A Hybrid Model using MobileNetv2 and SVM for Enhanced Classification and Prediction of Tomato Leaf Diseases
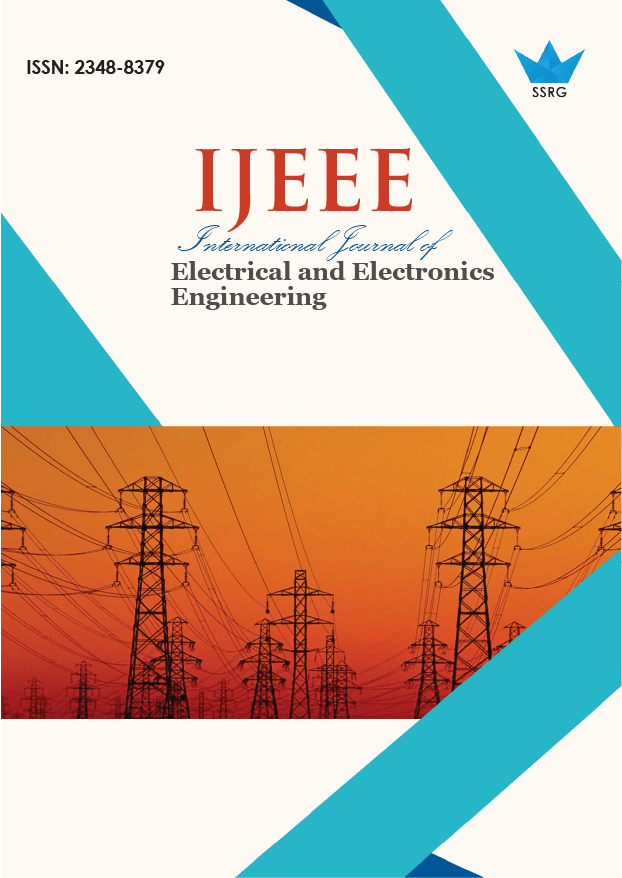
International Journal of Electrical and Electronics Engineering |
© 2023 by SSRG - IJEEE Journal |
Volume 10 Issue 8 |
Year of Publication : 2023 |
Authors : N. F. Esomonu, U. F. Eze, A. M. John-Otumu, I. I. Ayogu, O. C. Nwokonkwo, E. O. Oshoiribhor, S. A. Okolie, E. C. Nwokorie, C. V. Mbamala, O. Dokun |
How to Cite?
N. F. Esomonu, U. F. Eze, A. M. John-Otumu, I. I. Ayogu, O. C. Nwokonkwo, E. O. Oshoiribhor, S. A. Okolie, E. C. Nwokorie, C. V. Mbamala, O. Dokun, "A Hybrid Model using MobileNetv2 and SVM for Enhanced Classification and Prediction of Tomato Leaf Diseases," SSRG International Journal of Electrical and Electronics Engineering, vol. 10, no. 8, pp. 37-50, 2023. Crossref, https://doi.org/10.14445/23488379/IJEEE-V10I8P104
Abstract:
This study proposes an innovative method for automated categorising tomato leaf diseases using a hybrid model that combines deep learning and machine learning approaches. The proposed model integrates Convolutional Neural Networks (CNNs) pre-trained model (MobileNetv2) for feature mining and SVM for disease multi-class classification and prediction. A comprehensive dataset of 10,000 tomato leaf images was collected, preprocessed, and utilized for model training and evaluation. Experimental results demonstrate the efficacy of the hybrid model, achieving an impressive accuracy ranging from 88% to 99% in multi-class disease image classification. According to the comparative analysis, the relevant models' accuracies range from 70% to 97%. The outcome further endorses the proposed techniques' superiority. It highlights the necessity of choosing the appropriate model architecture and optimization strategies for obtaining high accuracy in image classification tasks, indicating its potential for real-world deployment. This study adds to the advancement of agronomic know-how by providing an efficient and accurate tool for tomato disease management, enabling early detection, and supporting sustainable crop production.
Keywords:
Deep learning, Pre-trained models, Machine learning, Classification, Prognosis, Tomato diseases.
