Integration of Timbrel, Cepstral Domain and Linear Prediction-Based Features for Replay Attack Detection
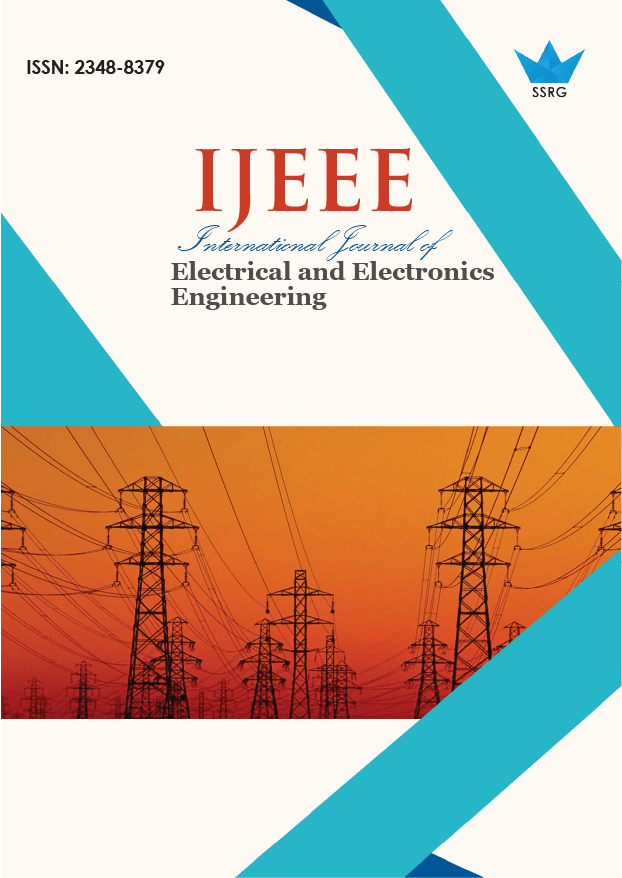
International Journal of Electrical and Electronics Engineering |
© 2023 by SSRG - IJEEE Journal |
Volume 10 Issue 10 |
Year of Publication : 2023 |
Authors : Amol A. Chaudhari, Dnyandeo K. Shedge, Vinayak K. Bairagi |
How to Cite?
Amol A. Chaudhari, Dnyandeo K. Shedge, Vinayak K. Bairagi, "Integration of Timbrel, Cepstral Domain and Linear Prediction-Based Features for Replay Attack Detection," SSRG International Journal of Electrical and Electronics Engineering, vol. 10, no. 10, pp. 108-125, 2023. Crossref, https://doi.org/10.14445/23488379/IJEEE-V10I10P112
Abstract:
The automatic speaker verification system is vulnerable to several spoofing attacks. Among these spoofing attacks, detecting replay attacks is challenging as attackers do not need any expertise to mount replay attacks. Many efforts from the research community have focused on anti-spoofing solutions against the reply attack. Such efforts are classified as one focusing on feature extraction and others concentrating on classifiers. This work evaluates the performance of feature extraction schemes CQCC, LFCC, and MFCC. The success of Linear Prediction analysis has been demonstrated in the past. This work evaluates the performance of LPC and LPCC features. The recent work in the literature has focused on using multiple features and combining these features for improved performance. In this work, numerous components of CQCC, MFCC, LFCC, LPC and LPCC are integrated considering various combinations and evaluated. In literature, the success of Timbrel features has been demonstrated for speaker identification. The feature vector formed using various Timbrel features is integrated with cepstral and linear prediction-based features. Finally, Timbrel features zero cross rate are combined with these multiple features. Among all experiments carried out on the ASVspoof 2017 version 2 database, EER 5.44% is achieved for the integration of zero cross rate and LPC on the development set and 17.79% EER is conducted for the integration of zero cross rate, MFCC, CQCC, LFCC, and LPCC features on evaluation set.
Keywords:
Automatic Speaker Verification, Replay attack, Timbrel features, T-SNE, Zero cross rate.
References:
[1] Madhu R. Kamble et al., “Advances in Anti-Spoofing: From the Perspective of ASVspoof Challenges,” APSIPA Transactions on Signal and Information Processing, vol. 9, pp. 1-18, 2020.
