Analyzing and Monitoring of People’s Attention from EEG Signals
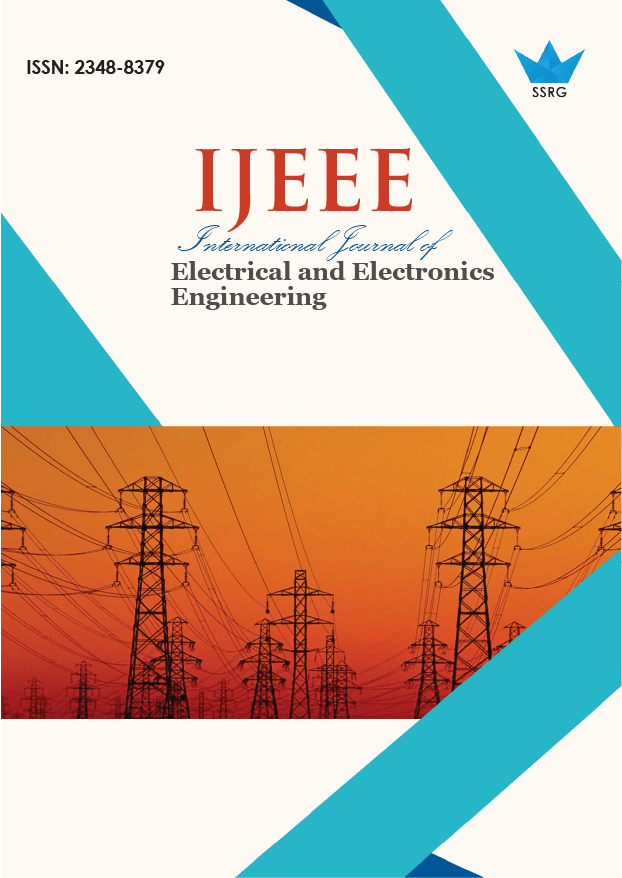
International Journal of Electrical and Electronics Engineering |
© 2024 by SSRG - IJEEE Journal |
Volume 11 Issue 3 |
Year of Publication : 2024 |
Authors : T. Manoj Prasath, R. Vasuki |
How to Cite?
T. Manoj Prasath, R. Vasuki, "Analyzing and Monitoring of People’s Attention from EEG Signals," SSRG International Journal of Electrical and Electronics Engineering, vol. 11, no. 3, pp. 42-64, 2024. Crossref, https://doi.org/10.14445/23488379/IJEEE-V11I3P104
Abstract:
The human brain is made up of millions of neurons which are responsible for the regulation of motor and sensory events. Analyzing brain signals or images can help one understand the cognitive behaviour of the brain. On earth, psychologists often think about reading EEG signals in order to get a typical analysis of an individual. Yet, it can be pretty challenging and varies from person to person to comprehend how an individual behaves and responds to the numerous orders set up for them. This research is prone to an experimental take away of the personage’s electroencephalographic waveform, retrieving the unusually fluctuating curves over suspected guidelines during the complete examination. EEG fluctuations play a significant role in this procedure because it is possible to distinguish crucial details specifically from the waveform. With the assistance of a connected set of probes, an impotent lead, an EEG kit, and the patient being evaluated, this intervention is carried out automatically. This work analyses the attention from EEG signals obtained. A proprietary eSense algorithm was designed to calculate attention. To verify the proposed algorithm, an application was created, which was used in the experiment itself. Next, an analysis of the results and progress of the different phases was carried out based on the data obtained from the measurements. Finally, the overall success of the proposed algorithm was evaluated, and the strengths and weaknesses of the chosen approach were discussed. The calculated values were compared with those from the headband to check whether the “eSense” algorithm matches methods based on traditional techniques.
Keywords:
Brain states, Electroencephalogram (EEG), Fluctuating curves, Nervous system, eSense algorithm.
References:
[1] Yongjae Park et al., “A Real-Time Depth of Anesthesia Monitoring System Based on Deep Neural Network with Large EDO Tolerant EEG Analog Front-End,” IEEE Transactions on Biomedical Circuits and Systems, vol. 14, no. 4, pp. 825-837, 2020.
[CrossRef] [Google Scholar] [Publisher Link]
[2] Yongcheng Li et al., “Electromyogram (EMG) Removal by Adding Sources of EMG (ERASE)-A Novel ICA-Based Algorithm for Removing Myoelectric Artifacts from EEG,” Frontiers in Neuroscience, vol. 14, pp. 1-16, 2021.
