Machine Learning-Based Structure Prediction for QM9 Quantum Datasets
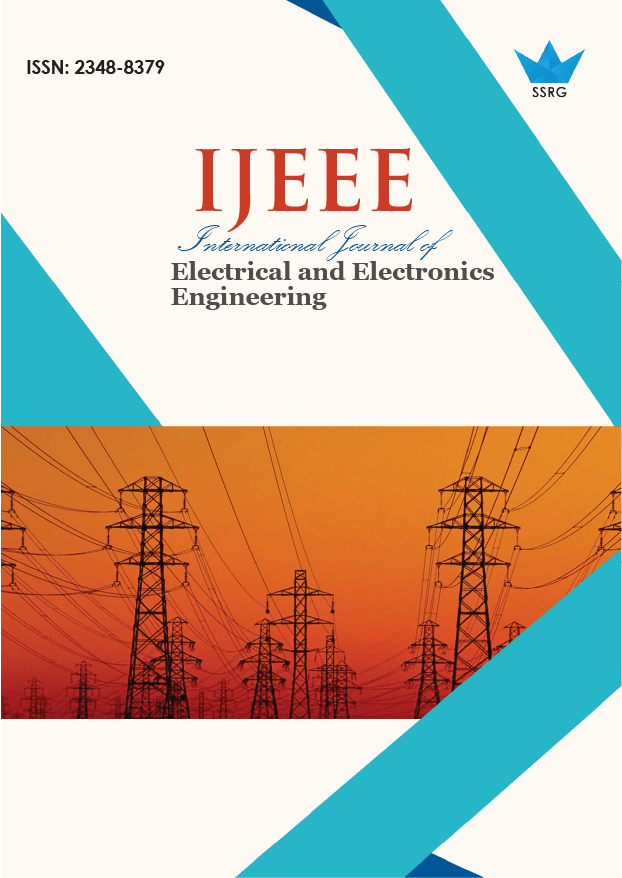
International Journal of Electrical and Electronics Engineering |
© 2024 by SSRG - IJEEE Journal |
Volume 11 Issue 4 |
Year of Publication : 2024 |
Authors : Nahla K, Maimoona Ansari, Salah Eldeen F. Hegazi, Anjali Appukuttan, Bincy Vincent, Huda Fatima |
How to Cite?
Nahla K, Maimoona Ansari, Salah Eldeen F. Hegazi, Anjali Appukuttan, Bincy Vincent, Huda Fatima, "Machine Learning-Based Structure Prediction for QM9 Quantum Datasets," SSRG International Journal of Electrical and Electronics Engineering, vol. 11, no. 4, pp. 226-233, 2024. Crossref, https://doi.org/10.14445/23488379/IJEEE-V11I4P124
Abstract:
The inner arrangement of the quantum mechanics dataset QM9 is investigated in this study. The dataset contains 1000 organic molecules as well as being defined in terms of electronic properties. To estimate the atomic composition using inverse molecular design attributes, one must understand the structure and properties of such data. The study used methods for detecting outliers, clustering, and intrinsic dimension analysis. The dataset was found to have descriptive dimensions far higher than their intrinsic dimensionality. Inliner items make up the majority of the inner core area of the QM9 data, whereas outliers dominate the outside region. The atom count in a molecule is strongly related to its outlier or inner character. Despite structural differences, important variables for inverse molecular design are very predictable. The molecular representation was estimated using Graph Neural Network (GNN), a modern Machine Learning (ML) algorithm. This study also did feature extraction and preprocessing before this algorithm. This proposed technique works for the outcomes.
Keywords:
Feature generation, Feature selection, Machine Learning, Outlier analysis, QM9 data.
References:
[1] Raghunathan, Shampa, and U. Deva Priyakumar, “Molecular Representations for Machine Learning Applications in Chemistry,” International Journal of Quantum Chemistry, vol. 122, no. 7, pp. 1-21, 2022.
[CrossRef] [Google Scholar] [Publisher Link]
[2] Clemens Isert et al., “QMugs, Quantum Mechanical Properties of Drug-Like Molecules,” Scientific Data, vol. 9, pp. 1-11, 2022.
[CrossRef] [Google Scholar] [Publisher Link]
[3] Mohammadamin Tavakoli et al., “Quantum Mechanics and Machine Learning Synergies: Graph Attention Neural Networks to Predict Chemical Reactivity,” Journal of Chemical Information and Modeling, vol. 62, no. 9, pp. 2121-2132, 2022.
