Scale Invariant Deep Neural Multiple Feature Learning Based Boosted Support Vector Entropy Classification for Breast Cancer Diagnosis using Mammograms
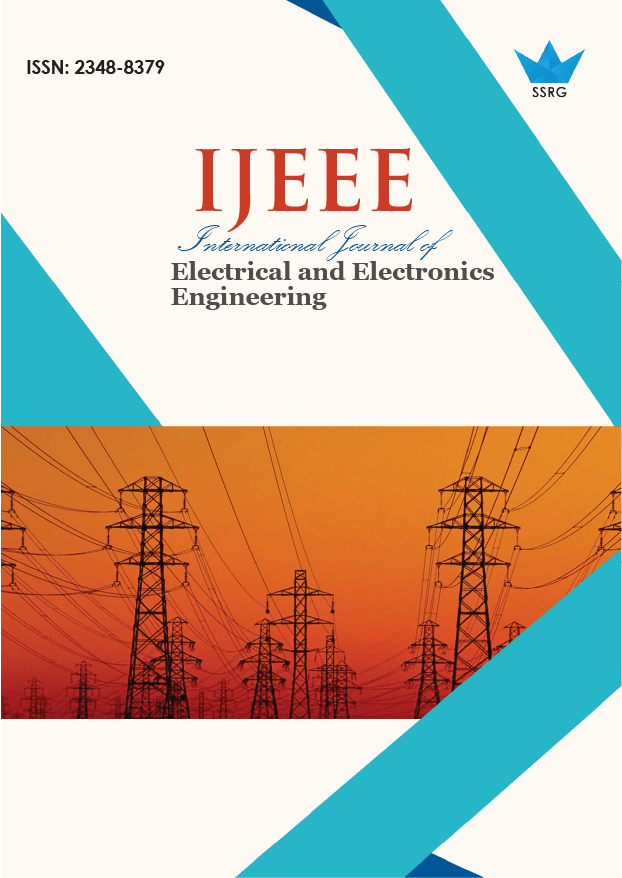
International Journal of Electrical and Electronics Engineering |
© 2023 by SSRG - IJEEE Journal |
Volume 10 Issue 8 |
Year of Publication : 2023 |
Authors : K. Sai Krishna, P. Grace Kanmani Prince |
How to Cite?
K. Sai Krishna, P. Grace Kanmani Prince, "Scale Invariant Deep Neural Multiple Feature Learning Based Boosted Support Vector Entropy Classification for Breast Cancer Diagnosis using Mammograms," SSRG International Journal of Electrical and Electronics Engineering, vol. 10, no. 8, pp. 79-88, 2023. Crossref, https://doi.org/10.14445/23488379/IJEEE-V10I8P108
Abstract:
An earlier diagnosis of cancer rises the living days of patients. The evolution of medical systems supports to identify the presence of cancer. In the state-of-the-art works, few methods were implemented for diagnosing breast cancer with different Deep Neural Network (DNN) support. However, the traditional DNN techniques were computationally expensive as it does provide better feature extraction performance with minimal time usage. Besides that, the misclassification error observed during the diagnosis process was higher, impacting the accuracy of early breast cancer prediction. Therefore, a novel Scale Invariant Deep Feature Learning Based Boosted Support Vector Entropy Classification (SIDFL-BSVEC) method is introduced. The SIDFL-BSVEC method is proposed to get better accuracy for the early identification of breast cancer with lesser time. The SIDFL-BSVEC method initially designs Scale Invariant Deep Neural Multiple Feature Extraction (SIDNMFE) algorithms to discover critical features in input mammograms with minimal time by working as robust to illumination variations, noise, partial occlusion, and minor viewpoint changes in mammograms images. These traits are significant for early cancer disease prediction when cells in the mammogram image have dissimilar sizes and orientations. After that, Support Vector Entropy Boosted Cancer Classifier (SVEBCC) algorithm is designed to minimize the error rate determined during the early analysis of breast cancer. The performance of the proposed CWDCMFE-MBRC technique is verified by taking the parameters such as disease diagnosis accuracy, disease diagnosis time, sensitivity, specificity and error rate along with various numbers of input images.
Keywords:
Scale invariant features transform, Deep learning, Boosting, Support vector entropy, Morphological features, Texture features, Density features.
References:
[1] Farnoosh Azour, and Azzedine Boukerche, “An Efficient Transfer and Ensemble Learning Based Computer Aided Breast Abnormality Diagnosis System,” IEEE Access, vol. 11, pp. 21199-21209, 2023.
[CrossRef] [Google Scholar] [Publisher Link]
[2] T. Kavitha et al., “Deep Learning Based Capsule Neural Network Model for Breast Cancer Diagnosis using Mammogram Images,” Interdisciplinary Sciences: Computational Life Sciences, vol. 14, pp. 113–129, 2022.
[CrossRef] [Google Scholar] [Publisher Link]
[3] Saliha Zahoor, Umar Shoaib, and Ikram Ullah Lali, “Breast Cancer Mammograms Classification using Deep Neural Network and Entropy-Controlled Whale Optimization Algorithm,” Diagnostics, vol. 12, no. 2, p. 557, 2022.
[CrossRef] [Google Scholar] [Publisher Link]
[4] Tariq Mahmood et al., “An Automated In-Depth Feature Learning Algorithm for Breast Abnormality Prognosis and Robust Characterization from Mammography Images using Deep Transfer Learning,” Biology, vol. 10, no. 9, p. 859, 2021.