[CrossRef] [Google Scholar] [Publisher Link]
[2] A.K. Jain, A. Ross, and S. Pankanti, “Biometrics: A Tool for Information Security,” IEEE Transactions on Information Forensics and Security, vol. 1, no. 2, pp. 125-143, 2006.
[CrossRef] [Google Scholar] [Publisher Link]
[3] Anamika Baradiya, and Vinay Jain, “Speech and Speaker Recognition Technology Using MFCC and SVM,” SSRG International Journal of Electronics and Communication Engineering, vol. 2, no. 5, pp. 6-9, 2015.
[CrossRef] [Google Scholar] [Publisher Link]
[4] Priyanka Gupta, Piyushkumar K. Chodingala, and Hemant A. Patil, “Replay Spoof Detection Using Energy Separation Based Instantaneous Frequency Estimation from Quadrature and In-Phase Components,” Computer Speech & Language, vol. 77, 2023.
[CrossRef] [Google Scholar] [Publisher Link]
[5] Nicholas Evans, Tomi Kinnunen, and Junichi Yamagishi, “Spoofing and Countermeasures for Automatic Speaker Verification,” Proceedings of the Annual Conference of the International Speech Communication Association, pp. 925-929, 2013.
[CrossRef] [Google Scholar] [Publisher Link]
[6] Zhizheng Wu et al., “ASVspoof 2015: Automatic Speaker Verification Spoofing and Countermeasures Challenge Evaluation Plan,” Training, pp. 1-5, 2014.
[Google Scholar]
[7] Tomi Kinnunen et al., “ASVspoof 2017: Automatic Speaker Verification Spoofing and Countermeasures Challenge Evaluation Plan*,” Taining, pp. 1-6, 2018.
[Google Scholar]
[8] Andreas Nautsch et al., “ASVspoof 2019: Spoofing Countermeasures for the Detection of Synthesized, Converted and Replayed Speech,” IEEE Transactions on Biometrics, Behavior, and Identity Science, vol. 3, no. 2, pp. 252-265, 2021.
[CrossRef] [Google Scholar] [Publisher Link]
[9] Héctor Delgado et al., “ASVspoof 2021: Automatic Speaker Verification Spoofing and Countermeasures Challenge Evaluation Plan,” Electrical Engineering and Systems Science, 2021.
[CrossRef] [Google Scholar] [Publisher Link]
[10] Madhu R. Kamble, and Hemant A. Patil, “Detection of Replay Spoof Speech Using Teager Energy Feature Cues,” Computer Speech & Language, vol. 65, 2021.
[CrossRef] [Google Scholar] [Publisher Link]
[11] Roberto Font, Juan M. Espín, and María José Cano, “Experimental Analysis of Features for Replay Attack Detection-Results on the ASVspoof 2017 Challenge,” Proceedings of the Annual Conference of the International Speech Communication Association, pp. 7-11, 2017.
[CrossRef] [Google Scholar] [Publisher Link]
[12] Hemant A. Patil et al., “Novel Variable Length Teager Energy Separation Based Instantaneous Frequency Features for Replay Detection,” Proceedings of the Annual Conference of the International Speech Communication Association, pp. 12-16, 2017.
[CrossRef] [Google Scholar] [Publisher Link]
[13] Sarfaraz Jelil et al., “Spoof Detection Using Source, Instantaneous Frequency and Cepstral Features,” Proceedings of the Annual Conference of the International Speech Communication Association, pp. 22-26, 2017.
[CrossRef] [Google Scholar] [Publisher Link]
[14] Marcin Witkowski et al., “Audio Replay Attack Detection Using High-Frequency Features,” Proceedings of the Annual Conference of the International Speech Communication Association, pp. 27-31, 2017.
[CrossRef] [Google Scholar] [Publisher Link]
[15] Choon Beng Tan et al., “A Survey on Presentation Attack Detection for Automatic Speaker Verification Systems: State-of-the-Art, Taxonomy, Issues and Future Direction,” Multimedia Tools and Applications, vol. 80, pp. 32725-32762, 2021.
[CrossRef] [Google Scholar] [Publisher Link]
[16] Xiaojiang Pen et al., “Bag of Visual Words and Fusion Methods for Action Recognition: Comprehensive Study and Good Practice,” Computer Vision and Image Understanding, vol. 150, pp. 109-125, 2016.
