The Impact of Nature-Inspired Optimization Techniques on Peak and Electricity Cost in Distribution Systems
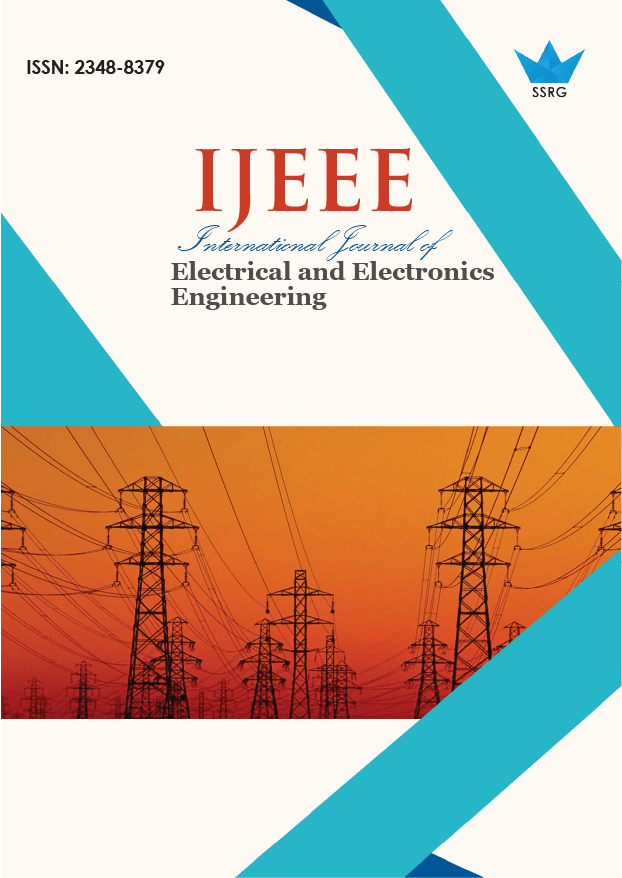
International Journal of Electrical and Electronics Engineering |
© 2023 by SSRG - IJEEE Journal |
Volume 10 Issue 10 |
Year of Publication : 2023 |
Authors : P. Kanakaraj, L. Ramesh, R. Narayanamoorthi |
How to Cite?
P. Kanakaraj, L. Ramesh, R. Narayanamoorthi, "The Impact of Nature-Inspired Optimization Techniques on Peak and Electricity Cost in Distribution Systems," SSRG International Journal of Electrical and Electronics Engineering, vol. 10, no. 10, pp. 165-175, 2023. Crossref, https://doi.org/10.14445/23488379/IJEEE-V10I10P116
Abstract:
In recent years, smart household appliances have led to an increase in residential electricity usage. Peak loads are created by these appliances in residential distribution systems. At peak times, residential distribution power consumption exceeds grid power. The imbalance in power demand results in low voltage in the distribution system, affecting household appliances. Increasing or decreasing grid power demand is necessary to protect these household appliances. The authors implemented renewable energy sources to address this issue, increasing grid power and demand-side management techniques to reduce energy consumption. Despite various research on optimal peak and cost reduction, a lack of different nature-inspired optimization techniques has been evident. The present paper proposes a demand-side load-shifting algorithm for peak load control in a residential building. This multi-objective load-shifting algorithm employs nature-inspired optimization techniques, including MBO, CSO, and AFSO, to reduce the utility’s peak load and the consumer’s electricity cost simultaneously. A comparison is made between the above-mentioned nature-inspired optimization approaches in this paper. Finally, the MBO’s results are superior to other nature-inspired optimization methods.
Keywords:
Peak Load Management (PLM), Demand Side Management (DSM), Load Shifting Algorithm (LSA), Monarch Butterfly Optimization (MBO), Crow Search Optimization (CSO), Artificial Fish Swarm Optimization (AFSO).
References:
[1] R. Snurr, and D. Freude, “Energy Fundamentals”. [Online]. Available: https://home.uni-leipzig.de/energy/energy-fundamentals/
[2] S. Yilmaz, A. Rinaldi, and M.K. Patel, “DSM Interactions: What is the Impact of Appliance Energy Efficiency Measures on the Demand Response (Peak Load Management)?,” Energy Policy, vol. 139, 2020.
[CrossRef] [Google Scholar] [Publisher Link]
[3] Olawale Popoola, and MoreBlessing Chipango, “Improved Peak Load Management Control Technique for Nonlinear and Dynamic Residential Energy Consumption Pattern,” Building Simulation, vol. 14, no. 1, pp. 195–208, 2021.
[CrossRef] [Google Scholar] [Publisher Link]
[4] Nihit Goyal, “Limited Demand or Unreliable Supply? A Bibliometric Review and Computational Text Analysis of Research on Energy Policy in India,” Sustainability, vol. 13, no. 23, pp. 1-23, 2021.
[CrossRef] [Google Scholar] [Publisher Link]
[5] B. Canizes et al., “Demand Response Driven by Distribution Network Voltage Limit Violation: A Genetic Algorithm Approach for Load Shifting,” IEEE Access, vol. 10, pp. 62183–62193, 2022.
