Machine Learning-Driven PCOS Prediction for Early Detection and Tailored Interventions
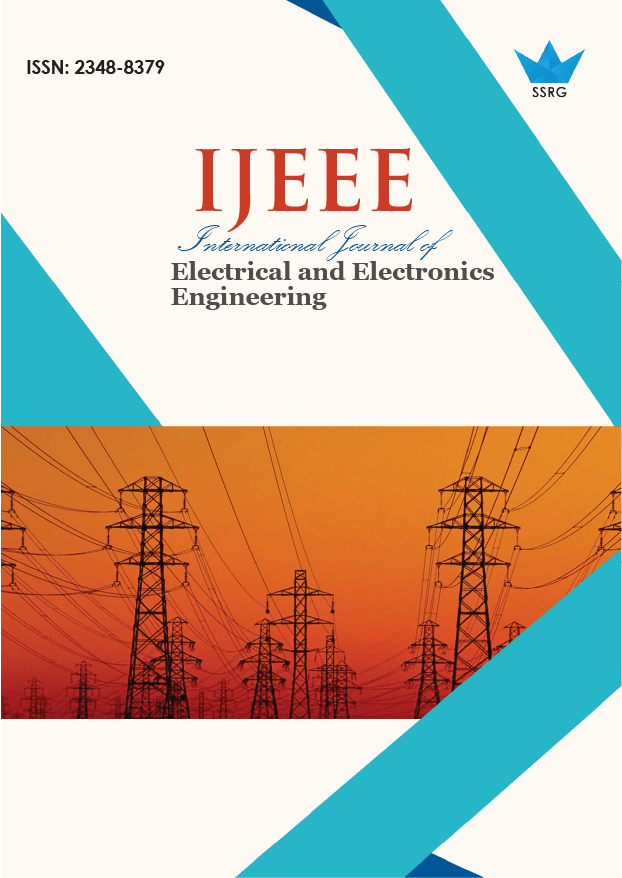
International Journal of Electrical and Electronics Engineering |
© 2023 by SSRG - IJEEE Journal |
Volume 10 Issue 9 |
Year of Publication : 2023 |
Authors : B.Yamini, Venkata Ramana Kaneti, Prema.P, Ambhika C, M.Nalini, Siva Subramanian.R |
How to Cite?
B.Yamini, Venkata Ramana Kaneti, Prema.P, Ambhika C, M.Nalini, Siva Subramanian.R, "Machine Learning-Driven PCOS Prediction for Early Detection and Tailored Interventions," SSRG International Journal of Electrical and Electronics Engineering, vol. 10, no. 9, pp. 61-75, 2023. Crossref, https://doi.org/10.14445/23488379/IJEEE-V10I9P106
Abstract:
This PCOS is a hormonal disorder that leads to the overproduction of androgens, resulting in symptoms such as interrupted periods, ovarian follicles, excess body hair, weight gain, and infertility. Hormonal imbalances and abnormal male hormone production characterize it. The precise aetiology of Polycystic Ovary Syndrome is unknown, but insulin resistance and genetic factors may play a role. Due to its prevalence and potential long-term health effects, understanding and predicting PCOS is most important in healthcare. The study of PCOS in women aids in identifying the condition earlier and protecting women from life-threatening medical complications. This research uses ML algorithms to develop a novel predictive modelling strategy to identify individuals at risk of developing PCOS. In the field of reproductive health, ML has the potential to revolutionize healthcare by enhancing detection and prediction. Multiple ML models, such as LR, RF, SVM, NB, K NN, and XGBoost, were used to predict PCOS. The examination uses a PCOS dataset containing clinical, hormonal, and biological information from women with and without PCOS issues. The acquired experimental results are projected using various validity metrics, including precision, recall, accuracy and F1-Score. The outcome indicates that Machine Learning models have promising predictive ability, and the random forest model has a 90% accuracy rate, which is higher than any other model. PCOS research is essential for encouraging early diagnosis, effective treatment, and improved reproductive health outcomes for those affected. Using Machine Learning algorithms, our proposed method provides a promising approach to PCOS prediction, enabling physicians to rapidly identify at-risk patients and perform tailored therapies. By treating PCOS early, healthcare practitioners may help women with this complicated endocrine illness avoid problems and enhance their quality of life.