[CrossRef] [Google Scholar] [Publisher Link]
[17] Kamer Vishi, and Vasileios Mavroeidis, “An Evaluation of Score Level Fusion Approaches for Fingerprint and Finger-Vein Biometrics,” Computer Science, pp. 1-10, 2018.
[CrossRef] [Google Scholar] [Publisher Link]
[18] Quan-Sen Sun et al., “A New Method of Feature Fusion and Its Application in Image Recognition,” Pattern Recognition, vol. 38, no. 12, pp. 2437-2448, 2005.
[CrossRef] [Google Scholar] [Publisher Link]
[19] Cemal Hanilci, “Speaker Verification Anti-Spoofing Using Linear Prediction Residual Phase Features,” 2017 25th European Signal Processing Conference (EUSIPCO), Greece, pp. 96-100, 2017.
[CrossRef] [Google Scholar] [Publisher Link]
[20] Madhu R. Kamble, and Hemant A. Patil, “Novel Energy Separation Based Frequency Modulation Features for Spoofed Speech Classification,” 2017 Ninth International Conference on Advances in Pattern Recognition (ICAPR), pp. 1-6, 2017.
[CrossRef] [Google Scholar] [Publisher Link]
[21] Jichen Yang, Rohan Kumar Das, and Haizhou Li, “Extended Constant-Q Cepstral Coefficients for Detection of Spoofing Attacks,” 2018 Asia-Pacific Signal and Information Processing Association Annual Summit and Conference (APSIPA ASC), USA, pp. 1024-1029, 2018.
[CrossRef] [Google Scholar] [Publisher Link]
[22] Krishna Dutta, Madhusudan Singh, and Debadatta Pati, “Detection of Replay Signals Using Excitation Source and Shifted CQCC Features,” International Journal of Speech Technology, vol. 24, pp. 497-507, 2021.
[CrossRef] [Google Scholar] [Publisher Link]
[23] Leian Liu, and Jichen Yang, “Study on Feature Complementarity of Statistics, Energy, and Principal Information for Spoofing Detection,” IEEE Access, vol. 8, pp. 141170-141181, 2020.
[CrossRef] [Google Scholar] [Publisher Link]
[24] Madhu R. Kamble, Hemlata Tak, and Hemant A. Patil, “Amplitude and Frequency Modulation-Based Features for Detection of Replay Spoof Speech,” Speech Communication, vol. 125, pp. 114-127, 2020.
[CrossRef] [Google Scholar] [Publisher Link]
[25] B.T. Balamurali et al., “Toward Robust Audio Spoofing Detection: A Detailed Comparison of Traditional and Learned Features,” IEEE Access, vol. 7, pp. 84229-84241, 2019.
[CrossRef] [Google Scholar] [Publisher Link]
[26] Khomdet Phapatanaburi et al., “Replay Attack Detection Using Linear Prediction Analysis-Based Relative Phase Features,” IEEE Access, vol. 7, pp. 183614-183625, 2019.
[CrossRef] [Google Scholar] [Publisher Link]
[27] Madhusudan Singh, and Debadatta Pati, “Usefulness of Linear Prediction Residual for Replay Attack Detection,” AEU - International Journal of Electronics and Communications, vol. 110, 2019.
[CrossRef] [Google Scholar] [Publisher Link]
[28] Madhusudan Singh, and Debadatta Pati, “Combining Evidences from Hilbert Envelope and Residual Phase for Detecting Replay Attacks,” International Journal of Speech Technology, vol. 22, pp. 313-326, 2019.
[CrossRef] [Google Scholar] [Publisher Link]
[29] Zeyan Oo et al., “Replay Attack Detection with Auditory Filter-Based Relative Phase Features,” EURASIP Journal on Audio, Speech, and Music Processing, vol. 2019, pp. 1-11, 2019.
[CrossRef] [Google Scholar] [Publisher Link]
[30] Sang Kyoon Park et al., “Zero-Crossing-Based Feature Extraction for Voice Command Systems Using Neck-Microphones,” International Symposium on Neural Networks in Advances in Neural Networks - ISNN 2007, pp. 1318-1326, 2007.
