An Ensemble of Deep Learning with Optimization Model for Activity Recognition in the Internet of Things Environment
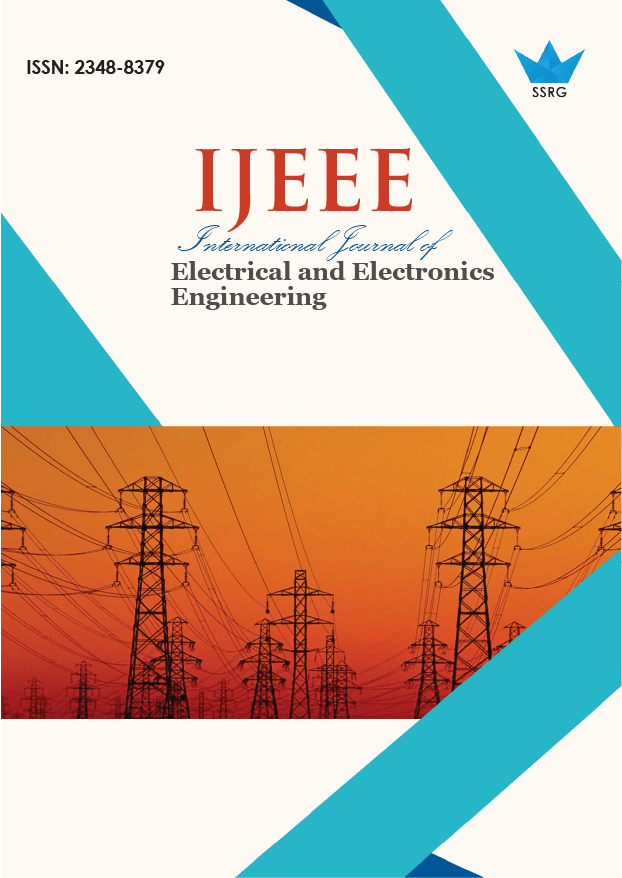
International Journal of Electrical and Electronics Engineering |
© 2023 by SSRG - IJEEE Journal |
Volume 10 Issue 4 |
Year of Publication : 2023 |
Authors : C. Nithyeswari, G. Karthikeyan |
How to Cite?
C. Nithyeswari, G. Karthikeyan, "An Ensemble of Deep Learning with Optimization Model for Activity Recognition in the Internet of Things Environment," SSRG International Journal of Electrical and Electronics Engineering, vol. 10, no. 4, pp. 91-104, 2023. Crossref, https://doi.org/10.14445/23488379/IJEEE-V10I4P109
Abstract:
Lately, IoT (Internet of Things) based m-healthcare application is rising to give real-time servicing in the present global lifestyle. Cloud-based healthcare architecture provides best results than traditional approaches. Currently, Integrating IoT devices in the medical environment plays a crucial role in handling a massive amount of medical data. Thus, researcher workers aimed to automate the procedure of diagnosis and detecting diseases with the help of could computing techniques. Furthermore, deep learning and machine learning techniques used in the healthcare field allow healthcare professionals to focus, monitor, highlight and diagnose the region of the problem and present the accurate and required solution in a short duration. Therefore, this paper presents Artificial Bee Colony Optimization with Ensemble Deep Learning based Disease Diagnosis (ABCO-EDLDD) in the IoT atmosphere. The proposed ABCO-EDLDD procedure effectively identifies the existence of diseases in the IoT atmosphere. At the initial stage, the ABCO-EDLDD technique transforms the input data gathered by the IoT devices in different ways. Next, the ABCO algorithm is utilized for the optimal selection of feature subsets. For disease classification, an ensemble of DL models such as Gated Recurrent Unit (GRU), Long Short Term Memory (LSTM), and Bidirectional Long Short Term Memory (BiLSTM) model. Finally, the RMSProp optimizer is used for the optimum tuning of the DL models. The experimental evaluation of the ABCO-EDLDD algorithm takes place by implementing two medical datasets: the HAPT dataset and the heart disease dataset. The experimental results reported the improved performance of the ABCO-EDLDD procedure over other current techniques.
Keywords:
Healthcare, Internet of Things, Deep learning, Disease diagnosis, Feature selection, Ensemble learning.
References:
[1] Priyan Malarvizhi Kumar et al., “Cloud and IoT Based Disease Prediction and Diagnosis System for Healthcare Using Fuzzy Neural Classifier,” Future Generation Computer Systems, vol. 86, pp. 527-534, 2018.
