Deep Learning-Based State-of-Charge Assessment Model for Hybrid Electric Vehicles Energy Management Systems
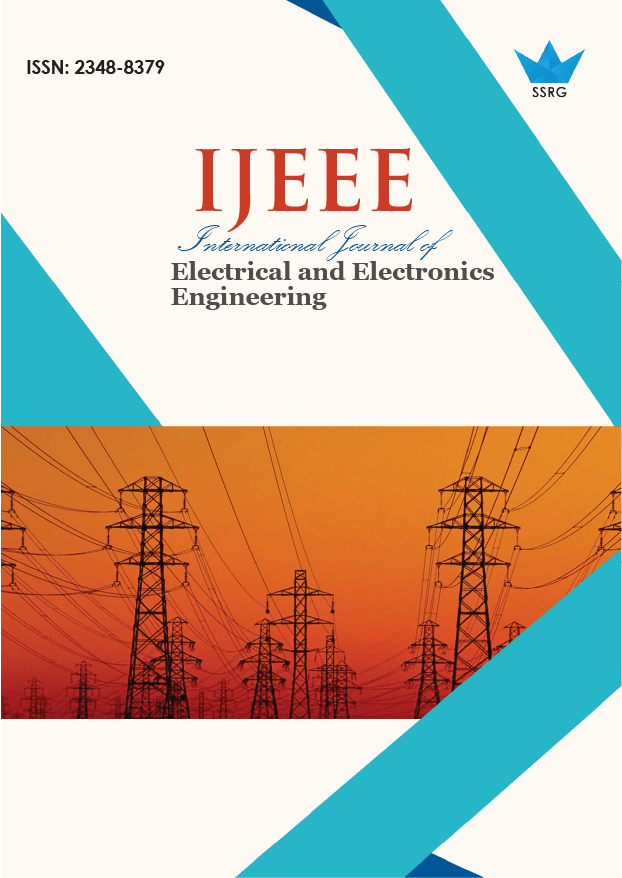
International Journal of Electrical and Electronics Engineering |
© 2023 by SSRG - IJEEE Journal |
Volume 10 Issue 1 |
Year of Publication : 2023 |
Authors : S. Manoj, S. Pradeep Kumar |
How to Cite?
S. Manoj, S. Pradeep Kumar, "Deep Learning-Based State-of-Charge Assessment Model for Hybrid Electric Vehicles Energy Management Systems," SSRG International Journal of Electrical and Electronics Engineering, vol. 10, no. 1, pp. 209-218, 2023. Crossref, https://doi.org/10.14445/23488379/IJEEE-V10I1P120
Abstract:
Promoting the use of Electric Vehicles (EVs) is a practical way to encourage carbon impartiality and thwart the environmental problem. Government regulations and user experiences directly correlate with EV batteries and battery management improvements. Alternative engine technologies have become increasingly important in addressing issues with traditional automobiles in recent years. To decarbonize the transportation industry, electric vehicles are practical solutions. It also becomes crucial to switch from conventional to smart homes and from traditional to EV or HEV vehicles. One of the most vital parts of electric vehicles is the battery. When dealing with larger capacity and high-power needs, high-power providing battery packs—which are made up of many batteries—are necessary. These large battery packs are prone to overheating while being charged and discharged, which can lead to a lot of problems. Consequently, it is imperative to employ a battery management system. It is in charge of optimizing the battery pack so that it functions more effectively and safely. This essay's primary goals are to simulate a Battery Management System (BMS) model and examine several approaches to parameter estimation for a battery management system. It also offers suggestions for the BMS's most effective and economical implementation strategies. An efficient battery management system (BMS), primarily used for signaling the battery level of charge, is still a key component among the numerous HEV technologies (SOC). Since excessive charging and discharging always cause damage to the batteries, the BMS must provide an accurate SOC estimation. Although several SOC prediction strategies are available to control battery cell SOC, HEVs require improved SOC estimation capability. The construction of a unique deep learning with SOC estimate model for safe energy management technique for this is the main emphasis of this paper from this perspective. The proposed model uses a hybrid convolution neural network with long short-term memory (HCL) model to precisely estimate SOC. The HCL model is used to facilitate modeling and provides an accurate representation of the input and output association of the battery model. A detailed experimental investigation showed that the proposed model was superior to other current methods in several ways.
Keywords:
Electric Vehicle, HCL, Battery Management System, SOC, Deep Learning.