[CrossRef] [Google Scholar] [Publisher Link]
[4] Surajit Nandi, Tejs Vegge, and Arghya Bhowmik, “MultiXC-QM9: Large Dataset of Molecular and Reaction Energies from Multi-Level Quantum Chemical Methods,” Scientific Data, vol. 10, pp. 1-6, 2023.
[CrossRef] [Google Scholar] [Publisher Link]
[5] Surajit Nandi, Tejs Vegge, and Arghya Bhowmik, “Large Dataset of Molecular and Reaction Energies from Multi-Level Quantum Chemical Methods,” ChemRxiv, pp. 1-7, 2022.
[CrossRef] [Google Scholar] [Publisher Link]
[6] Tien-Sinh Vu et al., “Towards Understanding Structure-Property Relations in Materials with Interpretable Deep Learning,” NPJ Computational Materials, vol. 9, pp. 1-12, 2023.
[CrossRef] [Google Scholar] [Publisher Link]
[7] Luis Itza Vazquez-Salazar et al., “Impact of the Characteristics of Quantum Chemical Databases on Machine Learning Prediction of Tautomerization Energies,” Journal of Chemical Theory and Computation, vol. 17, no. 8, pp.4769-4785, 2021.
[CrossRef] [Google Scholar] [Publisher Link]
[8] Maria Avramouli et al., “Quantum Machine Learning in Drug Discovery: Current State and Challenges,” Proceedings of the 26th Pan-Hellenic Conference on Informatics, pp. 394-401, 2022.
[CrossRef] [Google Scholar] [Publisher Link]
[9] Ge Yan, Huaijin Wu, and Junchi Yan, “Quantum 3D Graph Learning with Applications to Molecule Embedding,” Proceedings of the 40th International Conference on Machine Learning, pp. 39126-39137, 2023.
[Google Scholar] [Publisher Link]
[10] Hajime Shimakawa, Akiko Kumada, and Masahiro Sato, “Extrapolative Prediction of Small-Data Molecular Property Using Quantum Mechanics-Assisted Machine Learning,” NPJ Computational Materials, vol. 10, pp. 1-14, 2024.
[CrossRef] [Google Scholar] [Publisher Link]
[11] Julio J. Valdés, and Alain B. Tchagang, “Understanding the Structure of qm7b and qm9 Quantum Mechanical Datasets Using Unsupervised Learning,” arXiv, pp. 1-8, 2023.
[CrossRef] [Google Scholar] [Publisher Link]
[12] Christopher Gaul, and Santiago Cuesta-Lopez, “Machine Learning for Orbital Energies of Organic Molecules Upwards of 100 Atoms,” Physica Status Solidi, vol. 261, no. 1, pp. 1-11, 2024.
[CrossRef] [Google Scholar] [Publisher Link]
[13] Anton V. Sinitskiy, and Vijay S. Pande, “Physical Machine Learning Outperforms “Human Learning” in Quantum Chemistry,” arXiv, pp. 1-62, 2019.
[CrossRef] [Google Scholar] [Publisher Link]
[14] S. Heinen, G.F. von Rudorff, and O.A. von Lilienfeld, “Geometry Relaxation and Transition State Search throughout Chemical Compound Space with Quantum Machine Learning,” arXiv, pp. 1-7, 2022.
[CrossRef] [Google Scholar] [Publisher Link]
[15] Johannes Hoja et al., “QM7-X, A Comprehensive Dataset of Quantum-Mechanical Properties Spanning the Chemical Space of Small Organic Molecules,” Scientific Data, vol. 8, pp. 1-11, 2021.
[CrossRef] [Google Scholar] [Publisher Link]
[16] Dominik Lemm, Guido Falk von Rudorff, and O. Anatole von Lilienfeld, “Machine Learning Based Energy-Free Structure Predictions of Molecules, Transition States, and Solids,” Nature Communications, vol. 12, pp. 1-10, 2021.
[CrossRef] [Google Scholar] [Publisher Link]
[17] Guangyong Chen et al., “Alchemy: A Quantum Chemistry Dataset for Benchmarking AI Models,” arXiv, pp. 1-11, 2019.
[CrossRef] [Google Scholar] [Publisher Link]
[18] Julio J. Valdés, and Alain B. Tchagang, “Novel Machine Learning Insights into the QM7b and QM9 Quantum Mechanics Datasets,” Journal of Computational Chemistry, vol. 45, no. 15, pp. 1193-1214, 2024.
[CrossRef] [Google Scholar] [Publisher Link]