References:
[1] Tiago Domingues, Tomás Brandão, and João C. Ferreira, “Machine Learning for Detection and Prediction of Crop Diseases and Pests: A Comprehensive Survey,” Agriculture, vol. 12, no. 9, pp. 1-23, 2022.
[CrossRef] [Google Scholar] [Publisher Link]
[2] Dániel Fróna, János Szenderák, and Mónika Harangi-Rákos, “The Challenge of Feeding the World,” Sustainability, vol. 11, no. 20, pp. 1-18, 2019.
[CrossRef] [Google Scholar] [Publisher Link]
[3] Iftikhar Ahmad et al., “Optimizing Pretrained Convolutional Neural Networks for Tomato Leaf Disease Detection,” Complexity, vol. 2020, pp. 1-6, 2020.
[CrossRef] [Google Scholar] [Publisher Link]
[4] Max Roser et al., “World Population Growth,” Our World in Data, 2013. [Online]. Available: http://www.sisterschiropractor.com/world-population-growth.html#citation
[5] Wimpie Nell et al., “A Creative Multidisciplinary Approach Towards the Development of Food Gardening,” Development Southern Africa, vol. 17, no. 5, pp. 807-819, 2010.
[CrossRef] [Google Scholar] [Publisher Link]
[6] Deepak K. Ray et al., “Yield Trends are Insufficient to Double Global Crop Production by 2050,” PLoS One, vol. 8, no. 6, p. 0066428, 2013.
[CrossRef] [Google Scholar] [Publisher Link]
[7] Mary Izuaka, Traders Report a Tomato Shortage as a Pest Reappears in Kano, Premium Times, 2020. [Online]. Available: https://www.premiumtimesng.com/agriculture/agric-news/394536-traders-report-tomato-shortage-as-pest-reappears-in-kano.html
[8] Food and Agriculture Organization of the United Nations, The Future of Food and Agriculture: Trends and Challenges, Rome, 2017. [Online]. Available: https://www.fao.org/3/i6583e/i6583e.pdf
[9] C. P. Adekunle, C. I. Alarima, and E. T. Tolorunju, “Differentials in Willingness to Pay for Biofortified Tomato Fruits in Abeokuta Metropolis, Ogun State, Nigeria,” Nigeria Agricultural Journal, vol. 50, no. 2, pp. 1-9, 2019.
[Google Scholar] [Publisher Link]
[10] Minse Modi, Albert Modi, and Sheryl Hendriks, “Potential Role for Wild Vegetables in Household Food Security,” African Journal of Food, Agriculture, Nutrition, and Development, vol. 6, no. 1, pp. 1–13, 2006.
[CrossRef] [Google Scholar] [Publisher Link]
[11] Debjit bhowmik et al., “Tomato: A Natural Medicine and Its Health Benefits,” Journal of Pharmacognosy and Phytochemistry, vol. 1, no. 1, pp. 33-43, 2012.
[Google Scholar] [Publisher Link]
[12] Wilfried Baudoin et al., Good Agricultural Practices for Greenhouse Vegetable Production in the Southeast European Countries, 2017. [Online]. Available: http://www.fao.org/3/a-i6787e.pdf
[13] Food and Agriculture Organization of the United Nations, Integrated Pest Management in Tomatoes in Eritrea In Sustainable Management of Arthropod Pests of Tomato, Rome, 2019. [Online]. Available: https://www.fao.org/3/ca6480en/CA6480EN.pdf
[14] Illaria Buja et al., “Advances in Plant Disease Detection and Monitoring: From Traditional Assays to In-Field Diagnostic,” Sensors, vol. 21, no. 6, pp. 1-22, 2021.
[CrossRef] [Google Scholar] [Publisher Link]
[15] A. M. John-Otumu, G. U. Ogba, and O. C. Nwokonkwo, “A Survey on Artificial Intelligence based Techniques for Diagnosis of Hepatitis Variants,” Journal of Advances in Science and Engineering, vol. 3, pp. 43-56, 2020.
[CrossRef] [Google Scholar] [Publisher Link]
[16] A. M. John-Otumu et al., “AI-based Techniques for Online Social Media Network Sentiment Analysis: A Methodical Review,” International Journal of Computer and Information Engineering, vol. 16, no. 12, pp. 550-560, 2022.
