Insight on the Application of Deep Learning-Based Thermal Image Processing Methods in Electrical System Anomaly Detection and their Comparative Analysis
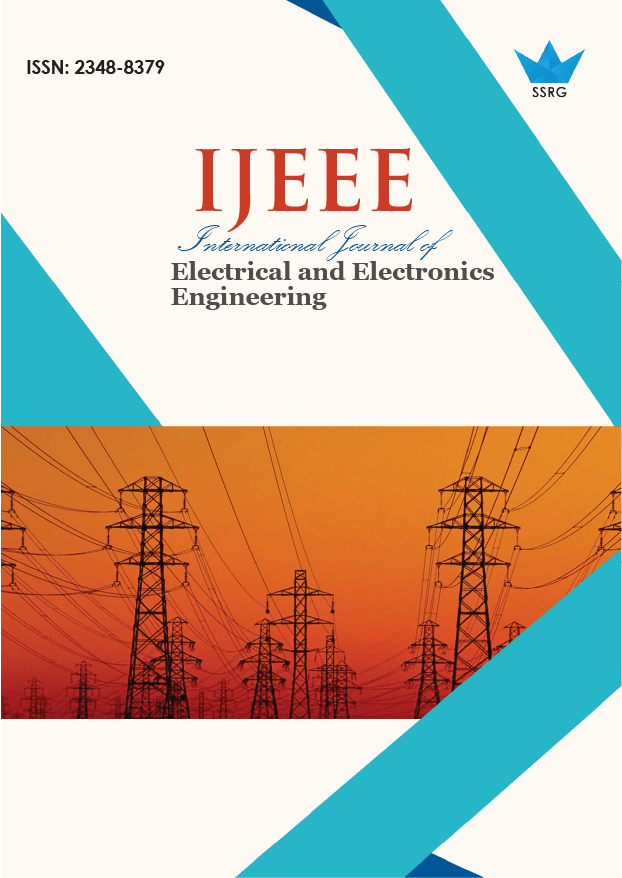
International Journal of Electrical and Electronics Engineering |
© 2024 by SSRG - IJEEE Journal |
Volume 11 Issue 4 |
Year of Publication : 2024 |
Authors : Pankaj Chaudhari, Sajid Patel, Mohammedirfan I. Siddiqui, Nupur Sinha |
How to Cite?
Pankaj Chaudhari, Sajid Patel, Mohammedirfan I. Siddiqui, Nupur Sinha, "Insight on the Application of Deep Learning-Based Thermal Image Processing Methods in Electrical System Anomaly Detection and their Comparative Analysis," SSRG International Journal of Electrical and Electronics Engineering, vol. 11, no. 4, pp. 240-253, 2024. Crossref, https://doi.org/10.14445/23488379/IJEEE-V11I4P126
Abstract:
Ensuring the reliability and safety of electrical systems necessitates constant inspections. However, manual inspections pose risks, are time-consuming, and are impractical for real-time monitoring. This paper presents a novel, noninvasive, and efficient approach for automated electrical system anomaly detection using deep learning and thermal image processing. We have proposed a Convolutional Neural Network (CNN) based framework utilizing the well-established GoogLeNet and other deep learning-based architecture to classify thermal images of electrical systems as “normal” or “abnormal.” This framework achieves a high accuracy of 99% in anomaly detection, surpassing traditional methods and paving the way for real-time monitoring and early fault identification.
Keywords:
Anomaly detection, CNN, Deep Learning, Electrical system inspection, Predictive maintenance, Thermal imaging.
References:
[1] Ahmad Bala Alhassan et al., “Power Transmission Line Inspection Robots: A Review, Trends and Challenges for Future Research,” International Journal of Electrical Power & Energy Systems, vol. 118, 2020.
[CrossRef] [Google Scholar] [Publisher Link]
[2] Zhaoyang Wang et al., “A Review of UAV Power Line Inspection,” Advances in Guidance, Navigation and Control, pp. 3147-3159, 2021.
[CrossRef] [Google Scholar] [Publisher Link]
[3] Amine Mahami et al., “Automated Transformer Fault Diagnosis Using Infrared Thermography Imaging, GIST and Machine Learning-Technique,” Proceedings of the Institution of Mechanical Engineers, Part E: Journal of Process Mechanical Engineering, vol. 236, no. 4, pp. 1747-1757, 2022.
[CrossRef] [Google Scholar] [Publisher Link]
[4] Zhi Qu, Peng Jiang, and Weixu Zhang, “Development and Application of Infrared Thermography Non-Destructive Testing Techniques,” Sensors, vol. 20, no. 14, pp. 1-26, 2020.
[CrossRef] [Google Scholar] [Publisher Link]
[5] Cheng Zhang, Haidi Yi, and Yuan Li, “A Novel Incipient Fault Detection and Diagnosis Scheme Based on Kernel Density Weighting Support Vector Data Description: Application on the DAMADICS Benchmark Process,” Journal of Chemical Engineering of Japan, vol. 56, no. 1, 2023.
[CrossRef] [Google Scholar] [Publisher Link]
[6] Ju Sik Kim, Kyu Nam Choi, and Sung Woo Kang, “Infrared Thermal Image-Based Sustainable Fault Detection for Electrical Facilities,” Sustainability, vol. 13, no. 2, pp. 1-15, 2021.
[CrossRef] [Google Scholar] [Publisher Link]
[7] Ezechukwu Kalu Ukiwe, Steve A. Adeshina, and Jacob Tsado, “Techniques of Infrared Thermography for Condition Monitoring of Electrical Power Equipment,” Journal of Electrical Systems and Information Technology, vol. 10, pp. 1-19, 2023.
[CrossRef] [Google Scholar] [Publisher Link]
[8] Shanmugam Chellamuthu, and E. Chandira Sekaran, “Fault Detection in Electrical Equipment’s Images by Using Optimal Features with Deep Learning Classifier,” Multimedia Tools and Applications, vol. 78, pp. 27333-27350, 2019.
[CrossRef] [Google Scholar] [Publisher Link]
[9] Hejin Yuan et al., “State Detection of Electrical Equipment Based on Infrared Thermal Imaging Technology,” Pattern Recognition and Computer Vision, pp. 251-260, 2019.
[CrossRef] [Google Scholar] [Publisher Link]