An Optimized Hyper Parameter Tuned Convolution Neural Frame for Potato Leaves Disease Prediction
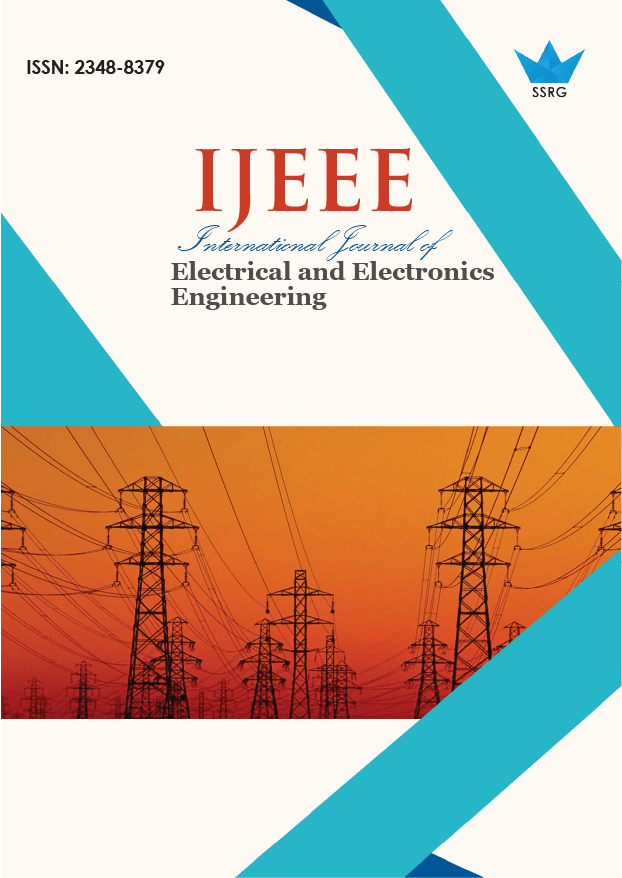
International Journal of Electrical and Electronics Engineering |
© 2023 by SSRG - IJEEE Journal |
Volume 10 Issue 2 |
Year of Publication : 2023 |
Authors : Anuj Rapaka, A. Clara Kanmani |
How to Cite?
Anuj Rapaka, A. Clara Kanmani, "An Optimized Hyper Parameter Tuned Convolution Neural Frame for Potato Leaves Disease Prediction," SSRG International Journal of Electrical and Electronics Engineering, vol. 10, no. 2, pp. 180-195, 2023. Crossref, https://doi.org/10.14445/23488379/IJEEE-V10I2P117
Abstract:
Image processing is an exciting concept in several digital applications for identifying features precisely. Hence, this technology is chiefly utilized in agriculture applications to predict the disease affection in plat and leaves. However, the complex image has reduced the exactness rate of classification and segmentation. In this present work, the potato leaves are normal, and disease images are considered. Hence, a novel Ant Lion-based Hyper-Parameter tuned Convolution Neural Approach (ALHTCNA) has been designed with the required parameters to maximize the exactness score. Initially, the preprocessing function was activated to obtain error-free data. Consequently, the error-free data is moved to the classification frame, in that feature extraction, segmentation, and disease classification process has functioned. The considered disease types in the present work are Early-Blight (EB) and Late-Blight (LB). If the tested data did not contain these disease features, it is considered healthy leaves. Moreover, the planned design is implemented in the python platform, and the metrics are validated and compared with other schemes and has observed the finest segmentation and specification exactness score.
Keywords:
Image processing, Segmentation, Feature extraction, Potato leaves data, Disease specification.