[CrossRef] [Google Scholar] [Publisher Link]
[3] Jiahui Xu, and Baichang Zhong, “Review on Portable EEG Technology in Educational Research,” Computers in Human Behavior, vol. 81, pp. 340-349, 2018.
[CrossRef] [Google Scholar] [Publisher Link]
[4] Chi-Yuan Chang et al., “Evaluation of Artifact Subspace Reconstruction for Automatic Artifact Components Removal in Multi-Channel EEG Recordings,” IEEE Transactions on Biomedical Engineering, vol. 67, no. 4, pp. 1114-1121, 2020.
[CrossRef] [Google Scholar] [Publisher Link]
[5] Rumen Pozharliev et al., “Merely being with you Increases my Attention to Luxury Products: Using EEG to Understand Consumers’ Emotional Experience with Luxury Branded Products,” Journal of Marketing Research, vol. 52, no. 4, pp. 546-558, 2019.
[CrossRef] [Google Scholar] [Publisher Link]
[6] Marie-Pierre Deiber et al., “Linking Alpha Oscillations, Attention and Inhibitory Control in Adult ADHD with EEG Neurofeedback,” NeuroImage: Clinical, vol. 25, 2019.
[CrossRef] [Google Scholar] [Publisher Link]
[7] Zehong Cao et al., “Multi-Channel EEG Recordings during a Sustained-Attention Driving Task,” Scientific Data, vol. 6, pp. 1-8, 2019.
[CrossRef] [Google Scholar] [Publisher Link]
[8] F. Lotte et al., “A Review of Classification Algorithms for EEG-Based Brain-Computer Interfaces: A 10 Year Update,” Journal of Neural Engineering, vol. 15, pp. 1-28, 2018.
[CrossRef] [Google Scholar] [Publisher Link]
[9] Alexander Craik, Yongtian He, and Jose L. Contreras-Vidal, “Deep Learning for Electroencephalogram (EEG) Classification Tasks: A Review,” Journal of Neural Engineering, vol. 16, pp. 1-28, 2019.
[CrossRef] [Google Scholar] [Publisher Link]
[10] Mohammad-Parsa Hosseini, Amin Hosseini, and Kiarash Ahi, “A Review on Machine Learning for EEG Signal Processing in Bioengineering,” IEEE Reviews in Biomedical Engineering, vol. 14, pp. 204-218, 2020.
[CrossRef] [Google Scholar] [Publisher Link]
[11] Michael Murphy et al., “Propofol Anesthesia and Sleep: A High-Density EEG Study,” Sleep, vol. 34, no. 3, pp. 283-291, 2011.
[CrossRef] [Google Scholar] [Publisher Link]
[12] Yvonne Blokland et al., “Decoding Motor Responses from the EEG during Altered States of Consciousness Induced by Propofol,” Journal of Neural Engineering, vol. 13, pp. 1-9, 2016.
[CrossRef] [Google Scholar] [Publisher Link]
[13] Sébastien Rimbert et al., “MOTANA: Study Protocol to Investigate Motor Cerebral Activity during A Propofol Sedation,” Trials, vol. 20, pp. 1-9, 2019.
[CrossRef] [Google Scholar] [Publisher Link]
[14] Ahmad Chaddad et al., “Electroencephalography Signal Processing: A Comprehensive Review and Analysis of Methods and Techniques,” Sensors, vol. 23, no. 14, pp. 1-27, 2023.
[CrossRef] [Google Scholar] [Publisher Link]
[15] Dias Issa, M. Fatih Demirci, and Adnan Yazici, “Speech Emotion Recognition with Deep Convolutional Neural Networks,” Biomedical Signal Processing and Control, vol. 59, 2020.
[CrossRef] [Google Scholar] [Publisher Link]
[16] Steven R. Livingstone, and Frank A. Russo et al., “The Ryerson Audio-Visual Database of Emotional Speech and Song (RAVDESS): A Dynamic, Multimodal Set of Facial and Vocal Expressions in North American English,” PLOS ONE, vol. 13, no. 5, 2018.
[CrossRef] [Google Scholar] [Publisher Link]
[17] Abdul Malik Badshah et al., “Speech Emotion Recognition from Spectrograms with Deep Convolutional Neural Network,” 2017 International Conference on Platform Technology and Service (PlatCon), Busan, Korea, pp. 1-5, 2017.