[CrossRef] [Google Scholar] [Publisher Link]
[2] Mohammad Ayoub Khan, and Fahad Algarni, “A Healthcare Monitoring System for the Diagnosis of Heart Disease in the IoMT Cloud Environment Using MSSO-ANFIS,” IEEE Access, vol. 8, pp. 122259-122269, 2020.
[CrossRef] [Google Scholar] [Publisher Link]
[3] Wei Li et al., “A Comprehensive Survey on Machine Learning-Based Big Data Analytics for IoT-Enabled Smart Healthcare System,” Mobile Networks and Applications, vol. 26, no. 1, pp. 234-252, 2021.
[CrossRef] [Google Scholar] [Publisher Link]
[4] Ahmed Abdelaziz et al., “A Machine Learning Model for Predicting of Chronic Kidney Disease Based Internet of Things and Cloud Computing In Smart Cities,” Security In Smart Cities: Models, Applications, and Challenges, pp. 93-114, 2018.
[CrossRef] [Google Scholar] [Publisher Link]
[5] Neha Agarwal et al., “Machine Learning Applications for IoT Healthcare,” Machine Learning Approaches for Convergence of IoT and Blockchain, pp.129-144, 2021.
[CrossRef] [Google Scholar] [Publisher Link]
[6] Farzad Samie, Lars Bauer, and Jörg Henkel, “From Cloud Down to Things: An Overview of Machine Learning in Internet of Things,” IEEE Internet of Things Journal, vol. 6, no. 3, pp. 4921-4934, 2019.
[CrossRef] [Google Scholar] [Publisher Link]
[7] S.M. Zobaed et al., Deep Learning in IoT-Based Healthcare Applications, Deep Learning for Internet of Things Infrastructure, 1st Edition, p. 18, 2021.
[Google Scholar] [Publisher Link]
[8] Shaik Abdul Khalandar Basha et al., "A Novel Approach To Analysis Consequence of Climate Changes on Erythemato-Squamous Diseases Using Machine Learning Algorithms," International Journal of Engineering Trends and Technology, vol. 70, no. 11, pp. 10- 18, 2022.
[CrossRef] [Publisher Link]
[9] Maamar Ali Saud AL Tobi et al., "Machinery Faults Diagnosis Using Support Vector Machine (SVM) and Naïve Bayes Classifiers," International Journal of Engineering Trends and Technology, vol. 70, no. 12, pp. 26-34, 2022.
[CrossRef] [Google Scholar] [Publisher Link]
[10] Stanislav Yamashkin et al., "Metageosystem Analysis Based on a System of Machine Learning and Simulation Algorithms," International Journal of Engineering Trends and Technology, vol. 70, no. 12, pp. 1-12, 2022.
[CrossRef] [Publisher Link]
[11] Pramila Arulanthu, and Eswaran Perumal, “An Intelligent IoT with Cloud Centric Medical Decision Support System for Chronic Kidney Disease Prediction,” International Journal of Imaging Systems and Technology, vol. 30, no. 3, pp. 815-827, 2020.
[CrossRef] [Google Scholar] [Publisher Link]
[12] A Angel Nancy et al., “IoT-Cloud-Based Smart Healthcare Monitoring System for Heart Disease Prediction via Deep Learning,” Electronics, vol. 11, no. 15, p. 1-19, 2022.
[CrossRef] [Google Scholar] [Publisher Link]
[13] Sushmitha.J, et al., "Patient Medical Checkup Using Webapp and IOT," SSRG International Journal of Computer Science and Engineering, vol. 5, no. 8, pp. 15-18, 2018.
[CrossRef] [Publisher Link]
[14] Arun Das et al., “Distributed Machine Learning Cloud Teleophthalmology IoT for Predicting AMD Disease Progression,” Future Generation Computer Systems, vol. 93, pp.486-498, 2019.
[CrossRef] [Google Scholar] [Publisher Link]
[15] Priyan Malarvizhi Kumar, “Cloud-and IoT-Based Deep Learning Technique-Incorporated Secured Health Monitoring System for Dead Diseases,” Soft Computing, vol. 25, no. 18, pp.12159-12174, 2021.
[CrossRef] [Google Scholar] [Publisher Link]
[16] Samira Akhbarifar et al., “A Secure Remote Health Monitoring Model for Early Disease Diagnosis in Cloud-Based IoT Environment,” Personal and Ubiquitous Computing, pp. 1-17, 2020.
[CrossRef] [Google Scholar] [Publisher Link]
[17] Mezui Eya’a Guy Lysmos, and Dr.Mostafa Hanoune, "Hybrid Data Compression System In Smart E-Health Gateway for Medical Monitoring Applications," SSRG International Journal of Computer Science and Engineering, vol. 7, no. 1, pp. 1-6, 2020.
