Power Transformer Classification through Dissolved Gas Analysis Utilizing Least-Square Support Vector Machine
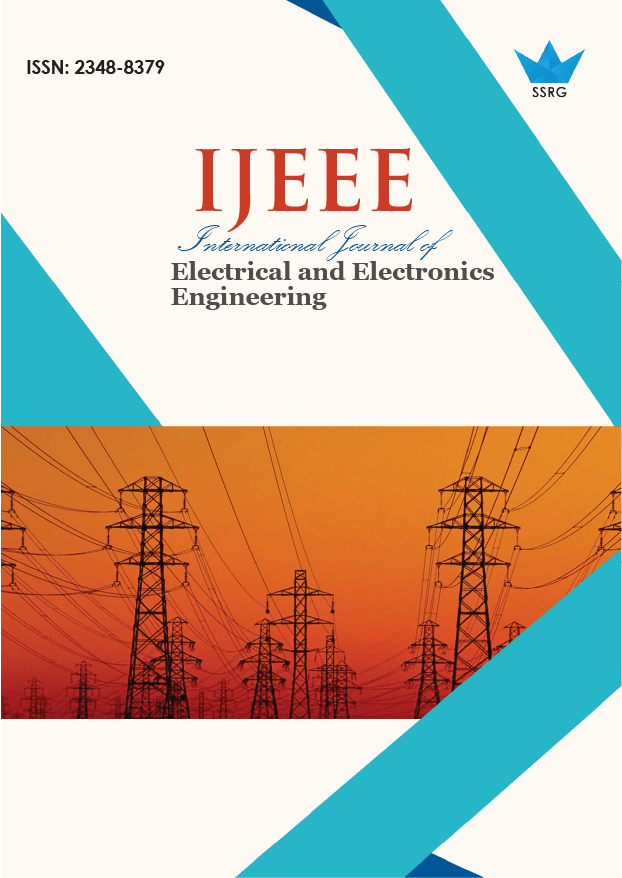
International Journal of Electrical and Electronics Engineering |
© 2024 by SSRG - IJEEE Journal |
Volume 11 Issue 2 |
Year of Publication : 2024 |
Authors : Zuhaila Mat Yasin, Fathiah Zakaria, Nur Ashida Salim, Nur Fadilah Ab Aziz |
How to Cite?
Zuhaila Mat Yasin, Fathiah Zakaria, Nur Ashida Salim, Nur Fadilah Ab Aziz, "Power Transformer Classification through Dissolved Gas Analysis Utilizing Least-Square Support Vector Machine," SSRG International Journal of Electrical and Electronics Engineering, vol. 11, no. 2, pp. 58-64, 2024. Crossref, https://doi.org/10.14445/23488379/IJEEE-V11I2P107
Abstract:
This article proposes the utilization of the Least-Square Support Vector Machine (LS-SVM) approach to ascertain the presence of a fault in power transformers. Power transformers are essential elements of electrical power systems. The failure of a power transformer can cause a disturbance in the functioning of power distribution and transmission systems. This situation will result in an increase in operating expenses due to the need for repairs and maintenance. The reliability of the electrical grid may be compromised. Therefore, it is crucial to identify any flaws in the power transformer at an early stage. In this paper, the LS-SVM utilizes Dissolved Gas Analysis (DGA) data as its input. The DGA methodology is widely accepted as the prevailing method for identifying the early stages of defects that arise in power transformers by analyzing the ratio of essential gases. The simulation data acquired from the industry comprises a standard state and six distinct fault types of transformers, which are utilized as input for the LS-SVM models. The suggested model underwent testing in multiple scenarios, yielding a maximum accuracy of 97.37%.
Keywords:
Dissolved Gas Analysis, Least-Square Support Vector Machine, Incipient fault, Power transformer, ANN.
References:
[1] Khmais Bacha, Seifeddine Souahlia, and Moncef Gossa, “Power Transformer Fault Diagnosis Based on Dissolved Gas Analysis by Support Vector Machine,” Electric Power Systems Research, vol. 83, no. 1, pp. 73-79, 2012.
[CrossRef] [Google Scholar] [Publisher Link]
[2] Mohamad Aiman Syahmi Rosli et al., “Power Transformer Incipient Fault Diagnosis Using Support Vector Machine,” 2022 3rd International Conference on Artificial Intelligence and Data Sciences (AiDAS), IPOH, Malaysia, pp. 182-185, 2022.
[CrossRef] [Google Scholar] [Publisher Link]
[3] Mohd Syukri Ali et al., “Conventional Methods of Dissolved Gas Analysis Using Oil-Immersed Power Transformer for Fault Diagnosis: A Review,” Electric Power Systems Research, vol. 216, pp. 1-16, 2023.
[CrossRef] [Google Scholar] [Publisher Link]
[4] Rahman Azis Prasojo et al., “A Fuzzy Logic Model for Power Transformer Faults’ Severity Determination Based on Gas Level, Gas Rate, and Dissolved Gas Analysis Interpretation,” Energies, vol. 13, no. 4, pp. 1-20, 2020.