Keywords:
Machine Learning, Polycystic Ovary Syndrome, Prediction, Random forest, Women healthcare.
References:
[1] S. Minooee et al., “Prediction of Age at Menopause in Women with Polycystic Ovary Syndrome,” Climacteric, vol. 21, no. 1, pp. 29- 34, 2018.
[CrossRef] [Google Scholar] [Publisher Link]
2] Angela Zigarelli, Ziyang Jia, and Hyunsun Lee, “Machine-Aided Self-Diagnostic Prediction Models for Polycystic Ovary Syndrome: Observational Study,” JMIR Formative Research, vol. 6, no. 3, pp. 1-23, 2022.
[CrossRef] [Google Scholar] [Publisher Link]
[3] Miaoxian Ou et al., “AMH is a Good Predictor of Metabolic Risk in Women with PCOS: A Cross-Sectional Study,” International Journal of Endocrinology, vol. 2021, pp. 1-7, 2021.
[CrossRef] [Google Scholar] [Publisher Link]
[4] Jacob P. Christ, and Tommaso Falcone, “Bariatric Surgery Improves Hyperandrogenism, Menstrual Irregularities, and Metabolic Dysfunction among Women with Polycystic Ovary Syndrome (PCOS),” Obesity Surgery, vol. 28, pp. 2171-2177, 2018.
[CrossRef] [Google Scholar] [Publisher Link]
[5] Amsy Denny et al., “i-HOPE: Detection and Prediction System for Polycystic Ovary Syndrome (PCOS) Using Machine Learning Techniques,” 2019 IEEE Region 10 Conference (TENCON), Kochi, India, pp. 673-678, 2019.
[CrossRef] [Google Scholar] [Publisher Link]
[6] Ashwini Kodipalli, and Susheela Devi, “Prediction of PCOS and Mental Health Using Fuzzy Inference and SVM,” Frontiers in Public Health, vol. 9, pp. 1-15, 2021.
[CrossRef] [Google Scholar] [Publisher Link]
[7] Preeti Chauhan et al., “Comparative Analysis of Machine Learning Algorithms for Prediction of PCOS,” 2021 International Conference on Communication Information and Computing Technology, Mumbai, India, pp. 1-7, 2021.
[CrossRef] [Google Scholar] [Publisher Link]
[8] Dipo Theophilus Akomolafe, Oluwatoyin Mary Yerokun, and Ayo Fasakin, “Resolving Some Critical Issues in the Prevention, Diagnosis, Treatment and Management of Covid-19 Using Machine Learning,” International Journal of Computer and Organization Trends, vol. 10, no. 4, pp. 1-8, 2020.
[CrossRef] [Google Scholar] [Publisher Link]
[9] Subrato Bharati, Prajoy Podder, and M. Rubaiyat Hossain Mondal, “Diagnosis of Polycystic Ovary Syndrome Using Machine Learning Algorithms,” 2020 IEEE Region 10 Symposium, Dhaka, Bangladesh, pp. 1486-1489, 2020.
[CrossRef] [Google Scholar] [Publisher Link]
[10] Sayma Alam Suha, and Muhammad Nazrul Islam, “An Extended Machine Learning Technique for Polycystic Ovary Syndrome Detection Using Ovary Ultrasound Image,” Scientific Reports, vol. 12, pp. 1-16, 2022.
[CrossRef] [Google Scholar] [Publisher Link]
[11] Subrato Bharati et al., “Ensemble Learning for Data-Driven Diagnosis of Polycystic Ovary Syndrome,” International Conference on Intelligent Systems Design and Applications, pp. 1250-1259, 2021.
[CrossRef] [Google Scholar] [Publisher Link]
[12] Muhammad Sakib Khan Inan et al., “Improved Sampling and Feature Selection to Support Extreme Gradient Boosting For PCOS Diagnosis,” 2021 IEEE 11th Annual Computing and Communication Workshop and Conference, USA, pp. 1046-1050, 2021.