References:
[1] Md. Al-Amin, Dewan Ziaul Karim, and Tasfia Anika Bushra, "Prediction of Rice Disease from Leaves Using Deep Convolution Neural Network Towards a Digital Agricultural System," 22nd International Conference on Computer and Information Technology, IEEE, pp. 1-5, 2019. Crossref, http://doi.org/10.1109/ICCIT48885.2019.9038229
[2] Sk Mahmudul Hassan et al., "Identification of Plant-Leaf Diseases Using CNN and Transfer-Learning Approach," Electronics, vol. 10, no. 12, p. 1388, 2021. Crossref, https://doi.org/10.3390/electronics10121388
[3] K. S. Chethan et al., "Mobile Application for Classification of Plant Leaf Diseases Using Image Processing and Neural Networks," Data Intelligence and Cognitive Informatics, Springer, Singapore, pp. 287-306, 2021. Crossref, https://doi.org/10.1007/978-981-15-8530- 2_22
[4] Ruchi Gajjar et al., "Real-Time Detection and Identification of Plant Leaf Diseases Using Convolutional Neural Networks on an Embedded Platform," The Visual Computer, pp. 2923-2938, 2022. Crossref, https://doi.org/10.1007/s00371-021-02164-9
[5] Ravindra Namdeorao Jogekar, and Tiwari Nandita, "A Review of Deep Learning Techniques for Identification and Diagnosis of Plant Leaf Disease," Smart Trends in Computing and Communications: Proceedings of SmartCom, vol. 182, pp. 435-441, 2021. Crossref, https://doi.org/10.1007/978-981-15-5224-3_43
[6] M. Karthick et al., "Machine Learning Approaches for Automatic Disease Detection from Paddy Crops - A Review," International Journal of Engineering Trends and Technology, vol. 70, no. 12, pp. 392-405, 2022. Crossref, https://doi.org/10.14445/22315381/IJETT-V70I12P237
[7] Mohamed Loey, "Big Data and Deep Learning in Plant Leaf Diseases Classification for Agriculture," Enabling AI Applications in Data Science, Springer, Cham, pp. 185-200, 2021. Crossref, https://doi.org/10.1007/978-3-030-52067-0_8
[8] A. Lakshmanarao, M. Raja Babu, and T. Srinivasa Ravi Kiran, "Plant Disease Prediction and Classification using Deep Learning ConvNets," International Conference on Artificial Intelligence and Machine Vision, IEEE, pp. 1-6, 2021. Crossref, https://doi.org/10.1109/AIMV53313.2021.9670918
[9] Man Gong et al., "Mechanism by Which Eucommiaulmoides Leaves Regulate Nonalcoholic Fatty Liver Disease Based on System Pharmacology," Journal of Ethnopharmacology, vol. 282, p. 114603, 2022. Crossref, https://doi.org/10.1016/j.jep.2021.114603
[10] Ravindra Jogekar, and Nandita Tiwari, "Enhanced Adaptive Creation of Visualisation Network by Detection of Leaves," Materials Today: Proceedings, 2021. Crossref, https://doi.org/10.1016/j.matpr.2021.01.506
[11] Tahmina Tashrif Mim et al., "Leaves Diseases Detection of Tomato Uusing Image Processing," 8th International Conference System Modeling and Advancement in Research Trends, IEEE, pp. 244-249, 2019. Crossref, https://doi.org/10.1109/SMART46866.2019.9117437
[12] D. Sheema et al., "The Detection and Identification of Pest-FAW Infestation in Maize Crops Using Iot-Based Deep-Learning Algorithm," SSRG International Journal of Electrical and Electronics Engineering, vol. 9, no. 12, pp. 180-188, 2022. Crossref, https://doi.org/10.14445/23488379/IJEEE-V9I12P116
[13] H. Suheri, and T. V. Price, "Infection of Onion Leaves by Alternariaporri and Stemphyliumvesicarium and Disease Development in Controlled Environments," Plant Pathology, vol. 49, no. 3, pp. 375-382, 2000. Crossref, https://doi.org/10.1046/j.1365- 3059.2000.00458.x
[14] G. Geetharamani, and J. Arun Pandian, "Identification of Plant Leaf Diseases using a Nine-Layer Deep Convolutional Neural Network," Computers & Electrical Engineering, vol. 76, pp. 323-338, 2019. Crossref, https://doi.org/10.1016/j.compeleceng.2019.04.011
[15] Rina Mahakud, Binod Kumar Pattanayak, and Bibudhendu Pati, "A Hybrid Multi-class Classification Model for the Detection of Leaf Disease using XGBoost and SVM," International Journal of Engineering Trends and Technology, vol. 70, no. 10, pp. 298-306, 2022. Crossref, https://doi.org/10.14445/22315381/IJETT-V70I10P229
[16] S. Ramesh, and D. Vydeki, "Recognition and Classification of Paddy Leaf Diseases using Optimized Deep Neural Network with Jaya Algorithm," Information Processing in Agriculture, vol. 7, no. 2, pp. 249-260, 2020. Crossref, https://doi.org/10.1016/j.inpa.2019.09.002