[Google Scholar] [Publisher Link]
[17] Boran Sekeroglu, and Ilker Ozsahin, “Detection of Covid-19 from Chest X-Ray Images using Convolutional Neural Networks,” SLAS Technology: Translating Life Sciences Innovation, vol. 25, no. 6, pp. 553-565, 2020.
[CrossRef] [Google Scholar] [Publisher Link]
[18] Y. Altunta, and F. Kocamaz, “Deep Feature Extraction for the Detection of Tomato Plant Diseases and Pests Based on Leaf Images,” Celal Bayar Üniversitesi Fen Bilimleri Dergisi, vol. 17, no. 2, pp. 145-152, 2021.
[CrossRef] [Google Scholar] [Publisher Link]
[19] Jun Liu Xuewei Wang, “Tomato Diseases and Pest Detection Based on an Improved Yolo V3 Convolutional Neural Network,” Frontiers in Plant Science, vol. 11, pp. 1-12, 2020.
[CrossRef] [Google Scholar] [Publisher Link]
[20] Vinutha B. Hiremath, and M. M. Sandarsh Gowda, “Disease Prediction of Tomato Leaf using CNN and Deep Learning Techniques,” International Research Journal of Modernization in Engineering, Technology, and Science, 2020.
[Google Scholar]
[21] John P. Damicone, and Lynn Brandenberger, “Common Diseases of Tomatoes: Part I. Diseases Caused by Fungi,” Oklahoma Cooperative Extension Service, pp. 1-6, 2016.
[Publisher Link]
[22] Abrham Debasu Mengistu, Seffi Gebeyehu Mengistu, and Dagnachew Melesew, “An Automatic Coffee Plant Diseases Identification using Hybrid Approaches of Image Processing and Decision Tree,” Indonesian Journal of Electrical Engineering and Computer Science, vol. 9, no. 3, pp. 806-811, 2018.
[CrossRef] [Google Scholar] [Publisher Link]
[23] M. P. Islam et al., “Performance Prediction of Tomato Leaf Disease by a Series of Parallel Convolutional Neural Networks,” Smart Agricultural Technology, vol. 2, p. 100054, 2022.
[CrossRef] [Google Scholar] [Publisher Link]
[24] Lilian Mkonyi et al., “Early Identification of Tuta Absoluta in Tomato Plants using Deep Learning,” Scientific African, vol. 10, p. e00590, 2020.
[CrossRef] [Google Scholar] [Publisher Link]
[25] M. M. Gunarathna, R. M. K. T. Rathnayaka, and W. M. W. Kandegama, “Identification of an Efficient Deep Leaning Architecture for Tomato Disease Classification using Leaf Images,” Journal of Food and Agriculture, vol. 13, no. 1, pp. 33-53, 2020.
[Google Scholar] [Publisher Link]
[26] Naresh K. Trivedi et al., “Early Detection and Classification of Tomato Leaf Disease using a High-Performance Deep Neural Network,” Sensors, vol. 21, no. 23, pp. 1-15, 2021.
[CrossRef] [Google Scholar] [Publisher Link]
[27] Shaheen Khatoon et al., “Image-based Automatic Diagnostic System for Tomato Plants using Deep Learning,” Computers, Materials, and Continua, vol. 67, no. 1, pp. 595-612, 2021.
[CrossRef] [Google Scholar] [Publisher Link]
[28] Rajath Ramakrishna, “A Machine Learning-Based Approach to the Detection and Classification of Tomato Plant Leaf Diseases,” NORMA eResearch @NCI Library, 2020.
[Publisher Link]
[29] P. Sreelatha et al., “Managing Tomato Leaf Disease Detection Accuracy using Computer Vision-Based Deep Neural Network,” Journal of Contemporary Issues in Business and Government, vol. 27, no. 1, pp. 3425–3437, 2021.
[Google Scholar] [Publisher Link]
[30] Mehdhar S. A. M. Al-gaashani et al., “Tomato Leaf Disease Classification by Exploiting Transfer Learning and Feature Concatenation,” IET Image Processing, vol. 16, no. 3, pp. 913-925, 2022.