[CrossRef] [Google Scholar] [Publisher Link]
[18] Paul L. Nunez, and Ramesh Srinivasan, Electric Fields of the Brain: The Neurophysics of EEG, 2nd ed., New York: Oxford University Press, 2006.
[CrossRef] [Google Scholar] [Publisher Link]
[19] Yuneisy Garcia Guzman, and Michael Lunglmayr, “Sparse Cyclic Coordinate Descent for Efficient Frequency Estimation,” 2019 IEEE 8 th International Workshop on Computational Advances in Multi-Sensor Adaptive Processing (CAMSAP), Le gosier, Guadeloupe, pp. 440-444, 2019.
[CrossRef] [Google Scholar] [Publisher Link]
[20] Gui-Lin Chen, Shang-Ho Tsai, and Kai-Jiun Yang, “On Performance of Sparse Fast Fourier Transform and Enhancement Algorithm,” IEEE Transactions on Signal Processing, vol. 65, no. 21, pp. 5716-5729, 2017.
[CrossRef] [Google Scholar] [Publisher Link]
[21] Bin Li, Zhikang Jiang, and Jie Chen, “On Performance of Sparse Fast Fourier Transform Algorithms Using the Aliasing Filter,” Electronics, vol. 10, no. 9, pp. 1-27, 2021.
[CrossRef] [Google Scholar] [Publisher Link]
[22] Hau-Tieng Wu, “Current State of Nonlinear-Type Time-Frequency Analysis and Applications to High-Frequency Biomedical Signals,” Current Opinion in Structural Biology, vol. 23, pp. 8-21, 2020.
[CrossRef] [Google Scholar] [Publisher Link]
[23] Harshit Bhardwaj et al., “EEG-Based Personality Prediction Using Fast Fourier Transform and DeepLSTM Model,” Computational Intelligence and Neuroscience, vol. 2021, pp. 1-10, 2021.
[CrossRef] [Google Scholar] [Publisher Link]
[24] Dhiran Kumar Mahto, and A.K. Singh, “A Survey of Color Image Watermarking: State-of-the-Art and Research Directions,” Computers & Electrical Engineering, vol. 93, 2021.
[CrossRef] [Google Scholar] [Publisher Link]
[25] Lauer C., (Prod.), Hangar 1 - The UFO Files (Own Recording), Prima ZOOM, A&E Television Networks, 2014.
[26] G.E. Berrios, “Musical Hallucinations - A Historical and Clinical Study,” The British Journal of Psychiatry, vol. 156, no. 2, pp. 188-194, 1990.
[CrossRef] [Google Scholar] [Publisher Link]
[27] Noam Brown, and Tuomas Sandholm, “Superhuman AI for Heads-Up No-Limit Poker: Libratus Beats Top Professionals,” Science, vol. 359, no. 6374, pp. 418-424, 2017.
[CrossRef] [Google Scholar] [Publisher Link]
[28] Moyuru Kurita, and Kunihito Hoki, “Method for Constructing Artificial Intelligence Player with Abstractions to Markov Decision Processes in Multiplayer Game of Mahjong,” IEEE Transactions on Games, vol. 13, no. 1, pp. 99-110, 2021.
[CrossRef] [Google Scholar] [Publisher Link]
[29] Genaro Rebolledo-Mendez et al., “Assessing NeuroSky’s Usability to Detect Attention Levels in an Assessment Exercise,” HumanComputer Interaction. New Trends, pp. 149-158, 2009.
[CrossRef] [Google Scholar] [Publisher Link]
[30] Thomas H. Budzynski et al., Introduction to Quantitative EEG and Neurofeedback: Advanced Theory and Applications, 2nd ed., Academic Press, 2009.
[CrossRef] [Google Scholar] [Publisher Link]
[31] Mengxi Dai et al., “EEG Classification of Motor Imagery Using a Novel Deep Learning Framework,” Sensors, vol. 19, no. 3, pp. 1-16, 2019.
[CrossRef] [Google Scholar] [Publisher Link]
[32] Md. Kafiul Islam, and Amir Rastegarnia, “Recent Advances in EEG (Non-Invasive) Based BCI Applications,” Frontiers in Computational Neuroscience, vol. 17, pp. 1-3, 2023.
[CrossRef] [Google Scholar] [Publisher Link]
[33] Szymon Fiałek, and Fotis Liarokapis, “Comparing Two Commercial Brain Computer Interfaces for Serious Games and Virtual Environments,” Emotion in Games, pp. 103-117, 2016.
[CrossRef] [Google Scholar] [Publisher Link]