[CrossRef] [Google Scholar] [Publisher Link]
[31] V.M. Sardar, and S.D. Shirbahadurkar, “Speaker Identification of Whispering Speech: An Investigation on Selected Timbrel Features and KNN Distance Measures,” International Journal of Speech Technology, vol. 21, pp. 545-553, 2018.
[CrossRef] [Google Scholar] [Publisher Link]
[32] Vijay M. Sardar, and S.D. Shirbahadurkar, “Timbre Features for Speaker Identification of Whispering Speech: Selection of Optimal Audio Descriptors,” International Journal of Computers and Applications, vol. 43, no. 10, pp. 1047-1053, 2021.
[CrossRef] [Google Scholar] [Publisher Link]
[33] Vijay M. Sardar, Manisha L. Jadhav, and Saurabh H. Deshmukh, “Use of Median Timbre Features for Speaker Identification of Whispering Sound,” Techno-Societal 2020, pp. 31-41, 2021.
[CrossRef] [Google Scholar] [Publisher Link]
[34] Héctor Delgado et al., “ASVspoof 2017 Version 2.0: Meta-Data Analysis and Baseline Enhancements,” Odyssey 2018 - The Speaker and Language Recognition Workshop, Les Sables d’Olonne, France, 2018.
[Google Scholar] [Publisher Link]
[35] Tomi Kinnunen et al., “The ASVspoof 2017 Challenge: Assessing the Limits of Replay Spoofing Attack Detection,” Proceedings of the Annual Conference of the International Speech Communication Association, pp. 2-6, 2017.
[CrossRef] [Google Scholar] [Publisher Link]
[36] Massimiliano Todisco, Héctor Delgado, and Nicholas Evans, “Constant Q Cepstral Coefficients: A Spoofing Counter Measure for Automatic Speaker Verification,” Computer Speech & Language, vol. 45, pp. 516-535, 2017.
[CrossRef] [Google Scholar] [Publisher Link]
[37] Prashanth Kannadaguli, and Vidya Bhat, “Phoneme Modeling for Speech Recognition in Kannada Using Multivariate Bayesian Classifier,” SSRG International Journal of Electronics and Communication Engineering, vol. 1, no. 9, pp. 1-4, 2014.
[CrossRef] [Google Scholar] [Publisher Link]
[38] Sabur Ajibola Alim, and Nahrul Khair Alang Rashid, Some Commonly Used Speech Feature Extraction Algorithms, Natural to Artificial Intelligence - Algorithms and Applications, IntechOpen, 2018.
[CrossRef] [Google Scholar] [Publisher Link]
[39] K.M. Ravikumar, H.C. Nagaraj, and R. Rajagopal, “An Approach for Objective Assessment of Stuttered Speech Using MFCC Features,” The International Congress for Global Science and Technology, vol. 19, 2009.
[Google Scholar] [Publisher Link]
[40] Linqiang Wei et al., “New Acoustic Features for Synthetic and Replay Spoofing Attack Detection,” Symmetry, vol. 14, no. 2, pp. 1-17, 2022.
[CrossRef] [Google Scholar] [Publisher Link]
[41] Tae Hong Park, Towards Automatic Musical Instrument Timbre Recognition [Microform], ProQuest Dissertations and Thesis, Princeton University, 2004.
[42] Anthony Larcher, Sylvain Meignier, and Kong Aik Lee, SIDEKIT Documentation, 2020. [Online]. Available: https://projets-lium.univ-lemans.fr/sidekit/_downloads/544a50fdcc0129b614b6f4b90f1c89d0/sidekit.pdf
[43] Paul Boersma, and David Weenink, Praat: Doing Phonetics by Computer. [Online]. Available: http://www.praat.org
[44] K. Raja, “Detection and Prevention of Ransomware Attacks using AES and RSA Algorithms,” DS Jornal of Digital Science and Technology, vol. 1, no. 1, pp. 1-9, 2022.
[CrossRef] [Publisher Link]
[45] Mike Brookes, VOICEBOX: Speech Processing Tool Box for MATLAB. [Online]. Available: http://www.ee.ic.ac.uk/hp/staff/dmb/voicebox/voicebox.html
[46] Olivier Lartillot, Petri Toiviainen, and Tuomas Eerola, “A Matlab Toolbox for Music Information Retrieval,” Data Analysis, Machine Learning and Applications Conference, pp. 261-268, 2008.
[CrossRef] [Google Scholar] [Publisher Link]