References:
[1] Pier Giuseppe Anselma, “Computationally Efficient Evaluation of Fuel and Electrical Energy Economy of Plug-In Hybrid Electric Vehicles with Smooth Driving Constraints,” Applied Energy, vol. 307, pp. 118247, 2022. Crossref, https://doi.org/10.1016/j.apenergy.2021.118247
[2] Chau K.T., Energy Systems for Electric and Hybrid Vehicles, Institution of Engineering and Technology, 2016 Crossref, https://doi.org/10.1049/PBTR002E
[3] Yury Dvorkin et al., “Ensuring Profitability of Energy Storage,” IEEE Transactions on Power Systems, vol. 32, no. 1, pp. 611– 623, 2017. Crossref, https://doi.org/10.1109/TPWRS.2016.2563259
[4] Ridoy Das et al., “Multi-Objective Technoeconomic-Environmental Optimisation Of Electric Vehicle For Energy Services,” Applied Energy, vol. 257, pp. 113965, 2020. Crossref, https://doi.org/10.1016/j.apenergy.2019.113965
[5] DolfGielen et al., “The Role of Renewable Energy in The Global Energy Transformation,” Energy Strategy Reviews, vol. 24, pp. 38– 50, 2019. Crossref, https://doi.org/10.1016/j.esr.2019.01.006
[6] Kailong Liu et al., “A Brief Review on Key Technologies in the Battery Management System of Electric Vehicles,” Frontiers of Mechanical Engineering, vol. 14, no. 1, pp. 47–64, 2019. Crossref, https://doi.org/10.1007/s11465-018-0516-8
[7] Nisha C. Rani, and N Amuthan, "Soft-Switching Integrated Quasi Resonance Buck-Boost Converter for HHO Optimized Grid Connected PV System," SSRG International Journal of Electrical and Electronics Engineering, vol. 9, no. 8, pp. 28-39, 2022. Crossref, https://doi.org/10.14445/23488379/IJEEE-V9I8P104
[8] R. Venkedesh, R. Anandha Kumar, and G. Renukadevi, "Multilevel Inverter Design with Reduced Switches & THD Using Fuzzy Logic Controller," SSRG International Journal of Electrical and Electronics Engineering, vol. 9, no. 12, pp. 1-21, 2022. Crossref, https://doi.org/10.14445/23488379/IJEEE-V9I12P101
[9] Farithkhan Abbas Ali, and E. D. Kanmani Ruby, "Clustering Metric Algorithm for Cost-Effective Routing in Flying Ad-hoc Networks," SSRG International Journal of Electrical and Electronics Engineering, vol. 9, no. 12, pp. 101-108, 2022. Crossref, https://doi.org/10.14445/23488379/IJEEE-V9I12P108
[10] Murali Matcha et al., "Design and Performance Analysis of Multilayer Neural Network-based Battery Energy Storage System for Enhancing Demand Side Management," SSRG International Journal of Electrical and Electronics Engineering, vol. 9, no. 10, pp. 7- 13, 2022. Crossref, https://doi.org/10.14445/23488379/IJEEE-V9I10P102
[11] Xiong, Rui et al., “Co-Estimation of State of Charge and Capacity for Lithium-Ion Batteries with Multi-Stage Model Fusion Method,” Engineering, vol. 7, no. 10, pp. 1469–1482, 2021. Crossref, https://doi.org/10.1016/j.eng.2020.10.022
[12] Chen, Xiaopeng et al., “Robust Adaptive Sliding-Mode Observer Using RBF Neural Network for Lithium-Ion Battery State of Charge Estimation in Electric Vehicles,” IEEE Transactions on Vehicular Technology, vol. 65, no. 4, pp. 1936–1947, 2015. Crossref, https://doi.org/10.1109/TVT.2015.2427659
[13] Thiruvonasundari Duraisamy, and Deepa Kaliyaperumal, “Machine Learning-Based Optimal Cell Balancing Mechanism for Electric Vehicle Battery Management System”, IEEE Access, vol. 9, pp. 132846-132861, 2021. Crossref, https://doi.org/10.1109/ACCESS.2021.3115255
[14] S. Gopiya Naik et al., "Battery Parameter Monitoring and Control System for Electric Vehicles," SSRG International Journal of Electrical and Electronics Engineering, vol. 9, no. 3, pp. 1-6, 2022. Crossref, https://doi.org/10.14445/23488379/IJEEE-V9I3P101
[15] Zhang Ming, Wang Kai, and Yan-Ting Zhou, “Online State of Charge Estimation of Lithium-Ion Cells Using Particle Filter-Based Hybrid Filtering Approach,” Complexity, pp. 1–10, 2020. Crossref, https://doi.org/10.1155/2020/8231243
[16] Zahid, Taimoor et al., “State of Charge Estimation for Electric Vehicle Power Batteries Using Advanced Machine Learning Algorithms under Diversified Drive Cycles,” Energy, vol. 162, no. 1, pp. 871–882, 2018. Crossref, https://doi.org/10.1016/j.energy.2018.08.071
[17] Lai, Xin et al., “A Hybrid State-of-Charge Estimation Method Based on Credible Increment for Electric Vehicle Applications with Large Sensor and Model Errors,” Journal of Energy Storage, vol. 27, pp. 101106, 2020. Crossref, https://doi.org/10.1016/j.est.2019.101106