[CrossRef] [Google Scholar] [Publisher Link]
[18] Shreshth Tuli et al., “Healthfog: An Ensemble Deep Learning Based Smart Healthcare System for Automatic Diagnosis of Heart Diseases in Integrated IoT and Fog Computing Environments,” Future Generation Computer Systems, vol. 104, pp. 187-200, 2020.
[CrossRef] [Google Scholar] [Publisher Link]
[19] Shuchi Juyal, Sachin Sharma, and Amal Shankar Shukla, “Smart Skin Health Monitoring Using AI-Enabled Cloud-Based IoT,” Materials Today: Proceedings, vol. 46, pp.10539-10545, 2021.
[CrossRef] [Google Scholar] [Publisher Link]
[20] Dr. Dervis Karaboga, “Artificial Bee Colony Algorithm,” Scholarpedia, vol. 5, no. 3, 2010.
[CrossRef] [Google Scholar] [Publisher Link]
[21] Ezgi Zorarpacı, and Selma Ayşe Özel, “Privacy Preserving Rule-Based Classifier Using Modified Artificial Bee Colony Algorithm,” Expert Systems with Applications, vol. 183, 2021.
[CrossRef] [Google Scholar] [Publisher Link]
[22] Yawen Xiao et al., “A Deep Learning-Based Multi-Model Ensemble Method for Cancer Prediction,” Computer Methods and Programs in Biomedicine, vol. 153, pp.1-9, 2018.
[CrossRef] [Google Scholar] [Publisher Link]
[23] Muhammad Zulqarnain et al., "IOT for Healthcare," SSRG International Journal of Electronics and Communication Engineering, vol. 5, no. 11, pp. 1-5, 2018.
[CrossRef] [Publisher Link]
[24] Muhammad Zulqarnain et al., “Efficient Processing of GRU Based on Word Embedding for Text Classification,” JOIV: International Journal on Informatics Visualization, vol. 3, no. 4, pp. 377-383, 2019.
[CrossRef] [Google Scholar] [Publisher Link]
[25] Suvidha Tripathi et al., “An End-To-End Breast Tumour Classification Model Using Context-Based Patch Modelling–A Bilstm Approach for Image Classification,” Computerized Medical Imaging and Graphics, vol. 87, 2021.
[CrossRef] [Google Scholar] [Publisher Link]
[26] Fangyu Zou et al., “A Sufficient Condition for Convergences of Adam and RMSprop,” In Proceedings of the IEEE/CVF Conference on Computer Vision and Pattern Recognition, pp. 11127-11135.
[CrossRef] [Google Scholar] [Publisher Link]
[27] Activity Recognition Dataset. [Online]. Available:
https://archive.ics.uci.edu/ml/datasets/smartphone-based+recognition+of+human+activities+and+postural+transitions
[28] Sakil Ahammed et al., "An IoT-Based Real-Time Remote Health Monitoring System," International Journal of Recent Engineering Science, vol. 8, no. 3, pp. 23-29, 2021.
[CrossRef] [Google Scholar] [Publisher Link]
[29] Heart Disease Dataset. [Online]. available: https://archive.ics.uci.edu/ml/datasets/heart+disease
[30] Miguel Altuve et al., “Human Activity Recognition Using Improved Complete Ensemble EMD with Adaptive Noise and Long Short-Term Memory Neural Networks,” Biocybernetics and Biomedical Engineering, vol. 40, no. 3, pp.901-909, 2020.
[CrossRef] [Google Scholar] [Publisher Link]
[31] Ohoud Nafea et al., “Sensor-Based Human Activity Recognition with Spatio-Temporal Deep Learning,” Sensors, vol. 21, no. 6, pp. 1- 20, 2021.
[CrossRef] [Google Scholar] [Publisher Link]
[32] Mohammad Shafenoor Amin, Yin Kia Chiam, and Kasturi Dewi Varathan, “Identification of Significant Features and Data Mining Techniques In Predicting Heart Disease,” Telematics and Informatics, vol. 36, pp. 82-93, 2019.
[CrossRef] [Google Scholar] [Publisher Link]
[33] C. Nithyeswari, and Dr.G. Karthikeyan, “Design of Optimal Deep Learning Based Disease Diagnosis Model for Cloud Centric IoT Healthcare Environment,” Turkish Journal of Physiotherapy and Rehabilitation, vol. 32, no. 3, 2021.
[Google Scholar]