Brain Storm Optimization with Deep Learning-Based Intrusion Detection System in Vehicular Adhoc Networks
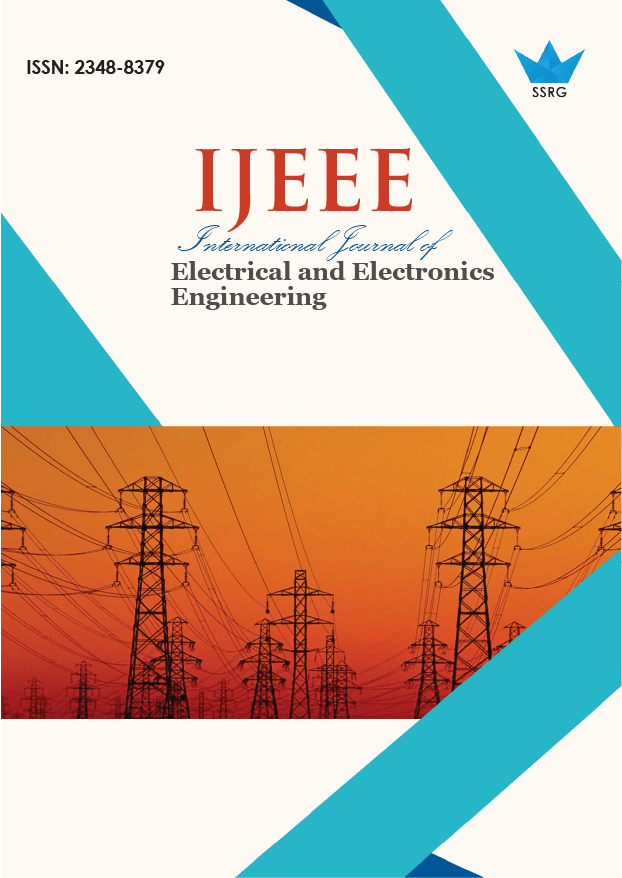
International Journal of Electrical and Electronics Engineering |
© 2023 by SSRG - IJEEE Journal |
Volume 10 Issue 1 |
Year of Publication : 2023 |
Authors : R. Mohan, G. Prabakaran, T. Priyaradhikadevi |
How to Cite?
R. Mohan, G. Prabakaran, T. Priyaradhikadevi, "Brain Storm Optimization with Deep Learning-Based Intrusion Detection System in Vehicular Adhoc Networks," SSRG International Journal of Electrical and Electronics Engineering, vol. 10, no. 1, pp. 176-186, 2023. Crossref, https://doi.org/10.14445/23488379/IJEEE-V10I1P117
Abstract:
Vehicular adhoc network (VANET) is an empowering technology in recent transportation systems for offering valuable information and safety, but prone to several attacks, such as active interference and passive eavesdropping. Intrusion detection systems (IDSs) are significant devices that alleviate threats by detecting malicious actions. In addition, the collaborations between vehicles in VANETs could enhance the accuracy level of detection by interacting with their experiences among nodes. So, distributed ML becomes a highly suitable structure for designing scalable and applicable collaborative detection techniques over VANETs. Therefore, this paper proposes a brain storm optimization with a deep learning-based intrusion detection system (BSODL-IDS) for VANET. In the presented BSODL-IDS technique, a primary stage of BSO based feature selection process is involved in it. For intrusion detection, the BSODL-IDS model exploits the long short-term memory recurrent neural network (LSTM-RNN) model. The Adamax optimizer is utilized at the last stage for the hyperparameter tuning of the LSTM-RNN technique. The experimental validation on the benchmark dataset illustrates the BSODL-IDS method's supremacy over other DL approaches.
Keywords:
Intrusion detection, Security, VANET, Deep learning, Feature selection, Parameter tuning.
