Spotted Hyena Optimization with Deep Learning-Based Automatic Text Document Summarization Model
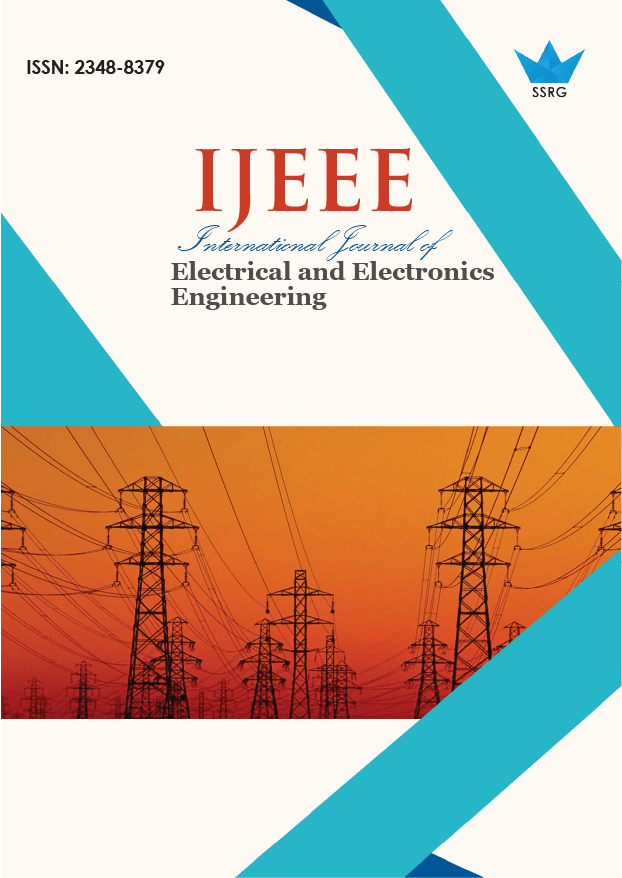
International Journal of Electrical and Electronics Engineering |
© 2023 by SSRG - IJEEE Journal |
Volume 10 Issue 5 |
Year of Publication : 2023 |
Authors : A. Leoraj, M. Jeyakarthic |
How to Cite?
A. Leoraj, M. Jeyakarthic, "Spotted Hyena Optimization with Deep Learning-Based Automatic Text Document Summarization Model," SSRG International Journal of Electrical and Electronics Engineering, vol. 10, no. 5, pp. 153-164, 2023. Crossref, https://doi.org/10.14445/23488379/IJEEE-V10I5P114
Abstract:
Automatic text summarization is an active investigation region determined as removing snippets or introductory sentences of a massive document and relating them as a short form of documents. Text Summarization can be either costefficient or time-efficient. An abstractive or extractive summary was studied with distinct algorithms comprising deep learning (DL), graph, and statistical-based techniques. DL has attained promising shows in comparison to the typical methods. With the development of various neural structures like the attention mechanism (usually called a transformer), there is a potential growth area for summarization tasks. Hence, this research presents a Spotted Hyena Optimization with Deep Learning based Automatic Text Summarization (SHODL-ATS) model. The SHODL-ATS technique's principal objective lies in the documents' automated summarization. To accomplish this, the presented SHODL-ATS technique performs data preprocessing to convert the data into a convenient form. The SHODL-ATS technique uses an Attention-based Bidirectional Gated Recurrent Unit (ABiGRU) model for summarizing the text documents. Finally, the SHO technique is enforced for the parameter tuning of the ABiGRU approach. To examine the achievement of the SHODL-ATS model, we validate the outcomes on benchmark datasets. The results indicate the promising achievement of the SHODL-ATS method over other existing techniques.
Keywords:
Text summarization, Spotted Hyena optimizer, Deep learning, Natural language processing.
References:
[1] Akim Tsvigun et al., “Active Learning for Abstractive Text Summarization,” Computation and Language, 2022.
[CrossRef][Google Scholar][Publisher Link]
[2] Ayham Alomari et al., “Deep Reinforcement and Transfer Learning for Abstractive Text Summarization: A Review,” Computer Speech and Language, vol. 71, 2022.
