transform and Spectral Features," Proceedings of International Conference on Electrical & Electronic Engineering, pp. 49-52, 2015. Crossref, https://doi.org/10.1109/CEEE.2015.7428289
[26] Lichuan Liu et al., “Infant Cry Language Analysis and Recognition: An Experimental Approach,” IEEE/CAA Journal of Automatica Sinica, vol. 6, no. 3, pp. 778-788, 2019. Crossref, https://doi.org/10.1109/JAS.2019.1911435
[27] Arul Valiyavalappil Haridas et al., "Emotion Recognition of Speech Signal Using Taylor Series and Deep Belief Network Based Classification," Evolutionary Intelligence, pp. 1145-1158, 2022. Crossref, https://doi.org/10.1007/s12065-019-00333-3
[28] Siti Nurulain Mohd Rum, and Braxton Isaiah Boilis, "Sign Language Communication through Augmented Reality and Speech Recognition (LEARNSIGN)," International Journal of Engineering Trends and Technology, vol. 69, no. 4, pp. 125-130, 2021. Crossref, https://doi.org/10.14445/22315381/IJETT-V69I4P218
[29] Pei Wang, Hui Fu, and Ke Zhang, "A Pixel-Level Entropy-Weighted Image Fusion Algorithm Based on Bidimensional Ensemble Empirical Mode Decomposition," International Journal of Distributed Sensor Networks, vol. 14, no. 12, 2018. Crossref, https://doi.org/10.1177/1550147718818755
[30] J.S. Anita, and J.S. Abinaya, "Impact of Supervised Classifier on Speech Emotion Recognition," Multimedia Research, vol. 2, no. 1, pp. 9-16, 2019. Crossref, https://doi.org/10.46253/j.mr.v2i1.a2
[31] Zeng Runhua, and Zhang Shuqun, "Improving Speech Emotion Recognition Method of Convolutional Neural Network" International Journal of Recent Engineering Science, vol. 5, no. 3, pp. 1-7, 2018. Crossref, https://doi.org/10.14445/23497157/IJRES-V5I3P101
[32] R. Tamilaruvi et al., "Brain Tumor Detection in MRI Images using Convolutional Neural Network Technique," SSRG International Journal of Electrical and Electronics Engineering, vol. 9, no. 12, pp. 198-208, 2022. Crossref, https://doi.org/10.14445/23488379/IJEEE-V9I12P118
[33] T. Madhubala, R. Umagandhi, and P. Sathiamurthi, "Diabetes Prediction using Improved Artificial Neural Network using Multilayer Perceptron," SSRG International Journal of Electrical and Electronics Engineering, vol. 9, no. 12, pp. 167-179, 2022. Crossref, https://doi.org/10.14445/23488379/IJEEE-V9I12P115
[34] S. Vijitha, and S. N. Sreelaja, "Modified Leading Diagonal Sorting with Probabilistic Visual Cryptography for Secure Medical Image Transmission," Journal of Computational Science and Intelligent Technologies, vol. 3, no. 3, pp. 1-13, 2022. Crossref, https://doi.org/10.53409/MNAA/JCSIT/e202203030113
[35] G. N. Srikanth, and M. K. Venkatesha, "Performance Analysis of AI Models for Audio Digit Utterance Detection," Journal of Computational Science and Intelligent Technologies, vol. 3, no. 3, pp. 14-29, 2022. Crossref, https://doi.org/10.53409/MNAA/JCSIT/e202203031429
[36] Wentao Yu, Steffen Zeiler, and Dorothea Kolossa, “Multimodal Integration for Large-Vocabulary Audio-Visual Speech Recognition,” Proceedings 2020 28th European Signal Processing Conference, pp. 341-345, 2021. Crossref, https://doi.org/10.23919/Eusipco47968.2020.9287841
[37] M. Lievin, and F. Luthon, “Lip Features Automatic Extraction,” Proceedings 1998 International Conference on Image Processing, ICIP98 (Cat. No. 98CB36269), pp. 168-172, 1998. Crossref, https://doi.org/10.1109/ICIP.1998.727160
[38] Naser Karimi, and Khosro Khandani, “Social Optimization Algorithm with Application to Economic Dispatch Problem,” International Transactions on Electrical Energy Systems, vol. 30, no. 11, p. e12593, 2020. Crossref, https://doi.org/10.1002/2050- 7038.12593
[39] Zhicong Chen et al., “Deep Residual Network Based Fault Detection and Diagnosis of Photovoltaic Arrays Using Current-Voltage Curves and Ambient Conditions,” Energy Conversion and Management, vol. 198, p. 111793, 2019. Crossref, https://doi.org/10.1016/j.enconman.2019.111793