Intelligent Crime Scene Recognition: Advancing Public Safety through Deep Learning Architectures and Event Sequence Analysis
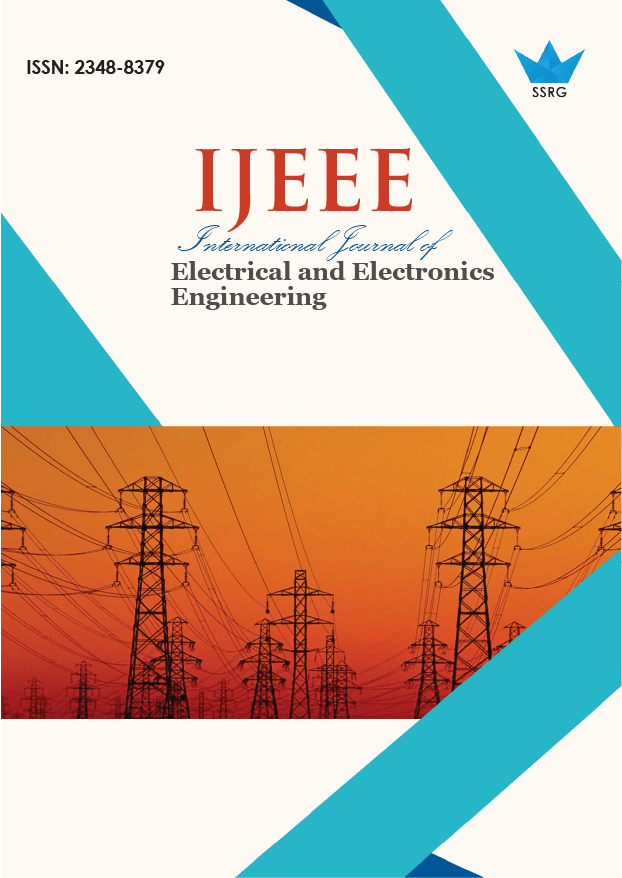
International Journal of Electrical and Electronics Engineering |
© 2024 by SSRG - IJEEE Journal |
Volume 11 Issue 4 |
Year of Publication : 2024 |
Authors : Anil Hingmire, Aman Sheikh, Saurabh Shukla, Rahul Shah, Sunayana Jadhav, Tatwadarshi P. Nagarhalli, Amrita Ruperee |
How to Cite?
Anil Hingmire, Aman Sheikh, Saurabh Shukla, Rahul Shah, Sunayana Jadhav, Tatwadarshi P. Nagarhalli, Amrita Ruperee, "Intelligent Crime Scene Recognition: Advancing Public Safety through Deep Learning Architectures and Event Sequence Analysis," SSRG International Journal of Electrical and Electronics Engineering, vol. 11, no. 4, pp. 64-71, 2024. Crossref, https://doi.org/10.14445/23488379/IJEEE-V11I4P108
Abstract:
In contemporary society, ensuring public safety is a paramount concern, and one of the significant challenges faced by law enforcement agencies is the swift detection and classification of criminal activities from surveillance footage. Current crime scene detection systems often lack real-time analysis and struggle with the prompt identification of criminal acts, hindering the timely response required for effective law enforcement. Consequently, there is a critical need for an advanced Crime Scene Detection System (CSDS) capable of classifying the type of crime occurring in real time, triggering immediate alarms, and aiding in the rapid identification of criminals captured within the surveillance footage. In this paper, we have highlighted various types’ of techniques that can be used to detect crime scenes using Artificial Intelligence (AI). Crime detection using Convolutional Neural Networks (CNNs) and Long Short-Term Memory (LSTM) involves the application of deep learning techniques to analyse and interpret complex patterns in crime-related data. The primary objective is to accurately identify and classify criminal activities, thereby assisting law enforcement agencies in taking proactive measures to ensure public safety. This multidimensional approach is essential for addressing the dynamic nature of criminal behavior, the diversity of criminal activities, and the need for real-time data processing. The analysis reveals that the LRCN model excels in accurately identifying crime events, achieving an impressive 94% accuracy. In contrast, the CNN-LRCN model lags behind with an 84% accuracy rate.
Keywords:
Artificial Intelligence, Crime Scene Detection System, Machine Learning, Deep Learning, Neural Network, Convolutional Neural Network (CNN), Long Short-Term Memory (LSTM), Chain snatching.
References:
[1] Varun Mandalapu et al., “Crime Prediction Using Machine Learning and Deep Learning: A Systematic Review and Future Directions,” IEEE Access, vol. 11, pp. 60153-60170, 2023.
[CrossRef] [Google Scholar] [Publisher Link]
[2] Karabo Jenga, Cagatay Catal, and Gorkem Kar, “Machine Learning in Crime Prediction,” Journal of Ambient Intelligence and Humanized Computing, vol. 14, pp. 2887–2913, 2023.