[CrossRef][Google Scholar][Publisher Link]
[3] Abdul Ghafoor Etemad, Ali Imam Abidi, and Megha Chhabra, “A Review on Abstractive Text Summarization using Deep Learning,” In 2021 9th International Conference on Reliability, Infocom Technologies and Optimization, pp. 1-6, 2021.
[CrossRef][Google Scholar][Publisher Link]
[4] Nida Shafiq et al., “Abstractive Text Summarization of Low-Resourced Languages using Deep Learning,” PeerJ Computer Science, vol. 9, pp. 1-22, 2023.
[CrossRef][Google Scholar][Publisher Link]
[5] Fatih Ertam, and Galip Aydin, “Abstractive Text Summarization using Deep Learning with a New Turkish Summarization Benchmark Dataset,” Concurrency and Computation: Practice and Experience, vol. 34, no. 9, pp. e6482, 2022.
[CrossRef][Google Scholar][Publisher Link]
[6] Yash Kumar Atri et al., “See, Hear, Read: Leveraging Multimodality with Guided Attention for Abstractive Text Summarization,” Knowledge-Based Systems, vol. 227, pp. 107152, 2021.
[CrossRef][Google Scholar][Publisher Link]
[7] K. Kavitha, “Optimal Installation for Enhancing Power System Security using Integrated BBO-PSO Techniques,” In 2022 International Conference on Automation, Computing and Renewable Systems, India, pp. 426-432, 2022.
[CrossRef][Google Scholar][Publisher Link]
[8] M. Jeyakarthic, and J. Senthilkumar, “Optimal Bidirectional Long Short Term Memory based Sentiment Analysis with Sarcasm Detection and Classification on Twitter Data,” In 2022 IEEE 2nd Mysore Sub Section International Conference (MysuruCon), India, pp. 1-6, 2022.
[CrossRef][Google Scholar][Publisher Link]
[9] C. Nithyeswari, and G. Karthikeyan, “An Ensemble of Deep Learning with Optimization Model for Activity Recognition in the Internet of Things Environment,” SSRG International Journal of Electrical and Electronics Engineering, vol. 10, no. 4, pp. 91-104, 2023.
[CrossRef][Publisher Link]
[10] V. Sujatha Bai, and M. Punithavalli, “Evolutionary Optimization Algorithm with Deep Echo State Network for Anomaly Detection on Secure Cloud Computing Environment,” SSRG International Journal of Electrical and Electronics Engineering, vol. 10, no. 4, pp. 46-56, 2023.
[CrossRef][Google Scholar][Publisher Link]
[11] Ridam Srivastava et al., “A Topic-Modelled Unsupervised Approach to Single Document Extractive Text Summarization,” Knowledge-Based Systems, vol. 246, 2022.
[CrossRef][Google Scholar][Publisher Link]
[12] Salima Lamsiyah et al., “Unsupervised Extractive Multi-Document Summarization Method Based on Transfer Learning from BERT Multi-Task Fine-Tuning,” Journal of Information Science, vol. 49, no. 1, pp. 164-182, 2023.
[CrossRef][Google Scholar][Publisher Link]
[13] Arun Kumar Yadav et al., “Extractive Text Summarization using Deep Learning Approach,” International Journal of Information Technology, vol. 14, no. 5, pp. 2407-2415, 2022.
[CrossRef][Google Scholar][Publisher Link]
[14] Asad Abdi et al., “A Hybrid Deep Learning Architecture for Opinion-Oriented Multi-Document Summarization Based on Multi-Feature Fusion,” Knowledge-Based Systems, vol. 213, 2021.
[CrossRef][Google Scholar][Publisher Link]
[15] Mahesh Gangathimmappa et al., “Deep Learning Enabled Cross‐Lingual Search with A Metaheuristic Web-Based Query Optimization Model for Multi‐Document Summarization,” Concurrency and Computation: Practice and Experience, vol. 35, no. 2, 2023.
[CrossRef][Google Scholar][Publisher Link]
[16] R. Shuba Shini, and V. D. Ambeth Kumar, “Recurrent Neural Network based Text Summarization Techniques by Word Sequence Generation,” In 2021 6th International Conference on Inventive Computation Technologies, pp. 1224-1229, 2021.
[CrossRef][Google Scholar][Publisher Link]
[17] M. Nafees Muneera, and P. Sriramya, “An Enhanced Optimized Abstractive Text Summarization Traditional Approach Employing Multi-Layered Attentional Stacked LSTM with the Attention RNN,” In: Computer Vision and Machine Intelligence Paradigms for SDGs, vol. 967, pp. 303-318, 2023.