[17] Sammy V. Militante, Bobby D. Gerardo, and Nanette V. Dionisio, "Plant Leaf Detection and Disease Recognition using Deep Learning," IEEE Eurasia Conference on IOT, Communication and Engineering, IEEE, pp. 579-582, 2019. Crossref, https://doi.org/10.1109/ECICE47484.2019.8942686
[18] Santosh Kumar Upadhyay, and Avadhesh Kumar, "A Novel Approach for Rice Plant Diseases Classification with Deep Convolutional Neural Network," International Journal of Information Technology, pp. 185-199, 2022. Crossref, https://doi.org/10.1007/s41870-021- 00817-5
[19] Gargi Sharma, and Gourav Shrivastava, "Crop Disease Prediction using Deep Learning Techniques - A Review," SSRG International Journal of Computer Science and Engineering, vol. 9, no. 4, pp. 23-28, 2022. Crossref, https://doi.org/10.14445/23488387/IJCSE-V9I4P104
[20] R. Sujatha et al., "Performance of Deep Learning vs Machine Learning in Plant Leaf Disease Detection," Microprocessors and Microsystems, vol. 80, p. 103615, 2021. Crossref, https://doi.org/10.1016/j.micpro.2020.103615
[21] Zhencun Jiang et al., "Recognition of Rice Leaf Diseases and Wheat Leaf Diseases Based on Multi-Task Deep Transfer Learning," Computers and Electronics in Agriculture, vol. 186, no. 1, p. 106184, 2021. Crossref, https://doi.org/10.1016/j.compag.2021.106184
[22] N. Krishnamoorthy et al., "Rice Leaf Diseases Prediction Using Deep Neural Networks with Transfer Learning," Environmental Research, vol. 198, p. 111275, 2021. Crossref, https://doi.org/10.1016/j.envres.2021.111275
[23] Reem Issa, Mitiady Boras, and Riad Zidan, "Effect of Seaweed Extract on the Growth and Productivity of Potato Plants," SSRG International Journal of Agriculture & Environmental Science, vol. 6, no. 2, pp. 83-89, 2019. Crossref, https://doi.org/10.14445/23942568/IJAES-V6I2P116
[24] Kshyanaprava Panda Panigrahi et al., "Maize Leaf Disease Detection and Classification Using Machine Learning Algorithms," Progress in Computing, Analytics and Networking, Springer, Singapore, pp. 659-669, 2020. Crossref, https://doi.org/10.1007/978-981-15-2414- 1_66
[25] Somnath Mukhopadhyay et al., "Tea Leaf Disease Detection Using Multi-Objective Image Segmentation," Multimedia Tools and Applications, vol. 80, no. 1, pp. 753-771, 2021. Crossref, https://doi.org/10.1007/s11042-020-09567-1
[26] T. Nagarathinam, and Dr. K. Rameshkumar, "A Survey on Cluster Analysis Techniques for Plant Disease Diagnosis," SSRG International Journal of Computer Science and Engineering, vol. 3, no. 6, pp. 11-17, 2016. Crossref, https://doi.org/10.14445/23488387/IJCSE-V3I6P103
[27] Dalwinder Singh, and Birmohan Singh, "Effective and Efficient Classification of Gastrointestinal Lesions: Combining Data Preprocessing, Feature Weighting, and Improved Ant Lion Optimization," Journal of Ambient Intelligence and Humanized Computing, vol. 12, no. 9, pp. 8683-8698, 2021. Crossref, https://doi.org/10.1007/s12652-020-02629-0
[28] R. Tamilaruvi et al., "Brain Tumor Detection in MRI Images using Convolutional Neural Network Technique," SSRG International Journal of Electrical and Electronics Engineering, vol. 9, no. 12, pp. 198-208, 2022. Crossref, https://doi.org/10.14445/23488379/IJEEE-V9I12P118
[29] Chandrasen Pandey et al., "Machine Learning Approach for Automatic Diagnosis of Chlorosis in Vignamungo Leaves," Multimedia Tools and Applications, vol. 80, no. 9, pp. 13407-13427, 2021. Crossref, https://doi.org/10.1007/s11042-020-10309-6
[30] Ümit Atila et al., "Plant Leaf Disease Classification Using Efficientnet Deep Learning Model," Ecological Informatics, vol. 61, p. 101182, 2021. Crossref, https://doi.org/10.1016/j.ecoinf.2020.101182
[31] Siddharth Singh Chouhan et al., "Bacterial Foraging Optimization Based Radial Basis Function Neural Network (BRBFNN) for Identification and Classification of Plant Leaf Diseases: An Automatic Approach towards Plant Pathology," IEEE Access, vol. 6, pp. 8852- 8863, 2018. Crossref, https://doi.org/10.1109/ACCESS.2018.2800685
[32] Nour Eldeen M. Khalifa et al., "Artificial Intelligence in Potato Leaf Disease Classification: A Deep Learning Approach," Machine Learning and Big Data Analytics Paradigms: Analysis, Applications and Challenges, Springer, Cham, pp. 63-79, 2021. Crossref, https://doi.org/10.1007/978-3-030-59338-4_4
[33] Henan Sun et al., "MEAN-SSD: A Novel Real-Time Detector for Apple Leaf Diseases using Improved Light-Weight Convolutional Neural Networks," Computers and Electronics in Agriculture, vol. 189, p. 106379, 2021. Crossref, https://doi.org/10.1016/j.compag.2021.106379