[CrossRef] [Google Scholar] [Publisher Link]
[6] Alwyn Mathew, Abhijit Roy, and Jimson Mathew, “Intelligent Residential Energy Management System Using Deep Reinforcement Learning,” IEEE Systems Journal, vol. 14, no. 4, pp. 5362–5372, 2020.
[CrossRef] [Google Scholar] [Publisher Link]
[7] Milad Afzalan, and Farrokh Jazizadeh, “Residential Loads Flexibility Potential for Demand Response Using Energy Consumption Patterns and User Segments,” Applied Energy, vol. 254, 2019.
[CrossRef] [Google Scholar] [Publisher Link]
[8] Swati Sharda, Mukhtiar Singh, and Kapil Sharma, “Demand Side Management through Load Shifting in IoT Based HEMS: Overview, Challenges and Opportunities,” Sustainable Cities and Society, vol. 65, 2021.
[CrossRef] [Google Scholar] [Publisher Link]
[9] Subhasis Panda et al., “Residential Demand Side Management Model, Optimization and Future Perspective: A Review,” Energy Reports, vol. 8, pp. 3727–3766, 2022.
[CrossRef] [Google Scholar] [Publisher Link]
[10] Hamed Shakouri G., and Aliyeh Kazemi, “Multi-Objective Cost-Load Optimization for Demand Side Management of a Residential Area in Smart Grids,” Sustainable Cities and Society, vol. 32, pp. 171–180, 2017.
[CrossRef] [Google Scholar] [Publisher Link]
[11] Gai-Ge Wang, Suash Deb, and Zhihua Cui, “Monarch Butterfly Optimization,” Neural Computing and Applications, vol. 31, no. 7, pp. 1995–2014, 2019.
[CrossRef] [Google Scholar] [Publisher Link]
[12] Sukhwinder Singh Dhillon et al., “Monarch Butterfly Optimized Control with Robustness Analysis for Grid Tied Centralized and Distributed Power Generations,” Journal of Ambient Intelligence and Humanized Computing, vol. 13, no. 7, pp. 3595–3608, 2022.
[CrossRef] [Google Scholar] [Publisher Link]
[13] Pushpendra Singh et al., “Multi-Criteria Decision Making Monarch Butterfly Optimization for Optimal Distributed Energy Resources Mix in Distribution Networks,” Applied Energy, vol. 278, 2020.
[CrossRef] [Google Scholar] [Publisher Link]
[14] Vivek Yadava, and Sakti Prasad Ghoshal, “Optimal Power Flow for IEEE 30 and 118-Bus Systems Using Monarch Butterfly Optimization,” Technologies for Smart-City Energy Security and Power (ICSESP), vol. 2018, no. 3, pp. 1–6, 2018.
[Google Scholar] [Publisher Link]
[15] Alireza Askarzadeh, “A Novel Metaheuristic Method for Solving Constrained Engineering Optimization Problems: Crow Search Algorithm,” Computers & Structures, vol. 169, pp. 1–12, 2016.
[CrossRef] [Google Scholar] [Publisher Link]
[16] Oscar Danilo Montoya, Carlos Alberto Ramírez-Vanegas, and Luis Fernando Grisales-Noreña, “Parametric Estimation in Photovoltaic Modules Using the Crow Search Algorithm,” International Journal of Electrical and Computer Engineering (IJECE), vol. 12, no. 1, pp. 82–91, 2022.
[CrossRef] [Google Scholar] [Publisher Link]
[17] Surender Reddy Salkuti, “Solving Reactive Power Scheduling Problem Using Multi-objective Crow Search Algorithm,” International Journal of Advanced Computer Science and Applications (IJACSA), vol. 12, no. 6, pp. 42–48, 2021.
[CrossRef] [Google Scholar] [Publisher Link]
[18] T. Johnson, and T. Moger, “Security-Constrained Optimal Placement of PMUs Using Crow Search Algorithm,” Applied Soft Computing., vol. 128, 2022.
[CrossRef] [Google Scholar] [Publisher Link]
[19] Murilo E.C. Bento, “Computing the Load Margin of Power Systems Using Crow Search Algorithm,” IFAC-PapersOnLine, vol. 55, no. 1, pp. 271–275, 2022.
[CrossRef] [Google Scholar] [Publisher Link]
[20] Surender Reddy Salkuti, “Artificial Fish Swarm Optimization Algorithm for Power System State Estimation,” The Indonesian Journal of Electrical Engineering and Computer Science (IJEECS), vol. 18, no. 3, pp. 1130–1137, 2020.
[CrossRef] [Google Scholar] [Publisher Link]
[21] C.H. Hariprasad, R. Kayalvizhi, and N. Karthik, “Optimum Restructuring of Radial Distribution Network with Integration of DG and DSTATCOM Using Artificial Fish Swarm Optimization Technique,” IEEE 2nd International Conference on Sustainable Energy and Future Electric Transportation (SeFeT), pp. 4–6, 2022.
[CrossRef] [Google Scholar] [Publisher Link]
[22] K. Prakash Kumar, B. Saravanan, and K.S. Swarup, “Day Ahead Scheduling of Generation and Storage Sources in a Microgrid Using Artificial Fish Swarm Algorithm,” 21st Century Energy Needs - Materials, Systems and Applications (ICTFCEN), pp. 1–6, 2017.
[CrossRef] [Google Scholar] [Publisher Link]