References:
[1] Ahmed A. Aboelfottoh, and Marianne A. Azer, “Intrusion Detection in VANETs and ACVs using Deep Learning,” 2nd International Mobile, Intelligent, and Ubiquitous Computing Conference (MIUCC), pp. 241-245, 2022. Crossref, https://doi.org/10.1109/miucc55081.2022.9781691
[2] Hind Bangui, Mouzhi Ge, and Barbora Buhnova, “A Hybrid Machine Learning Model for Intrusion Detection in VANET,” Computing, vol. 104, no. 3, pp.503-531, 2022. Crossref, https://doi.org/10.1007/S00607-021-01001-0
[3] Fábio Gonçalves et al., “A Systematic Review on Intelligent Intrusion Detection Systems for Vanets,” 2019 11th International Congress on Ultra Modern Telecommunications and Control Systems and Workshops (ICUMT), pp. 1-10, 2019. Crossref, https://doi.org/10.1109/icumt48472.2019.8970942
[4] Jiangang Shu et al., “Collaborative Intrusion Detection for Vanets: A Deep Learning-Based Distributed SDN Approach,” IEEE Transactions on Intelligent Transportation Systems, vol. 22, no. 7, pp. 4519-4530, 2021. Crossref, https://doi.org/10.1109/TITS.2020.3027390
[5] Viacheslav Belenko, Vasiliy Krundyshev, and Maxim Kalinin, “Synthetic Datasets Generation for Intrusion Detection in VANET,” in Proceedings of the 11th International Conference on Security of Information and Networks, pp. 1-6, 2018. Crossref, https://doi.org/10.1145/3264437.3264479
[6] Ila Naqvi, and Alka Chaudhary, “Intrusion Detection Using Soft Computing Techniques in Vanets,” 2021 9th International Conference on Reliability, Infocom Technologies and Optimization (Trends and Future Directions) (ICRITO), pp. 1-4, 2021. Crossref, https://doi.org/10.1109/icrito51393.2021.9596468
[7] J. A. Smitha et al., "Optimized Routing on Wireless Body Sensor Network Using Adaptive Lion Optimization Algorithm for IoT," SSRG International Journal of Electrical and Electronics Engineering, vol. 9, no. 12, pp. 189-197, 2022. Crossref, https://doi.org/10.14445/23488379/IJEEE-V9I12P117
[8] Farithkhan Abbas Ali, and E. D. Kanmani Ruby, "Clustering Metric Algorithm for Cost-Effective Routing in Flying Ad-Hoc Networks," SSRG International Journal of Electrical and Electronics Engineering, vol. 9, no. 12, pp. 101-108, 2022. Crossref, https://doi.org/10.14445/23488379/IJEEE-V9I12P108
[9] Karpaga Priya R et al., "A Novel Spider Swarm Optimized Energy and Security Aware Clustering Protocol for Smart Grid Wireless Sensor Network," SSRG International Journal of Electrical and Electronics Engineering, vol. 9, no. 10, pp. 19-26, 2022. Crossref, https://doi.org/10.14445/23488379/IJEEE-V9I10P104
[10] S. Gavaskar, E. Ramaraj, and R. Surendiran, “A Compressed Anti IP Spoofing Mechanism Using Cryptography,” IJCSNS International Journal of Computer Science and Network Security, vol. 12, no. 11, pp.137-140, 2012.
[11] BasantSubba, Santosh Biswas, and SushantaKarmakar, “A Game Theory Based Multi Layered Intrusion Detection Framework for VANET,” Future Generation Computer Systems, vol. 82, pp. 12-28, 2018. Crossref, https://doi.org/10.1016/j.future.2017.12.008
[12] S. Revathi, and A. Malathi, “Network Intrusion Detection Using Hybrid Simplified Swarm Optimization Technique,” International Journal of P2P Network Trends and Technology (IJPTT), vol. 3, no. 5, pp. 6-10, 2013.
[13] Tarak Nandy et al., “T-BCIDS: Trust-Based Collaborative Intrusion Detection System for VANET,” 2020 National Conference on Emerging Trends on Sustainable Technology and Engineering Applications (NCETSTEA), IEEE, 2020. Crossref, https://doi.org/10.1109/NCETSTEA48365.2020.9119934
[14] Junwei Liang et al., “A Novel Intrusion Detection System for Vehicular Ad Hoc Networks (Vanets) Based on Differences of Traffic Flow and Position,” Applied Soft Computing, vol. 75, pp. 712-727.
[15] Man Zhou et al., “Distributed Collaborative Intrusion Detection System for Vehicular Ad Hoc Networks Based on Invariant,” Computer Networks, vol. 172, 2020. Crossref, https://doi.org/10.1016/j.comnet.2020.107174
[16] S.Navya Sai, and K. Kishoreraju, "Improved Privacy Preserving Decision Tree Approach for Network Intrusion Detection,” International Journal of Computer & Organization Trends, vol. 6, no. 1, pp. 55-60, 2016.
[17] Mohammad Dawood Momand, Dr Vikas Thada, and Mr. Utpal Shrivastava, "Intrusion Detection System in IoT Network," SSRG International Journal of Computer Science and Engineering , vol. 7, no. 4, pp. 11-15, 2020. Crossref, https://doi.org/10.14445/23488387/IJCSE-V7I4P104
[18] Yuhui Shi, “Brain Storm Optimization Algorithm,” International Conference in Swarm Intelligence, vol. 67285, 2011. Crossref, https://doi.org/10.1007/978-3-642-21515-5_36
[19] C. Narmatha et al., “A Hybrid Fuzzy Brain-Storm Optimization Algorithm for the Classification of Brain Tumor MRI Images,” Journal of Ambient Intelligence and Humanized Computing, pp. 1-9, 2020. Crossref, https://doi.org/10.1007/s12652-020-02470-5
[20] Gunja Ambica , and Mrs.N.Rajeswari, "Robust Data Clustering Algorithms for Network Intrusion Detection,” International Journal of Computer & Organization Trends, vol. 2, no. 5, pp. 6-11, 2012.