[CrossRef] [Google Scholar] [Publisher Link]
[31] Radwan Qasrawi et al., “Machine Learning Techniques for Tomato Plant Disease Clustering, Prediction, and Classification,” In Proceedings of the 2021 International Conference on Promising Electronic Technologies, Palestine, pp. 40–45, 2021.
[CrossRef] [Google Scholar] [Publisher Link]
[32] Lerina Aversano et al., “Tomato Disease Classification based on VGG and Transfer Learning,” 2020 IEEE International Workshop on Metrology for Agriculture and Forestry, MetroAgriFor, Italy, pp. 129-133, 2020.
[CrossRef] [Google Scholar] [Publisher Link]
[33] H. S. Nagamani, and H. Sarojadevi, “Tomato Leaf Disease Detection using Deep Learning Techniques,” International Journal of Advanced Computer Science and Applications, vol. 13, no. 1, pp. 305-311, 2022.
[CrossRef] [Google Scholar] [Publisher Link]
[34] D. Sheema et al., “The Detection and Identification of Pest-FAW Infestation in Maize Crops using Iot-Based Deep-Learning Algorithm,” SSRG International Journal of Electrical and Electronics Engineering, vol. 9, no. 12, pp. 180-188, 2022.
[CrossRef] [Publisher Link]
[35] Victor Gonzalez-Huitron et al., “Disease Detection in Tomato Leaves via CNN with Lightweight Architectures Implemented in the Raspberry Pi4,” Computers and Electronics in Agriculture, vol. 181, p. 105951, 2021.
[CrossRef] [Google Scholar] [Publisher Link]
[36] G. Sumalatha, Jhansi Rani Singothu, and S. Krishna Rao, “Transfer Learning-based Plant Disease Detection,” International Journal for Innovative Engineering and Management Research, vol. 10, no. 3, pp. 469-477, 2021.
[Google Scholar] [Publisher Link]
[37] Nithish Kannan E et al., “Tomato Leaf Disease Detection using A Convolutional Neural Network with Data Augmentation,” Proceedings of the 5th International Conference on Communication and Electronics Systems, pp. 1125-1132, 2020.
[CrossRef] [Google Scholar] [Publisher Link]
[38] Vatsal Mahajan, Dilip Jain, and Abhinav Dua, “Plant Leaf Segmentation Invariant of Background,” International Journal of Computer & Organization Trends, vol. 4, no. 5, pp. 15-18, 2014.
[CrossRef] [Publisher Link]
[39] Huiqun Hong, Jinfa Lin, and Fenghua Huang, “Tomato Disease Detection and Classification by Deep Learning,” Proceedings of the 2020 International Conference on Big Data, Artificial Intelligence, and Internet of Things Engineering, China, pp. 25-29, 2020.
[CrossRef] [Google Scholar] [Publisher Link]
[40] Gargi Sharma, and Gourav Shrivastava, “Crop Disease Prediction using Deep Learning Techniques - A Review,” SSRG International Journal of Computer Science and Engineering, vol. 9, no. 4, pp. 23-28, 2022.
[CrossRef] [Publisher Link]
[41] R. Raja Kumar et al., “Novel Segmentation and Classification Algorithm for Detection of Tomato Leaf Disease,” Concurrency and Computation: Practice and Experience, vol. 335, no. 12, p. e7674, 2023.
[CrossRef] [Google Scholar] [Publisher Link]
[42] Mohit Agarwal et al., “ToLeD: Tomato Leaf Disease Detection using Convolutional Neural Network,” Procedia Computer Science, vol. 167, pp. 293-301, 2020.
[CrossRef] [Google Scholar] [Publisher Link]
[43] Garima Shrestha et al., “Plant Disease Detection using CNN,” Proceedings of the 2020 IEEE Applied Signal Processing Conference, pp. 109-113, 2020.
[CrossRef] [Google Scholar] [Publisher Link]
[44] M. Sardogan, A. Tuncer, and Y. Ozen, “Plant Leaf Disease Detection and Classification based on CNN with the LVQ Algorithm,” UBMK 2018: 3rd International Conference on Computer Science and Engineering, pp. 382-385, 2018.
[CrossRef] [Google Scholar] [Publisher Link]