[CrossRef] [Google Scholar] [Publisher Link]
[13] Satish C.R. Nandipati, Chew Xin Ying, and Khaw Khai Wah, “Polycystic Ovarian Syndrome (PCOS) Classification and Feature Selection by Machine Learning Techniques,” Applied Mathematics and Computational Intelligence, vol. 9, pp. 65- 74, 2020.
[Google Scholar] [Publisher Link]
[14] Harsita Batra et al., “Machine Learning Techniques for Data-Driven Computer-Aided Diagnostic Method of Polycystic Ovary Syndrome (PCOS) Resulting from Functional Ovarian Hyperandrogenism (FOH),” 2023 International Conference on Computational Intelligence and Sustainable Engineering Solutions (CISES), Greater Noida, India, pp. 195-201, 2023.
[CrossRef] [Google Scholar] [Publisher Link]
[15] B. Rachana et al., “Detection of Polycystic Ovarian Syndrome Using Follicle Recognition Technique,” Global Transitions Proceedings, vol. 2, no. 2, pp. 304-308, 2021.
[CrossRef] [Google Scholar] [Publisher Link]
[16] Kanish Shah et al., “A Comparative Analysis of Logistic Regression, Random Forest and KNN Models for the Text Classification,” Augmented Human Research, vol. 5, 2020.
[CrossRef] [Google Scholar] [Publisher Link]
[17] Derek A. Pisner, and David M. Schnyer, Support Vector Machine, Machine Learning, pp. 101-121, 2020.
[CrossRef] [Google Scholar] [Publisher Link]
[18] Smriti Gupta, Harsh Kumar Verma, and Divyansh Bhardwaj, “Classification of Diabetes Using Naive Bayes and Support Vector Machine as a Technique,” Operations Management and Systems Engineering, Singapore, pp. 365–376, 2021.
[CrossRef] [Google Scholar] [Publisher Link]
[19] M.N.A.H. Sha‟abani et al., “KNN and SVM Classification for EEG: A Review,” Proceedings of the 5th International Conference on Electrical, Control & Computer Engineering, Kuantan, Pahang, Malaysia, pp. 555-565, 2020.
[Google Scholar] [Publisher Link]
[20] Nayan Kumar Sinha et al., “Developing a Web-Based System for Breast Cancer Prediction Using Xgboost Classifier,” International Journal of Engineering Research and Technology, vol. 9, no. 6, pp. 852-856, 2020.
[Google Scholar] [Publisher Link]
[21] Zulaiha Parveen A., and T. Senthil Kumar, “The Deep Learning Methodology for Improved Breast Cancer Diagnosis in MRI,” International Journal of Computer and Organization Trends, vol. 11, no. 3, pp. 11-14, 2021.
[CrossRef] [Publisher Link]
[22] D. Prabha et al., “Performance Evaluation of Naive Bayes Classifier with and without Filter-Based Feature Selection,” International Journal of Innovative Technology and Exploring Engineering, vol. 8, no. 10, pp. 2154-2158, 2019.
[Google Scholar] [Publisher Link]
[23] R. Gurumoorthy, and M. Kamarasan, “Wildebeest Habit Optimizer with Deep Learning-Based Histopathological Image Analysis for Breast Cancer Diagnosis,” International Journal of Engineering Trends and Technology, vol. 71, no. 7, pp. 233-243, 2023.
[CrossRef] [Publisher Link]
[24] Mohammed S. Atoum et al., “A Fog-Enabled Framework for Ensemble Machine Learning-Based Real-Time Heart Patient Diagnosis,” International Journal of Engineering Trends and Technology, vol. 71, no. 8, pp. 39-47, 2023.
[CrossRef] [Google Scholar] [Publisher Link]
[25] S. Praveena Rachel Kamala et al., “Predictive Analytics for Heart Disease Detection: A Machine Learning Approach,” 4 th International Conference on Electronics and Sustainable Communication Systems, Coimbatore, India, pp. 1583-1589, 2023.
[CrossRef] [Google Scholar] [Publisher Link]
[26] Varada Vivek Khanna et al., “A Distinctive Explainable Machine Learning Framework for the Detection of Polycystic Ovary Syndrome,” Applied System Innovation, vol. 6, no. 2, pp. 1-26, 2023.
[CrossRef] [Google Scholar] [Publisher Link]