[CrossRef][Google Scholar][Publisher Link]
[18] Shengli Song, Haitao Huang, and Tongxiao Ruan, “Abstractive Text Summarization using LSTM-CNN Based deep Learning,” Multimedia Tools and Applications, vol. 78, pp. 857-875, 2019.
[CrossRef][Google Scholar][Publisher Link]
[19] Zeyu Liang, Junping Du, and Chaoyang Li, “Abstractive Social Media Text Summarization using Selective Reinforced Seq2Seq Attention Model,” Neurocomputing, vol. 410, pp. 432-440, 2020.
[CrossRef][Google Scholar][Publisher Link]
[20] T. B. Lalitha, and P. S. Sreeja, “Potential Web Content Identification and Classification System using NLP and Machine Learning Techniques,” International Journal of Engineering Trends and Technology, vol. 71, no. 4, pp. 403-415, 2023.
[CrossRef][Google Scholar][Publisher Link]
[21] Min Yang et al., “Hierarchical Human-Like Deep Neural Networks for Abstractive Text Summarization,” IEEE Transactions on Neural Networks and Learning Systems, vol. 32, no. 6, pp. 2744-2757, 2020.
[CrossRef][Google Scholar][Publisher Link]
[22] Amar Jeet Rawat, Sunil Ghildiyal, and Anil Kumar Dixit, “Topic Modeling Techniques for Document Clustering and Analysis of Judicial Judgements,” International Journal of Engineering Trends and Technology, vol. 70, no. 11, pp. 163-169, 2022.
[CrossRef][Google Scholar][Publisher Link]
[23] Panagiotis Kouris, Georgios Alexandridis, and Andreas Stafylopatis, “Abstractive Text Summarization Based on Deep Learning and Semantic Content Generalization,” Proceedings of the 57th Annual Meeting of the Association for Computational Linguistics, pp. 5082–5092, 2022.
[Google Scholar][Publisher Link]
[24] Uthkarsha sagar, “A Broad Survey of Natural Language Processing,” SSRG International Journal of Computer Science and Engineering, vol. 6, no. 12, pp. 15-18, 2019.
[CrossRef][Publisher Link]
[25] Heewon Jang, and Wooju Kim, “Reinforced Abstractive Text Summarization with Semantic Added Reward,” IEEE Access, vol. 9, pp. 103804-103810, 2021.
[CrossRef][Google Scholar][Publisher Link]
[26] M. A. Bhalekar, and M. V. Bedekar, “Review on Latest Approaches used in Natural Language Processing for Generation of Image Captioning,” SSRG International Journal of Computer Science and Engineering, vol. 4, no. 6, pp. 41-48, 2017.
[CrossRef][Google Scholar][Publisher Link]
[27] Balaanand Muthu et al., “A Framework for Extractive Text Summarization Based on Deep Learning Modified Neural Network Classifier,” Transactions on Asian and Low-Resource Language Information Processing, vol. 20, no. 3, pp. 1-20, 2021.
[CrossRef][Google Scholar][Publisher Link]
[28] Asif Ansari, and N. M. Sreenarayanan, “Analysis of Text Classification of Dataset using NB-Classifier,” SSRG International Journal of Computer Science and Engineering, vol. 7, no. 6, pp. 24-28, 2020.
[CrossRef][Google Scholar][Publisher Link]
[29] Jin Liu et al., “Attention-Based BiGRU-CNN for Chinese Question Classification,” Journal of Ambient Intelligence and Humanized Computing, pp. 1-12, 2019.
[CrossRef][Google Scholar][Publisher Link]
[30] Sayali Hande, and M. A. Potey, “Temporal Text Summarization of TV Serial Excerpts using Lingo Clustering and Lucene Summarizer,” SSRG International Journal of Computer Science and Engineering, vol. 2, no. 5, pp. 16-22, 2015.
[CrossRef][Google Scholar][Publisher Link]
[31] Hamza Mohammed Ridha Al-Khafaji, “Data Collection in IoT using UAV Based on Multi-Objective Spotted Hyena Optimizer,” Sensors, vol. 22, no. 22, pp. 1-15, 2022.
[CrossRef][Google Scholar][Publisher Link]