[21] Salah Bouktif et al., “Multi-Sequence LSTM-RNN Deep Learning and Metaheuristics for Electric Load Forecasting,” Energies, vol. 13, no. 2, pp. 1-21. Crossref, https://doi.org/10.3390/en13020391
[22] Mr. Kulkarni Sagar S, and Prof. Kahate Sandip A., "Review of a Semantic Approach to Host-Based Intrusion Detection Systems Using Contiguous and Dis-Contiguous System Call Patterns," SSRG International Journal of Computer Science and Engineering , vol. 2, no. 6, pp. 9-12, 2015. Crossref, https://doi.org/10.14445/23488387/IJCSE-V2I6P109
[23] Vulavabeti Raghunath Reddy et al., "Intrusion Detection and Monitoring Using IOT," SSRG International Journal of Electronics and Communication Engineering, vol. 5, no. 9, pp. 13-15, 2018. Crossref, https://doi.org/10.14445/23488549/IJECE-V5I9P103
[24] Ananth, C. A., et al., “Intrusion Detection System for Energy Efficient Cluster Based Vehicular Adhoc Networks,” International Journal of Advanced Computer Science and Applications, vol. 12, no. 10, 2021. Crossref, https://doi.org/10.14569/IJACSA.2021.0121025
[25] Neelu Khare et al., “SMO-DNN: Spider Monkey Optimization and Deep Neural Network Hybrid Classifier Model for Intrusion Detection,” Electronics, vol. 9, no. 4, pp. 1-18, 2020. Crossref, https://doi.org/10.3390/electronics9040692
[26] S.Kavitha et al., "Detecting Network Intrusion Based on Machine Learning Algorithms," International Journal of P2P Network Trends and Technology, vol. 10, no. 3, pp. 1-5, 2020.
[27] Praveensankar Manimaran, and Arun Raj Kumar P.NDNIDS, “An Intrusion Detection System for NDN Based VANET,” 2020 IEEE 91st Vehicular Technology Conference (VTC2020-Spring, IEEE. pp. 1-5, 2020. Crossref, https://doi.org/10.1109/VTC2020- Spring48590.2020.9129365
[28] Tao Zhang, and Quanyan Zhu, “Distributed Privacy-Preserving Collaborative Intrusion Detection Systems for Vanets,” IEEE Transactions on Signal and Information Processing Over Networks, vol. 4, no. 1, pp. 148-161, 2018. Crossref, https://doi.org/10.1109/TSIPN.2018.2801622
[29] Yi Zeng et al., “DeepVCM: A Deep Learning Based Intrusion Detection Method in VANET,” 2019 IEEE 5th Intl Conference on Big Data Security on Cloud (Bigdata security), IEEE Intl Conference on High Performance and Smart Computing,(HPSC) and IEEE Intl Conference on Intelligent Data and Security (IDS), pp. 288-293, 2019. Crossref, https://doi.org/10.1109/BigDataSecurity-HPSC-IDS.2019.00060
[30] Sanjeevaiah Kuraganti, and Jeevana Jyothi. P, “Privacy Preservation Approach Using K-Anonymity Chinese Remainder Theorem for Intrusion Detection,” International Journal of Computer & Organization Trends, vol. 4, no. 5, pp. 31-38, 2014.
[31] FeiWang et al., “A Day-Ahead PV Power Forecasting Method Based on LSTM-RNN Model and Time Correlation Modification Under Partial Daily Pattern Prediction Framework,” Energy Conversion and Management, vol. 212, 2020. Crossref, https://doi.org/10.1016/j.enconman.2020.112766
[32] Alzahrani, A.O., and Alenazi, M.J, “Designing a Network Intrusion Detection System Based on Machine Learning for Software Defined Networks, Future Internet, vol. 13, no. 5, pp. 1-18, 2021. Crossref, https://doi.org/10.3390/fi13050111
[33] Lianbo Ma et al., “Enhancing Learning Efficiency of Brain Storm Optimization via Orthogonal Learning Design,” IEEE Transactions on Systems, Man, and Cybernetics: Systems, vol. 51, no. 11, pp. 6723-6742. Crossref, https://doi.org/10.1109/TSMC.2020.2963943
[34] Noam Shazeer, and Mitchell Stern, “Adafactor: Adaptive Learning Rates With Sublinear Memory Cost,” International Conference on Machine Learning, pp. 4596-4604, 2018.