[18] Ravindra Panchariya, and Dr. Poonam Syal, "An Improved Current Control Charging Scheme Using Neuro-fuzzy and Fopid Based Mppt System for EV Charging," International Journal of Engineering Trends and Technology, vol. 69, no. 10, pp. 251-257, 2021. Crossref, https://doi.org/10.14445/22315381/IJETT-V69I10P232
[19] Eric Paglia, and Charles Parker, “The Intergovernmental Panel on Climate Change: Guardian of Climate Science,” Guardians of Public Value, pp. 295–321, 2021. Crossref, https://doi.org/10.1007/978-3-030-51701-4_12
[20] Wei He et al., “Optimal Analysis of a Hybrid Renewable Power System for a Remote Island,” Renewable Energy, vol. 179, pp. 96– 104, 2021. Crossref, https://doi.org/10.1016/j.renene.2021.07.034
[21] Alberto Boretti, and Stefania Castelletto, “Cost and Performance of CSP and PV Plants of Capacity above 100 MW Operating in the United States of America,” Renewable Energy Focus, vol. 39, pp. 90–98, 2021. Crossref, https://doi.org/10.1016/j.ref.2021.07.006
[22] Octavio Alves et al., “Techno-Economic Study For a Gasification Plant Processing Residues of Sewage Sludge and Solid Recovered Fuels,” Waste Management, vol. 131, pp. 148–162, 2021. Crossref, https://doi.org/10.1016/j.wasman.2021.05.026
[23] J. Chatzakis et al., “Designing a New Generalized Battery Management System,” IEEE Transactions on Industrial Electronics, vol. 50, no. 5, pp. 990–999, 2003. Crossref, https://doi.org/10.1109/TIE.2003.817706
[24] R Sivapriyan et al., "Comprehensive Review on State of Charge Estimation in Battery Management System" International Journal of Engineering Trends and Technology, vol. 70, no. 7, pp. 169-179, 2022. Crossref, https://doi.org/10.14445/22315381/IJETT-V70I7P218
[25] Z. Cheng-Ning et al., “Design on the Dispersed Management System for the Traction Battery Pack in Electric Transmission Vehicle,” Acta Armamentarii, vol. 28, no. 4, pp. 396–398, 2017.
[26] Min Luo et al., “Online Battery Monitoring System Based on GPRS for Electric Vehicles,” 2013 5th International Conference on Intelligent Human-Machine Systems and Cybernetics, pp. 122-125, 2013. Crossref, https://doi.org/10.1109/IHMSC.2013.36
[27] G. Muneeswari et al., "Urban Computing: Recent Developments and Analytics Techniques in Big Data" International Journal of Engineering Trends and Technology, vol. 70, no. 7, pp. 158-168, 2022. Crossref, https://doi.org/10.14445/22315381/IJETT-V70I7P217
[28] Venkatesan Chandran et al., “State of Charge Estimation of Lithium-Ion Battery for Electric Vehicles Using Machine Learning Algorithms,” World Electric Vehicle Journal, vol. 12, no. 1, pp. 38, 2021. Crossref, https://doi.org/10.3390/wevj12010038
[29] Dickson N.T. How et al., “State-of-Charge Estimation of Li-Ion Battery In Electric Vehicles: A Deep Neural Network Approach,” IEEE Transactions on Industry Applications. Vol. 56, pp. 5565–5574, 2020. Crossref, https://doi.org/10.1109/TIA.2020.3004294
[30] R.Jeyapandi Prathap et al., "A New Resonant Converter Topology for Ev," SSRG International Journal of Electronics and Communication Engineering, vol. 9, no. 3, pp. 9-15, 2022. Crossref, https://doi.org/10.14445/23488549/IJECE-V9I3P102
[31] Yuanliang Fan et al., “Data-driven State-of-Charge Estimation of Lithium-Ion Batteries,” International Conference on Power Electronics Systems and Application, pp. 1-5, 2021. Crossref, https://doi.org/10.1109/PESA50370.2020.9344017
[32] Kailong Li et al., “A Data-Driven Approach With Uncertainty Quantification for Predicting Future Capacities and Remaining Useful Life of Lithium-ion Battery,” IEEE Transactions On Industrial Electronics, vol. 68, no. 4, pp. 3170-3180, 2021. Crossref, https://doi.org/10.1109/TIE.2020.2973876
[33] Changhao Piao et al., “VRLA Battery Management System Based on LIN bus for electric vehicle,” Advanced Technology in Teaching, vol. 163, pp. 753–763, 2012. Crossref, https://doi.org/10.1007/978-3-642-29458-7_105
[34] Arigela Satya Veerendra et al., “Hybrid Power Management for Fuel Cell/Supercapacitor Series Hybrid Electric Vehicle,” International Journal of Green Energy, vol. 18, no. 2, pp. 128–143, 2020. Crossref, https://doi.org/10.1080/15435075.2020.1831511