[CrossRef] [Google Scholar] [Publisher Link]
[5] Asaf Raza et al., “Deep Breast Cancer Net: A Novel Deep Learning Model for Breast Cancer Detection using Ultrasound Images,” Applied Sciences, vol. 13, no. 4, 2023.
[CrossRef] [Google Scholar] [Publisher Link]
[6] Naveed Chouhan et al., “Deep Convolutional Neural Network and Emotional Learning Based Breast Cancer Detection using Digital Mammography,” Computers in Biology and Medicine, vol. 132, p. 104318, 2021.
[CrossRef] [Google Scholar] [Publisher Link]
[7] Manisha Bhende et al., “Deep Learning-Based Real-Time Discriminate Correlation Analysis for Breast Cancer Detection,” BioMed Research International, vol. 2022, pp. 1-12, 2022.
[CrossRef] [Google Scholar] [Publisher Link]
[8] Abdullah-Al Nahid, and Yinan Kong, “Involvement of Machine Learning for Breast Cancer Image Classification: A Survey,” Computational and Mathematical Methods in Medicine, vol. 2017, pp. 1-30, 2017.
[CrossRef] [Google Scholar] [Publisher Link]
[9] Nusrat Mohi ud din et al., “Breast Cancer Detection using Deep Learning: Datasets, Methods, and Challenges Ahead,” Computers in Biology and Medicine, vol. 149, p. 106073, 2022.
[CrossRef] [Google Scholar] [Publisher Link]
[10] Subasish Mohapatra et al., “Evaluation of Deep Learning Models for Detecting Breast Cancer using Histopathological Mammograms Images,” Sustainable Operations and Computers, vol. 3, pp. 296-302, 2022.
[CrossRef] [Google Scholar] [Publisher Link]
[11] K. Shwetha et al., “Breast Cancer Detection using Deep Learning Technique,” International Journal of Engineering Research & Technology (IJERT), vol. 6, no. 13, pp. 1-4, 2018.
[Google Scholar] [Publisher Link]
[12] Susmita Mishra, and M. Prakash, “Digital Mammogram Inferencing System using Intuitionistic Fuzzy Theory,” Computer Systems Science and Engineering, vol. 41, no. 3, pp. 1099-1115, 2022.
[CrossRef] [Google Scholar] [Publisher Link]
[13] Olaide N. Oyelade, and Absalom E. Ezugwu, “Characterization of Abnormalities in Breast Cancer Images using Nature-Inspired Metaheuristic Optimized Convolutional Neural Networks Model,” Concurrency and Computation Practice and Experience, vol. 34, no. 4, p. e6629, 2021.
[CrossRef] [Google Scholar] [Publisher Link]
[14] Han Jiao et al., “Deep Convolutional Neural Networks-Based Automatic Breast Segmentation and Mass Detection in DCE-MRI,” Computational and Mathematical Methods in Medicine, vol. 2020, pp. 1-12, 2020.
[CrossRef] [Google Scholar] [Publisher Link]
[15] Nonita Sharma et al, “Breast Cancer Classification using Snapshot Ensemble Deep Learning Model and T-Distributed Stochastic Neighbor Embedding,” Multimedia Tools and Applications, vol. 82, pp. 4011-4029, 2022.
[CrossRef] [Google Scholar] [Publisher Link]
[16] Linling Qiu et al., “Gated Graph Attention Network for Cancer Prediction,” Sensors, vol. 21, no. 6, pp. 1-21, 2021.
[CrossRef] [Google Scholar] [Publisher Link]
[17] G. Rajasekaran, and C. Sunitha Ram, “Effective Breast Cancer Prediction Based on Feature Extraction, Fusion and Selection using Hybrid Methodologies,” SSRG International Journal of Electrical and Electronics Engineering, vol. 10, no. 5, pp. 131-142, 2023.
[CrossRef] [Publisher Link]
[18] Nada M. Hassan, Safwat Hamad, and Khaled Mahar, “Mammogram Breast Cancer CAD Systems for Mass Detection and Classification: A Review,” Multimedia Tools and Applications, vol. 81, pp. 20043–20075, 2022.
[CrossRef] [Google Scholar] [Publisher Link]
[19] Mariam Shadan et al., “Histological Categorization of Stromal Desmoplasia in Breast Cancer and Its Diagnostic and Prognostic Utility,” SSRG International Journal of Medical Science, vol. 4, no. 6, pp. 8-11, 2017.
[CrossRef] [Google Scholar] [Publisher Link]
[20] A. Zulaiha Parveen, and T. Senthil Kumar, “The Deep Learning Methodology for Improved Breast Cancer Diagnosis in MRI,” International Journal of Computer and Organization Trends, vol. 11, no. 3, pp. 11-14, 2021.
[CrossRef] [Publisher Link]
[21] T. Rajendran et al., “A Deep Learning Based Methodological Analysis for Breast Cancer Classification,” SSRG International Journal of Electronics and Communication Engineering, vol. 10, no. 6, pp. 52-68, 2023.
[CrossRef] [Publisher Link]
[22] Farnoosh Azour, and Azzedine Boukerche, “Design Guidelines for Mammogram-Based Computer-Aided Systems using Deep Learning Techniques,” IEEE Access, vol. 10, pp. 21701-21726, 2022.
[CrossRef] [Google Scholar] [Publisher Link]
[23] Dong Li et al., “Convolutional Feature Descriptor Selection for Mammogram Classification,” IEEE Journal of Biomedical and Health Informatics, vol. 27, no. 3, pp. 1467-1476, 2023.
[CrossRef] [Google Scholar] [Publisher Link]