[CrossRef] [Google Scholar] [Publisher Link]
[3] Ashokkumar Palanivinayagam et al., “An Optimized Machine Learning and Big Data Approach to Crime Detection,” Wireless Communications and Mobile Computing, vol. 2021, pp. 1-10, 2021.
[CrossRef] [Google Scholar] [Publisher Link]
[4] Amril Nazir et al., “Suspicious Behavior Detection with Temporal Feature Extraction and Time-Series Classification for Shoplifting Crime Prevention,” Sensors, vol. 23, no. 13, pp. 1-19, 2023.
[CrossRef] [Google Scholar] [Publisher Link]
[5] Sakib Mahmud, Musfika Nuha, and Abdus Sattar, “Crime Rate Prediction Using Machine Learning and Data Mining,” Soft Computing Techniques and Applications, vol. 1248, 2020.
[CrossRef] [Google Scholar] [Publisher Link]
[6] Mohammad Reza Keyvanpour, Mostafa Javideh, and Mohammad Reza Ebrahimi, “Detecting and Investigating Crime by Means of Data Mining: A General Crime Matching Framework,” Procedia Computer Science, vol. 3, pp. 872-880, 2011.
[CrossRef] [Google Scholar] [Publisher Link]
[7] Neil Shah, Nandish Bhagat, and Manan Shah, “Crime Forecasting: A Machine Learning and Computer Vision Approach to Crime Prediction and Prevention,” Visual Computing for Industry, Biomedicine, and Art, vol. 4, pp. 1-14, 2021.
[CrossRef] [Google Scholar] [Publisher Link]
[8] Mark Petty, Shyh Wei Teng, and Manzur Murshed, “Improved Image Analysis Methodology for Detecting Changes in Evidence Positioning at Crime Scenes,” 2019 Digital Image Computing: Techniques and Applications (DICTA), Perth, Australia, pp. 1-8, 2019.
[CrossRef] [Google Scholar] [Publisher Link]
[9] Mohammed Boukabous, and Mostafa Azizi, “Image and Video-Based Crime Prediction Using Object Detection and Deep Learning,” Bulletin of Electrical Engineering and Informatics, vol. 12, no. 3, pp. 1630-1638, 2023.
[CrossRef] [Google Scholar] [Publisher Link]
[10] G. Sreenu, and M.A. Saleem Durai, “Intelligent Video Surveillance: A Review through Deep Learning Techniques for Crowd Analysis,” Journal of Big Data, vol. 6, pp. 1-27, 2019.
[CrossRef] [Google Scholar] [Publisher Link]
[11] Yu-Gang Jiang et al., “High-Level Event Recognition in Unconstrained Videos,” International Journal of Multimedia Information Retrieval, vol. 2, pp. 73-101, 2013.
[CrossRef] [Google Scholar] [Publisher Link]
[12] Christos Tzelepis et al., “Learning to Detect Video Events from Zero or Very Few Video Examples,” Image and Vision Computing, vol. 53, pp. 35-44, 2016.
[CrossRef] [Google Scholar] [Publisher Link]
[13] Roberto Arroyo et al., “Expert Video-Surveillance System for Real-Time Detection of Suspicious Behaviors in Shopping Malls,” Expert Systems with Applications, vol. 42, no. 21, pp. 7991-8005, 2015.
[CrossRef] [Google Scholar] [Publisher Link]
[14] Anima Pramanik, Sobhan Sarkar, and J. Maiti, “A Real-Time Video Surveillance System for Traffic Pre-Events Detection,” Accident Analysis & Prevention, vol. 154, 2021.
[CrossRef] [Google Scholar] [Publisher Link]
[15] Jianguo Chen et al., “Distributed Deep Learning Model for Intelligent Video Surveillance Systems with Edge Computing,” IEEE Transactions on Industrial Informatics, 2019.
[CrossRef] [Google Scholar] [Publisher Link]
[16] Shiju Sathyadevan et al., “Identifying Moving Bodies from CCTV Videos Using Machine Learning Techniques,” 2014 First International Conference on Networks & Soft Computing (ICNSC2014), India, pp. 151-157, 2014.
[CrossRef] [Google Scholar] [Publisher Link]
[17] Year-wise Details of the Number of Cases Reported against Women: Harassment, Rape, Chain Snatching, Theft, and Robbery from 2018 to 2022, A Digital India Initiative, 2023. [Online]. Available: https://data.gov.in/resource/year-wise-details-number-cases-reported-against-women-harassment-rape-chain-snatching
[18] State/UT-wise Details of Cases Registered under Crime against Women in the Country from 2019 to 2021, A Digital India Initiative, 2023. [Online]. Available: https://data.gov.in/resource/stateut-wise-details-cases-registered-under-crime-against-women-country-2019- 2021