[CrossRef] [Google Scholar] [Publisher Link]
[5] Syahiduddin Misbahulmunir, Vigna K. Ramachandaramurthy, and Yasmin H. MD. Thayoob, “Improved Self-Organizing Map Clustering of Power Transformer Dissolved Gas Analysis Using Inputs Pre-Processing,” IEEE Access, vol. 8, pp. 71798-71811, 2020.
[CrossRef] [Google Scholar] [Publisher Link]
[6] Secil Genc, and Serap Karagol, “Fuzzy Logic Application in DGA Methods to Classify Fault Type in Power Transformer,” 2020 International Congress on Human-Computer Interaction, Optimization and Robotic Applications (HORA), Ankara, Turkey, pp. 1-4, 2020.
[CrossRef] [Google Scholar] [Publisher Link]
[7] Mohammad Ali Akhtar Siddique, and Shabana Mehfuz, “Artificial Neural Networks Based Incipient Fault Diagnosis for Power Transformers,” 2015 Annual IEEE India Conference (INDICON), New Delhi, India, pp. 1-6, 2015.
[CrossRef] [Google Scholar] [Publisher Link]
[8] Nitchamon Poonnoy, Cattareeya Suwanasri, and Thanapong Suwanasri, “Neural Network Approach to Dissolved Gas Analysis for Fault Analysis in Power Transformers,” 2022 International Electrical Engineering Congress (iEECON), Khon Kaen, Thailand, pp. 1-4, 2022.
[CrossRef] [Google Scholar] [Publisher Link]
[9] Kalinda D. Patekar, and Bhoopesh Chaudhry, “DGA Analysis of Transformer Using Artificial Neutral Network to Improve Reliability in Power Transformers,” 2019 IEEE 4th International Conference on Condition Assessment Techniques in Electrical Systems (CATCON), Chennai, India, pp. 1-5, 2019.
[CrossRef] [Google Scholar] [Publisher Link]
[10] D. Saravanan et al., “Fault Prediction of Transformer Using Machine Learning and DGA,” 2020 IEEE International Conference on Computing, Power and Communication Technologies (GUCON), Greater Noida, India, pp. 1-5, 2020.
[CrossRef] [Google Scholar] [Publisher Link]
[11] Hazlee Azil Illias et al., “Fault Identification in Power Transformers Using Dissolve Gas Analysis and Support Vector Machine,” 2021 IEEE International Conference on the Properties and Applications of Dielectric Materials (ICPADM), Johor Bahru, Malaysia, pp. 33-36, 2021.
[CrossRef] [Google Scholar] [Publisher Link]
[12] Fernanda Rabelo Souza, and Bhuvana Ramachandran, “Dissolved Gas Analysis to Identify Faults and Improve Reliability in Transformers Using Support Vector Machines,” 2016 Clemson University Power Systems Conference (PSC), Clemson, USA, pp. 1-4, 2016.
[CrossRef] [Google Scholar] [Publisher Link]
[13] Nutthaphan Boonsaner et al., “The Study and Analysis of Oil-Immersed Power Transformer by Using Artificial Neural Network for Designing Program Apply in the Industry of Testing Oil-immersed Transformers,” 2020 8th International Conference on Condition Monitoring and Diagnosis (CMD), Phuket, Thailand, pp. 274-277, 2020.
[CrossRef] [Google Scholar] [Publisher Link]
[14] Arian Dhini et al., “Intelligent Fault Diagnosis for Power Transformer Based on DGA Data Using Support Vector Machine (SVM),” 2018 3rd International Conference on System Reliability and Safety (ICSRS), Barcelona, Spain, pp. 294-298, 2018.
[CrossRef] [Google Scholar] [Publisher Link]
[15] Teguh Handjojo Dwiputranto, Noor Akhmad Setiawan, and Teguh Bharata Adji, “DGA-Based Early Transformer Fault Detection Using GA-Optimized ANN,” 2021 International Conference on Technology and Policy in Energy and Electric Power (ICT-PEP), Jakarta, Indonesia, pp. 342-347, 2021.
[CrossRef] [Google Scholar] [Publisher Link]
[16] Jagdeep Singh et al., “Condition Assessment of Power Transformer Using SVM Based on DGA,” 2016 Al-Sadeq International Conference on Multidisciplinary in IT and Communication Science and Applications (AIC-MITCSA), AIC-MITCSA 2016, Baghdad, Iraq, pp. 1-5, 2016.
[CrossRef] [Google Scholar] [Publisher Link]
[17] Lov Kumar et al., “Effective Fault Prediction Model Developed Using Least Square Support Vector Machine (LSSVM),” Journal of Systems and Software, vol. 137, pp. 686-712, 2018.
[CrossRef] [Google Scholar] [Publisher Link]